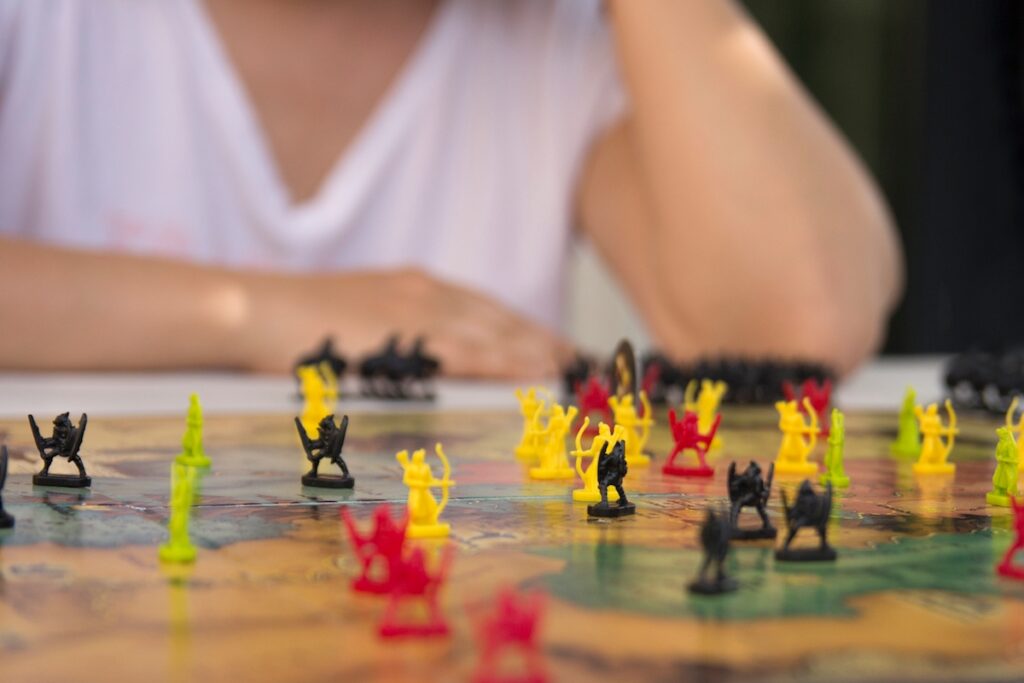
This article originally appeared in the Harvard Business Review.
Most major companies, including Google, Amazon, Microsoft, Uber, and Tesla, have had their artificial intelligence (AI) and machine learning (ML) systems tricked, evaded, or unintentionally misled. Yet despite these high profile failures, most organizations’ leaders are largely unaware of their own risk when creating and using AI and ML technologies. This is not entirely the fault of the businesses. Technical tools to limit and remediate damage have not been built as quickly as ML technology itself, existing cyber insurance generally doesn’t fully cover ML systems, and legal remedies (e.g., copyright, liability, and anti-hacking laws) may not cover such situations. An emerging solution is AI/ML-specific insurance. But who will need it and exactly what it will cover are still open questions.
Understanding risks
Recent events have shown that AI and ML systems are brittle and their failures can lead to real-world disasters. Our research, wherein we systematically studied AI failures published by the academic community, revealed that ML systems can fail in two ways: intentionally and unintentionally.
- In intentional failures an active adversary attempts to subvert the AI system to attain their goals of inferring private training data, stealing the underlying algorithm, or getting any desired output from the AI system. For example, when Tumblr announced its decision to stop hosting pornographic content, users bypassed the filter by coloring body images green and adding a picture of an owl — an example of a “perturbation attack.”
- In unintentional failures ML systems fail by their own accord without any adversarial tampering. For instance, OpenAI taught a machine learning system to play a boating game by rewarding its actions of getting a high score. However, the ML system went in a circle hitting the same targets, accruing more points, instead of finishing the race. One leading cause of unintentional failure is faulty assumptions by ML developers that produce a formally correct — but practically unsafe — outcome.
In its report on attacking machine learning models, Gartner issued a dire warning to executives: “Application leaders must anticipate and prepare to mitigate potential risks of data corruption, model theft, and adversarial samples.” But organizations are woefully underprepared. As the head of security of one of the largest banks in the United States told us, “We want to protect client information used in ML models but we don’t know how to get there.” The bank is not alone. When we informally interviewed 28 organizations spanning Fortune 500, small-and-medium businesses, non-profits, and government organizations we found that 25 of them didn’t have a plan in place to tackle adversarial attacks on their ML models. There were three reasons.
First, because AI failure modes are still an active and evolving area of research, it is not possible to provide prescriptive technological mitigations. For instance, recently researchers showed how 13 defenses for adversarial examples that were published in top academic journals are ineffective. Second, existing copyright, product liability, and U.S. “anti-hacking” statutes may not address all AI failure modes. Finally, since the primary modality through which ML and AI systems manipulate data is code and software, a natural place to turn for answers is classic cyber insurance. Yet discussions with insurance experts show that some AI failures may be covered by existing cyber insurance, but some may not.
Understanding the differences between cyber insurance and AI/ML insurance
To better understand the relationship between traditional cyber insurance and AI failure, we talked with a number of insurance experts. Broadly speaking, cyber insurance covers information security and privacy liability, and business interruption. For example, AI failures resulting in business interruption and breach of private information are most likely covered by existing cyber insurance, but AI failures resulting in brand damage, bodily harm, and property damage will not likely be covered by existing cyber insurance. Here’s how this breaks down.
Cyber insurance typically covers these common failures:
- Model Stealing Attacks: For example, OpenAI recently created an AI system to automatically generate text but did not initially completely disclose the underlying model on the grounds that it could be misused to spread disinformation. However, two researchers were able to recreate the algorithm and released it, before OpenAI released the full model. Attacks like these demonstrate how businesses could incur brand damage and intellectual property losses because of fallible AI systems. In this case, cyber insurance may hypothetically cover the scenario as there was a breach of private information.
- Data Leakage: For example, researchers were able to reconstruct faces with just the name of a person and access to a facial recognition system. This was so effective that people were able to use the reconstructed photo to identify an individual from a line-up with up to 87% accuracy. If this were to happen in real life, cyber insurance may be able to help as this could be a breach of private information, which in this case is the private training data.
However, cyber insurance does not typically cover these real-life AI/ML failures:
- Bodily Harm: Uber’s self-driving car killed a pedestrian in Arizona because its machine learning system failed to account for jaywalking. This event would likely not be covered by cyber insurance as its roots are in financial line insurance which has historically avoided such liabilities. When bodily harm occurs because of an AI failure — either by a package delivering drone or in the case of autonomous cars, when image recognition systems fail to perform in snow, fog, or frost conditions, cyber insurance is not likely to cover the damage (although it may cover the losses from the interruption of business that results from such events). In this event’s aftermath, Uber ceased its testing of self-driving cars in Arizona, Pennsylvania, and California. For any types of losses that Uber incurred because of business interruption, cyber insurance may apply, although it is unlikely it will apply for the bodily harm.
- Brand Damage: Consider a situation where company A uses a smart conversational bot designed by company B to promote company A’s brand on the Internet. If the bot goes awry, much like the poisoning attack on Microsoft’s Tay tweetbot, and brings about massive damage to company A’s brand, existing formulations of cyber insurance are less likely to cover company A’s losses. In another case, researchers tricked Cylance’s AI-based antivirus engine into thinking that a malicious piece of ransomware was benign. Should the company have suffered any brand damage as part of this attack, cyber insurance would likely not cover them.
- Damage to Physical Property: A paper by Google researchers poses the scenario wherein a cleaning robot uses reinforcement learning to explore its environment and learn the layout. As part of this exploration process, it inserts a wet mop into an electrical outlet which causes a fire. Should this example play out in real life, the cyber insurance of the maker of the cleaning robot will most likely not cover the loss.
Is It time for your company to purchase ML/AI insurance?
When organizations place machine learning systems at the center of their businesses, they introduce the risk of failures that could lead to a data breach, brand damage, property damage, business interruption, and in some cases, bodily harm. Even when companies are empowered to address AI failure modes, it is important to recognize that it is harder to be the defender than the attacker since the former needs to guard against all possible scenarios, while the latter only needs to find a single weakness to exploit. As a security manager at one of the big four consulting groups put it in an interview with us, “Traditional software attacks are a known unknown. Attacks on our ML models are unknown unknowns.”
Insurance companies are aware of this gap and are actively trying to reconcile the differences between traditional software style insurance and machine learning. Today, cyber insurance is the fastest growing insurance market targeting Small and Medium sized businesses and insurers want to sustain the momentum.
Given that AI adoption has tripled in the last 3 years, insurance providers see this as the next big market. Additionally, two major insurers pointed out that standards organizations such as ISO and NIST are in the process of formulating trustworthy AI frameworks. Moreover, countries are considering AI strategies and are so far emphasizing safety, security, and privacy of ML systems, with the EU leading the effort — all of this activity could lead to regulations in the future.
To get the best rates possible when AI insurance debuts, it is important to understand the options and start preparing now. We believe that AI insurance will first be available via major insurance carriers as bespoke insurers may not have sufficient safety nets to invest in new areas. From a pricing perspective, using the past cyber insurance market as a template, businesses can expect stringent requirements when AI insurance is introduced to limit the insurance provider’s liability with rates cooling off as the AI insurance market matures.
How to get started
To help managers get started, we put together an action plan to begin the conversation about securing, and insuring, machine learning models.
By next week:
- Start talking to your insurance provider about what will be covered and what will not, so that you are not operating with incorrect assumptions.
- Given the proliferation of AI systems in businesses, especially in large organizations it is important to first assess the potential impact of failure. We recommend taking stock of all the AI systems in the organization, and bucketing them based on a high, medium, and low criticality rating and then implementing insurance and protection measures accordingly.
By next month:
- Assign human oversight over business-critical decisions, rather than solely relying on automated systems.
- Perform table top exercises to account for failures in AI systems and assess the outcomes. We recommend evaluating your organization against the draft EU Trustworthy AI Guidelines especially Section 2 (Technical Robustness and Safety) and Section 3 (Privacy and Data Governance Checklist).
By next year:
- Assign a safety officer to assess the safety and security of AI systems. This would be a close collaboration between the Chief Information Security Officer and the Chief Data Officer’s personnel.
- Revamp security practices for the age of adversarial machine learning: update incident response playbooks and consider hiring a red team to stress test your ML systems.
AI and ML systems can help create large amounts of value for many organizations. However, as with any new technology, the risks must be understood — and mitigated — before the technology is fully integrated into the organization’s value creation process.
Editor’s note: This article has been updated to clarify the timeline of the release of OpenAI’s algorithm.