As businesses grapple with an ever-growing volume of ideas, products, and solutions to evaluate, decision-making processes are being reshaped by artificial intelligence (AI). Generative AI, in particular, has emerged as a game-changer in creative problem-solving and evaluation, as demonstrated by a recent field experiment described in the working paper “The Narrative AI Advantage? A Field Experiment on Generative AI-Augmented Evaluations of Early-Stage Innovations.”
The paper—by Jacqueline N. Lane, Assistant Professor at Harvard Business School and a co-Principal Investigator of the Laboratory for Innovation Science (LISH) at Harvard’s Digital Data Design Institute (D^3) and a team of researchers (see Meet the Authors section below for details)—describes how AI can augment decision-making for early-stage innovation screening.
The experiment, conducted with MIT Solve, included 72 experts and 156 non-expert community screeners who evaluated 48 solutions submitted to the 2024 Global Health Equity Challenge. The team used the GPT-4 large language model (LLM) to recommend whether to pass or fail each idea and provide criteria for failure. The evaluation phase was designed with three conditions:
- A human-only control condition, with no AI assistance
- Treatment 1: black box AI (BBAI), AI recommendations without rationale
- Treatment 2: Narrative AI (NAI), AI recommendations with rationale
Key Insight: AI-Augmented Decisions Are More Stringent
“Screeners were 9 percentage points more likely to fail a solution under the treatment conditions than the control condition.” [1]
Generative AI can be a source of rigor in evaluation. According to the authors, evaluators using AI recommendations were more discerning in their decision-making compared to human-only groups. The study highlights that AI-assisted screeners tended to fail solutions more often than their human-only counterparts, particularly when using treatment 2, which provided detailed narratives justifying its recommendations.
The NAI approach stood out as particularly effective, especially for subjective criteria like quality or alignment with goals. The researchers observed that human screeners were significantly more likely to follow narrative AI’s recommendations because the rationale added credibility and context to its suggestions.
Key Insight: Balancing Objectivity and Subjectivity in AI Collaboration
“[E]ffective decision-making for subjective criteria requires human oversight and close collaboration with AI.” [2]
While AI excels at tasks requiring objective analysis, its role in subjective evaluations remains nuanced. The study revealed a marked difference in human alignment with AI recommendations based on whether the criteria were objective or subjective. For objective tasks, such as assessing technical feasibility, AI provided valuable consistency. However, for subjective tasks, such as evaluating novelty or aesthetics, human oversight was indispensable. The researchers noted that over-reliance on AI narratives for subjective decisions could sometimes lead to uncritical acceptance of its conclusions.
Key Insight: The Rise of AI Interaction Expertise
“[Our findings suggest] the emergence of a new form of expertise—AI interaction expertise—which involves effectively interpreting, questioning, and integrating AI-generated insights into decision-making processes.” [3]
The authors suggested that integrating AI into decision-making demands more than technical know-how; it requires “AI interaction expertise.” The paper emphasized that screeners who deeply engaged with AI recommendations—examining and, when necessary, challenging them—were better able to integrate AI insights into their decisions. This highlights a new skill set for the modern workforce: the ability to collaborate effectively with AI systems.
Why This Matters
The authors’ experiment and conclusions can help C-suite and business executives assess the value of using LLMs in decision-making, specifically by:
- Recognizing AI’s strengths and weaknesses related to objective and subjective decision-making criteria. LLMs can potentially be used to pre-screen decisions based on objective criteria, and send those results to human screeners. Decisions involving subjective criteria require close human-AI collaboration, where AI tools act as “sounding boards” that complement the decision-making process.
- Understanding the importance of AI interaction expertise in the workforce to interpret AI results and implementing AI training that highlights the value of human perspectives and the uses and risks of AI tools.
As is often the case in studies of the current state of generative AI tools, the authors concluded that “The key lies in leveraging LLMs as tools to augment human decision-making rather than replace it entirely.” [4]
References
[1] Jacqueline N. Lane, Léonard Boussioux, Charles Ayoubi, Ying Hao Chen, Camila Lin, Rebecca Spens, Pooja Wagh, and Pei-Hsin Wang, “The Narrative AI Advantage? A Field Experiment on Generative AI-Augmented Evaluations of Early-Stage Innovations”, Harvard Business School Working Paper 25-001 (2024): 1-60, 5.
[2] Lane, et al., “The Narrative AI Advantage? A Field Experiment on Generative AI-Augmented Evaluations of Early-Stage Innovations”, 33.
[3] Lane, et al., “The Narrative AI Advantage? A Field Experiment on Generative AI-Augmented Evaluations of Early-Stage Innovations”, 31.
[4] Lane, et al., “The Narrative AI Advantage? A Field Experiment on Generative AI-Augmented Evaluations of Early-Stage Innovations”, 36.
Meet the Authors
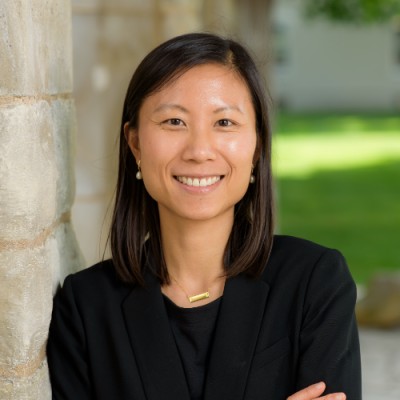
Jacqueline N. Lane is an Assistant Professor at Harvard Business School and a co-Principal Investigator of the Laboratory for Innovation Science (LISH) at Harvard’s Digital Data Design Institute (D^3). She earned her Ph.D. from Northwestern University.

Léonard Boussioux, is an Assistant Professor in the Department of Information Systems and Operations Management at the University of Washington, Foster School of Business, with an adjunct position at the Allen School of Computer Science and Engineering. He earned his Ph.D. in Operations Research at the Massachusetts Institute of Technology.

Charles Ayoubi is a Postdoctoral Research Fellow at the Laboratory for Innovation Science at Harvard (LISH) supported by a research grant from the Swiss National Science Foundation (SNSF). His research examines the processes of knowledge creation and diffusion in the context of science and innovation. He studies how scientists use their resources and informational advantages to achieve scientific breakthroughs, greater dissemination of knowledge and accessibility of innovation.

Ying Hao Chen is a Lecturer at the University of Washington Global Innovation Exchange.

Camila Lin is an AIOps Product Manager at Microsoft. Prior to her work at Microsoft, Lin earned her Master’s in Information Systems from the University of Washington where she worked as a Research Assistant.

Rebecca Spens is MIT Solve’s Results Measurement Manager and focuses on using research methods to understand Solve’s effectiveness and impact. Before joining Solve, Rebecca worked on evaluation and research in UK government, most recently at the Ministry of Justice. Rebecca holds a Master’s in Development Practice from Emory University and a BA in Modern History and French from the University of St. Andrews.

Pooja Wagh is Director, Operations & Impact at MIT Solve. Pooja came to Solve in 2017 with over a decade of experience in international development, program evaluation, and data analysis in the private and nonprofit sectors. Pooja holds a Masters in Public Policy from the Harvard Kennedy School and a Bachelors in electrical engineering from MIT.

Pei-Hsin Wang is a Cloud First Product Manager at Accenture. At the time of the research article’s publication, Wang was a Research Assistant and Data Scientist at the University of Washington.