In an era of data-driven business decision-making, the ability to design experiments that produce reliable, actionable results is essential. In their research, “Anytime-Valid Inference in Linear Models and Regression-Adjusted Causal Inference”, first published in 2022 and updated in 2024, Michael Lindon, research scientist at Netflix; Dae Woong Ham, Assistant Professor at University of Michigan’s Ross School; Martin Tingley, Head of the Experimentation Platform Analysis Team at Netflix; and Iavor Bojinov, Assistant Professor of Business Administration at HBS and PI at D^3’s Data Science and AI Operations Lab, explore “anytime-valid inference”, an approach that enhances statistical rigor in A/B testing while opening the door to more agile experimentation.
Key Insight: The Basics – Fixed-N Tests, Sequential Analysis, and Anytime-Valid Inference
“Sequential analysis is a natural solution for technology companies performing A/B tests over streams of outcomes.” [1]
A/B testing that uses fixed-n tests1 analyzes data at a pre-set sample size, which becomes a challenge in fast-paced environments where data is continuously generated. Sequential analysis2 solves this by allowing ongoing evaluation as data arrives, but it risks inflating Type-I errors (false positives)3 because, if you repeatedly apply a fixed-sample-size hypothesis test to the same data as it accumulates, each test provides another opportunity for a false positive. This research paper introduces the anytime-valid inference method to tackle this issue.
Key Insight: An Innovative and Simple Solution to Dynamic Testing
“Detecting differences as early as possible necessitates the use of anytime-valid methods.” [2]
The team’s anytime-valid inference method allows researchers to monitor experiments as they unfold, allowing early stopping when strong effects are evident, all while maintaining strict statistical rigor. The approach is both innovative and straightforward, as it is easily accessible and implementable for researchers and practitioners familiar with standard linear regression analysis.
The Netflix case study in the paper highlights how regression-adjusted sequential tests required half the sample size of standard methods, allowing the company to detect performance improvements faster and deploy optimized software sooner.
Key Insight: How Anytime-Valid Inference Works
“Using bootstrap resampling from the empirical distribution of the control data, we demonstrate that the Type-I error remains below the nominally chosen α.” [3]
Anytime-valid inference is a statistical method that lets researchers check the results of an A/B test as the data comes in, without increasing the chance of a false positive. To put it in technical terms, it utilizes a mixture-martingale approach4 or, equivalently, a Bayes factor5 derived from a specific prior, to construct sequential tests6 and confidence sequences that maintain Type-I error and coverage guarantees at all sample sizes, allowing for continuous monitoring of experiments without inflating the risk of Type-I errors.
Key Insight: Addressing the Replication Crisis
“It is estimated that only one-third of statistically significant results published in top psychology journals can be reproduced in follow-up experiments.” [4]
While the Netflix case study demonstrates the practical value of anytime-valid inference in a specific business context, the underlying statistical methods are widely applicable. In fact, the researchers point out that the approach could help solve the replication crisis in some scientific-research practices by tackling a core statistical problem that contributes to the issue. The anytime-valid inference method, by providing guarantees that hold regardless of when the analysis is performed, can help safeguard research against spurious results.
Why This Matters
For business professionals and C-suite executives, this research has profound implications. Continuous, statistically valid A/B testing without fixed sample sizes can offer unprecedented and easy-to-implement flexibility, allowing companies of all sizes to:
- Respond quickly to market changes by detecting significant effects earlier
- Optimize resources by stopping tests as soon as results are conclusive
- Increase confidence in results, reducing the risk of false positives
- Improve experimentation efficiency, enabling faster innovation cycles
In an era where data is a competitive advantage, this innovation in statistical methodology provides a valuable tool for extracting reliable and timely insights. By merging academic rigor with practical use, this research can transform how companies approach A/B testing and make strategic decisions.
Endnotes
1) A fixed-n test is a traditional approach to A/B testing where the sample size (n) is determined before the experiment begins. This predetermined sample size is critical to maintaining the statistical validity of the test, specifically in ensuring that the probability of a Type-I error (a false positive) stays at or below a pre-specified level, often denoted as alpha (α).
2) Sequential analysis is a statistical method that analyzes data as it becomes available, allowing for continuous monitoring of experiments and the possibility of early stopping.
3) A Type-I error is a fundamental concept in statistical hypothesis testing, particularly relevant in the context of A/B testing. Type-I errors occur when researchers incorrectly reject the null hypothesis, which happens when the data suggests a statistically significant difference or effect, but in reality, there is no true difference between the groups or conditions being compared. In simpler terms, a Type-I error is a false positive. It’s like concluding that a new marketing campaign increased sales when, in fact, any observed increase was due to random fluctuations rather than the campaign itself.
4) A mixture-martingale, as used in anytime-valid inference, is a mathematical tool that combines a sequence of probability ratios (which compare the likelihood of observed data under different hypotheses) with a specific probability distribution (called a mixture) over the possible values of the parameters being tested.
5) Bayes factor is a test statistic that is invariant under specific transformations of the data and parameters. This invariance ensures that the test is robust to certain nuisance parameters.
6) A confidence sequence is a sequence of confidence intervals that shrink with increasing data, all containing the true parameter with a pre-specified probability.
References
[1] Michael Lindon, Dae Woong Ham, Martin Tingley, Iavor Bojinov, “Anytime-Valid Linear Models and Regression Adjusted Causal Inference in Randomized Experiments”, arXiv:2210.08589v4 [stat.ME] (February 8, 2024): 1-77, 7.
[2] Lindon et al., “Anytime-Valid Linear Models and Regression Adjusted Causal Inference in Randomized Experiments”, 30.
[3] Lindon et al., “Anytime-Valid Linear Models and Regression Adjusted Causal Inference in Randomized Experiments”, 4.
[4] Lindon et al., “Anytime-Valid Linear Models and Regression Adjusted Causal Inference in Randomized Experiments”, 2.
Meet the Authors
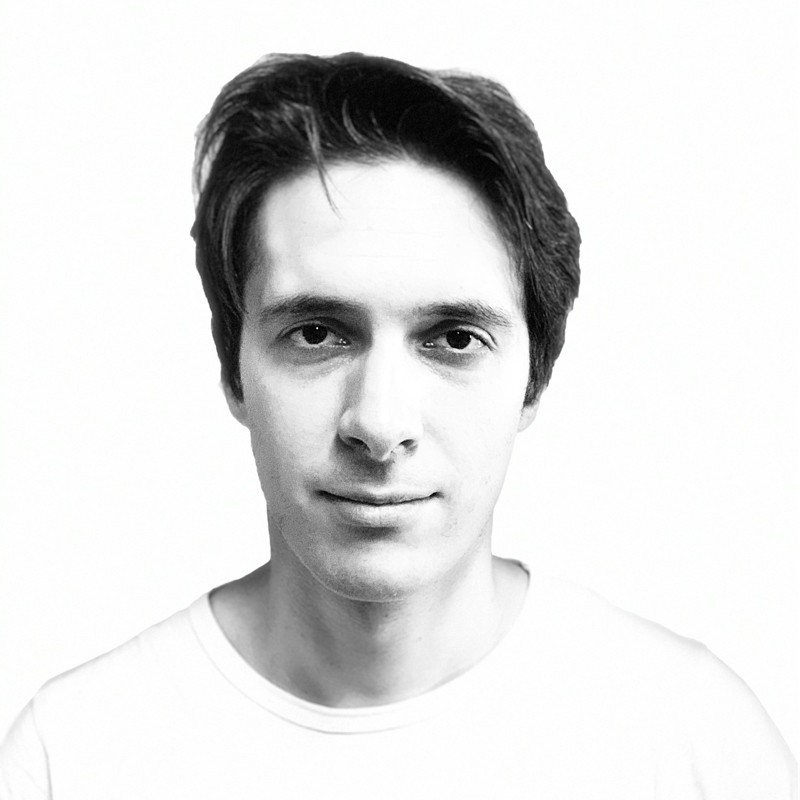
Michael Lindon is a Research Scientist at Netflix. Prior to his work at Netflix, he completed his PhD in Statistics at Duke University, and worked as a Statistician at Optimizely and as a Senior Data Scientist at Tesla.
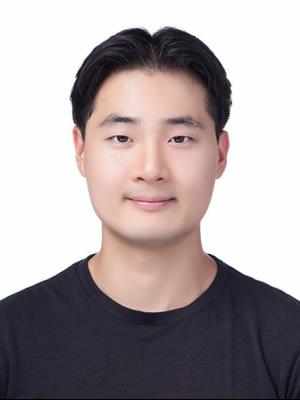
Dae Woong Ham is an Assistant Professor of Technology and Operations at University of Michigan’s Ross School. He conducts research in the intersection of causal inference and business/social science applications. He obtained his Ph.D. from the Harvard Statistics Department.
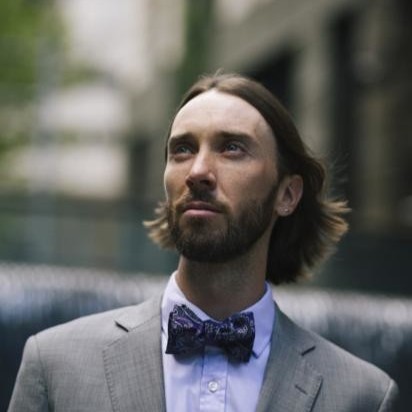
Martin Tingley is the Head of the Experimentation Platform Analysis Team at Netflix. Prior to his work at Netflix, he was an Assistant Professor at Penn State University and Principal Statistician at IAG. Tingley completed his PhD at Harvard University in Earth and Planetary Sciences.
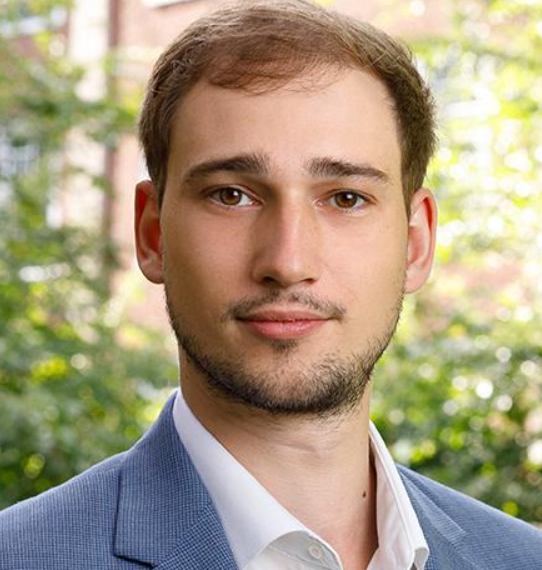
Iavor Bojinov is an Assistant Professor of Business Administration and the Richard Hodgson Fellow at HBS, as well as a faculty PI at D^3’s Data Science and AI Operations Lab and a faculty affiliate in the Department of Statistics at Harvard University and the Harvard Data Science Initiative. His research focuses on developing novel statistical methodologies to make business experimentation more rigorous, safer, and efficient, specifically homing in on the application of experimentation to the operationalization of artificial intelligence (AI), the process by which AI products are developed and integrated into real-world applications.