In today’s fast-evolving business landscape, the ability to innovate rapidly has become a defining factor for success. Companies like Netflix, Amazon, and Microsoft have leveraged robust experimentation frameworks to enhance decision-making, optimize products, and continue innovating. Iavor Bojinov, Assistant Professor at Harvard Business School and Principal Investigator at the Digital Data Design Institute (D^3) Data Science and AI Operations Lab hosted within the Laboratory for Innovation Science; David Holtz, Assistant Professor at the Haas School of Business, University of California, Berkeley; Ramesh Johari, Professor of Management Science and Engineering at Stanford University; Sven Schmit, Head of Statistics Engineering at Eppo; and Martin Tingley, Head of the Experimentation Platform Analysis Team at Netflix, recently published an analysis of this topic, “Want Your Company to Get Better at Experimentation? Learn Fast by Democratizing Testing.” The article provides actionable insights on how firms can democratize and scale experimentation enterprise-wide.
Key Insight: The Need for Speed and Scale
“[T]eams and companies that run lots of tests outperform those that conduct just a few.” [1]
The authors cite research at Microsoft and elsewhere that shows that the sheer volume of experimentation often correlates with success. Most ideas fail to produce meaningful outcomes, so running more experiments increases the chances of discovering impactful changes. The advent of generative AI further accelerates this process by making it cheaper and faster to create and test digital product experiences.
Key Insight: Democratizing Experimentation
“Scaling up experimentation entails moving away from a data-scientist-centric approach to one that empowers everyone on product, marketing, engineering, and operations teams.” [2]
The research emphasizes the limitations of relying solely on data scientists for experimentation. While this centralized model ensures statistical rigor, it restricts scalability. By transitioning to a self-service model, companies can empower a broader range of employees to test ideas and take action based on the results. Testing tools with user-friendly interfaces, automatically imposed statistical rigor, embedded experimentation protocols, automated rollbacks, and AI-powered assistants are key to democratizing experimentation. In this context, data scientists set up the testing platform, train employees to use it, and provide ongoing support; however, they can shift their focus to new and high-impact tests that require specialized expertise. The authors also emphasize the need to adjust incentives for employees to experiment, by evaluating them on overall department and company performance rather than the success of individual tests.
Key Insight: Hypothesis-Driven Innovation
“The experiment allows them to test the theory; by considering additional metrics, they can understand the mechanism that drove the result.” [3]
Hypothesis-driven experimentation extends beyond simply choosing between alternatives—it seeks to uncover the “why” behind results and provide initial insights into additional experiments to inform next steps and strategy direction. For example, Netflix introduced a Top 10 row, hypothesizing that it would help members find content and increase satisfaction, as measured by engagement. It was a success in terms of its initial goals, and as the team tracked additional metrics in the experiment, it helped them understand related user behaviors (for example, how members used the home page) and design potential additional tests to explore continued improvements. These actionable insights for future iterations encourage a customer-centric approach to innovation.
Key Insight: Learning from Experimentation
“A repository allows the organization not only to track the state of any experimentation program but also to spread learning across the enterprise, which is crucial for hypothesis-driven innovation when a company is running a huge number of experiments each year.” [4]
When they become successful at experimenting at scale, companies can move past evaluating the results of individual tests to analyzing and learning from groups of experiments through “experimentation programs.” These programs can help to describe the performance of multiple product areas and identify potential future innovations. To take advantage of this learning, the authors propose creating a centralized “knowledge repository” to document and store results, track key performance indicators, and synthesize lessons across related experiments. The knowledge repository should be easy to access for all employees through dashboards or an AI assistant that can answer questions about experiments.
Why This Matters
For C-suite executives and business leaders, embracing a culture of experimentation is no longer optional—it’s a strategic imperative. The insights provided by Bojinov, Holtz, Johari, Schmit, and Tingley underscore the transformative power of scalable, democratized, and hypothesis-driven experimentation. By investing in the right tools, empowering employees, and institutionalizing knowledge-sharing, organizations can drive innovation by understanding both how and why certain experiments succeed or fail. As the authors conclude, companies can learn much more and “innovate and improve performance rapidly by testing all ideas—not just carefully vetted ones or only the big ones.” [5]
References
[1] Iavor Bojinov, David Holtz, Ramesh Johari, Sven Schmit, and Martin Tingley, “Want Your Company to Get Better at Experimentation? Learn Fast by Democratizing Testing”, Harvard Business Review (December 2024), https://hbr.org/2025/01/want-your-company-to-get-better-at-experimentation, accessed December 2024.
[2] Bojinov et al., “Want Your Company to Get Better at Experimentation? Learn Fast by Democratizing Testing”, https://hbr.org/2025/01/want-your-company-to-get-better-at-experimentation.
[3] Bojinov et al., “Want Your Company to Get Better at Experimentation? Learn Fast by Democratizing Testing”, https://hbr.org/2025/01/want-your-company-to-get-better-at-experimentation.
[4] Bojinov et al., “Want Your Company to Get Better at Experimentation? Learn Fast by Democratizing Testing”, https://hbr.org/2025/01/want-your-company-to-get-better-at-experimentation.
[5] Bojinov et al., “Want Your Company to Get Better at Experimentation? Learn Fast by Democratizing Testing”, https://hbr.org/2025/01/want-your-company-to-get-better-at-experimentation.
Meet the Authors
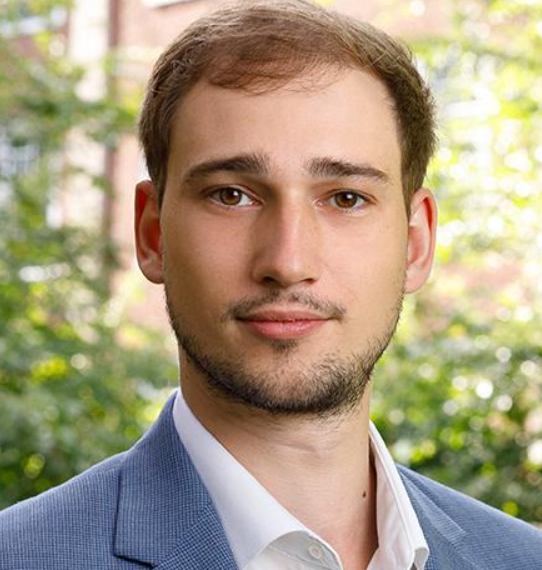
Iavor Bojinov is an Assistant Professor of Business Administration and the Richard Hodgson Fellow at HBS, as well as a faculty PI at D^3’s Data Science and AI Operations Lab and a faculty affiliate in the Department of Statistics at Harvard University and the Harvard Data Science Initiative. His research focuses on developing novel statistical methodologies to make business experimentation more rigorous, safer, and efficient, specifically homing in on the application of experimentation to the operationalization of artificial intelligence (AI), the process by which AI products are developed and integrated into real-world applications.
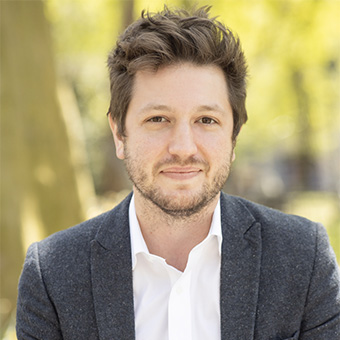
David Holtz is an Assistant Professor in the Management of Organizations (MORS) and Entrepreneurship and Innovation groups at the Haas School of Business, University of California, Berkeley. He earned his PhD at the MIT Sloan School of Management, in the Information Technology (IT) group. He also holds an MA in Physics and Astronomy from Johns Hopkins University, and a BA in Physics from Princeton University.
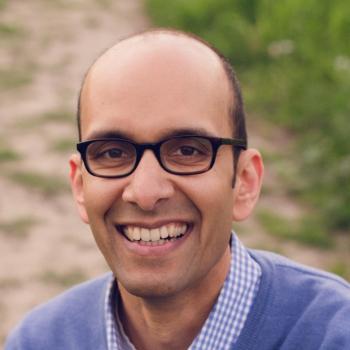
Ramesh Johari is a Professor of Management Science and Engineering at Stanford University. He is broadly interested in the design, economic analysis, and operation of online platforms, as well as statistical and machine learning techniques used by these platforms (such as search, recommendation, matching, and pricing algorithms).
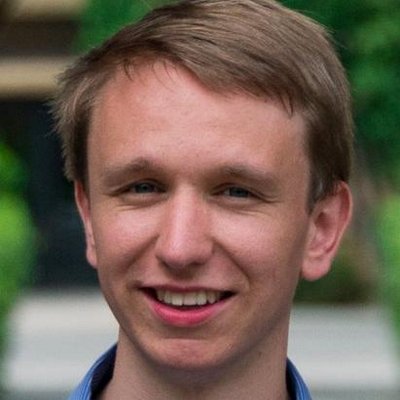
Sven Schmit is the Head of Statistics Engineering at Eppo, an experimentation and feature management platform that makes advanced A/B testing accessible. He obtained his PhD at Stanford while working with Ramesh Johari. Prior to his time at Eppo, he led the Core Representation Learning team at Stitch Fix.
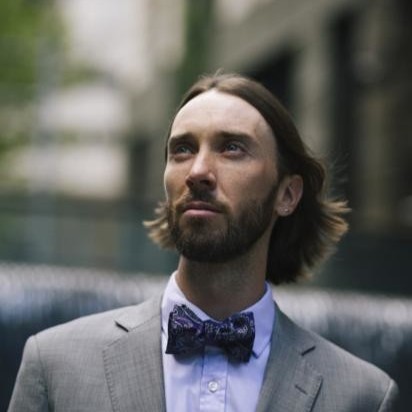
Martin Tingley is the Head of the Experimentation Platform Analysis Team at Netflix. Prior to his work at Netflix, he was an Assistant Professor at Penn State University and Principal Statistician at IAG. Tingley completed his PhD at Harvard University in Earth and Planetary Sciences.