The following insights are derived from a recent session on GenAI in Healthcare.
On November 15th, the D^3 Institute’s Generative AI Observatory hosted the first session in its GenAI in Healthcare series, produced in collaboration with the Responsible AI Institute. Speakers Satish Tadikonda (HBS), Nikhil Bhojwani (Recon Strategy), Var Shankar (Responsible AI Institute), and Alyssa Lefaivre Skopac (Responsible AI Institute) discussed key insights on operationalizing GenAI while minimizing risks, as well as the different guardrails to consider across various healthcare sectors. Below are some of the session’s key takeaways, which will be explored further in subsequent sessions.
Many Stages of AI Adoption in Healthcare
The healthcare industry already contains many AI use cases, and GenAI represents the next stage of potential adoption. There are multiple examples where such tools are already being used to measure and evaluate, aid in decision-making, or execute treatment plans. However, organizations are currently at different levels of maturity in how they approach AI implementation, with many unsure of how best to approach this type of change. A sample poll of our audience showed that 37% were just starting out on their AI journey, and 30% felt they were headed in the right direction but not in the realm of industry leaders. With the exception of 4% who felt they were in that frontrunner category, the rest expressed uncertainty with how their organization would undertake AI adoption.
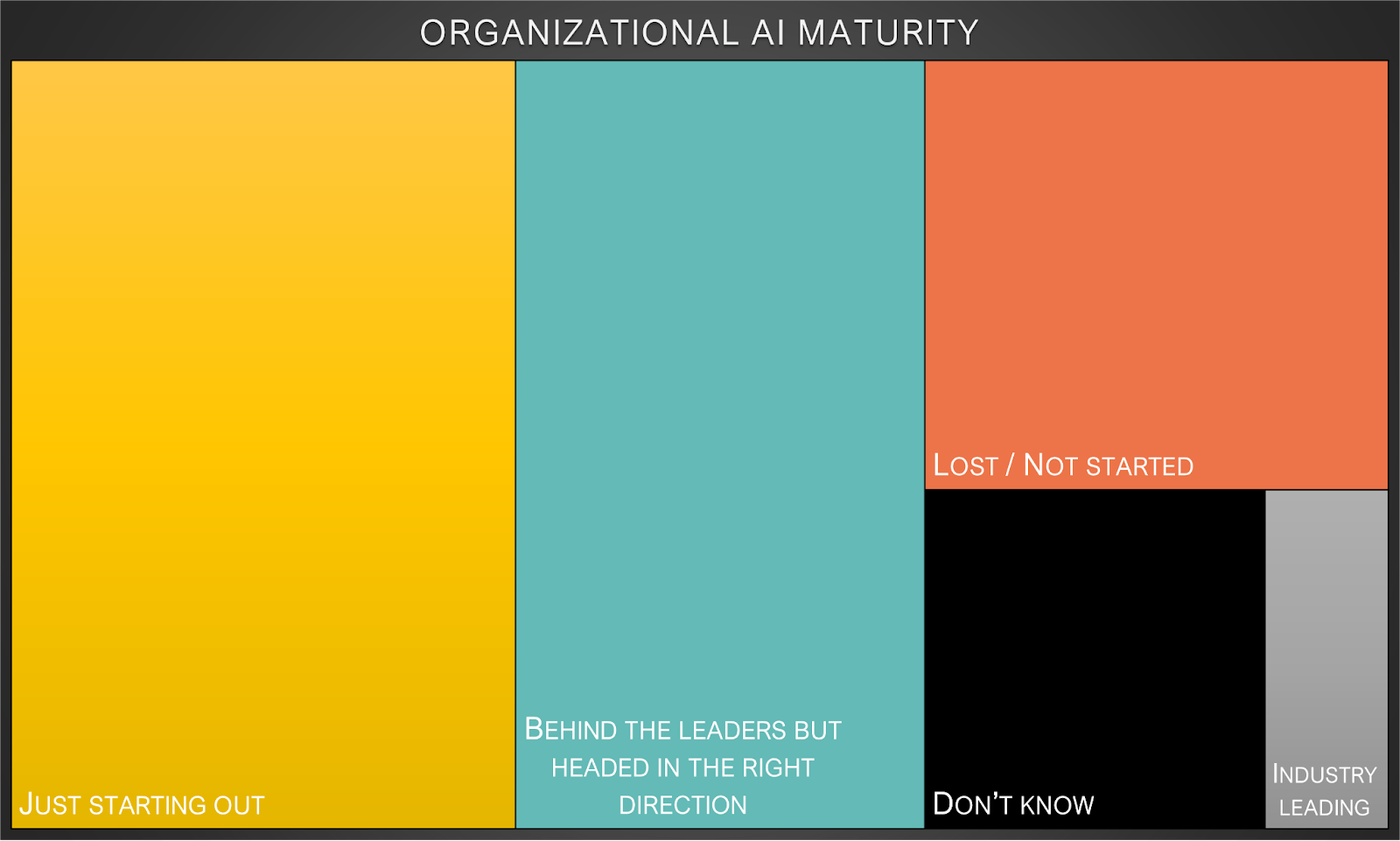
Broad, Scaleable Impact – If Approached Correctly
The world is rapidly coming to terms with how impactful AI will be, particularly with growing advancements and acceptance of new tools like those powered by GenAI. In order to ensure those impacts are positive, however, there are underlying concerns which must be addressed before such methods can be achieved at scale. This is especially true in the healthcare industry, where relying on the right data and decision-making process is extremely vital. As with the risks listed below, failing to responsibly address AI concerns can lead to dire outcomes, and should be approached with a great deal of care.
Responsible AI Commonalities and Considerations
The concerns represented in the left column above can be applied to a wide array of industries and use cases, as represented by examples in pharmaceutical and AI-powered skin disease detection during the first GenAI in Healthcare session. For that reason, organizations like the Responsible AI Institute and their partners are working to develop actionable frameworks, tools, and best practices which can offer guidance on how best to embark on the AI implementation journey.
While there are common elements of responsible AI, there are also specific considerations for each healthcare sector. In the next GenAI in Healthcare sessions, we’ll dive deeper into sectors such as Biotech, Pharma, Digital Health, and more, sharing specific case studies and insights from industry leaders about how to utilize powerful AI innovations responsibly in healthcare.
Join us on December 13 for a deep dive on scaling AI applications in the Biopharma sector: