In the world of high-stakes business transactions, auctions play a crucial role in allocating resources efficiently. However, traditional auction methods often struggle with complexity, especially when dealing with multiple items or intricate buyer preferences. David Huang, PhD candidate in Quantitative Marketing at Harvard Business School; Francisco Marmolejo Cossío, Assistant Professor of Computer Science at Boston College and Postdoctoral Fellow at the Harvard School of Engineering and Applied Sciences; Edwin Lock, Postdoctoral Researcher at Oxford University; and David Parkes, Dean of the John A. Paulson School of Engineering and Applied Sciences and Faculty Co-Director at the Laboratory for Innovation Science at Harvard, looked at this issue in their recent study, “Accelerated Preference Elicitation with LLM-Based Proxies.” The study introduces an innovative approach that leverages AI to simplify and enhance the auction process, potentially transforming how businesses conduct large-scale transactions.
Key Insight: AI-Powered Proxies: A New Frontier in Auction Technology
“In our approach, LLM-powered proxies act as intermediaries in the elicitation process, interacting with the auction mechanism on behalf of the bidder. The proxies query the bidder through natural language interactions to uncover preferences.”[1]
Huang and his team developed a system that uses large language models (LLMs) as intermediaries between bidders and auctioneers. These AI proxies can communicate in natural language, making it easier for bidders to express complex preferences (elicitation) without getting bogged down in technical details. This approach addresses a long-standing challenge in combinatorial auctions, where participants bid on combinations of items (packages). Traditionally, these auctions require bidders to fully articulate their preferences – a task that can be cognitively demanding and time-consuming.
Key Insight: Accelerated Efficiency in Complex Markets
“Our experiments demonstrate that LLM-based proxies, particularly those that use LLMs to infer the person’s valuation on unspecified bundles, achieve approximately efficient auction outcomes up to five times faster than [traditional methods].” [2]
The study reveals that AI-powered proxies can dramatically speed up the auction process while maintaining or even improving efficiency. By inferring bidder preferences from natural language interactions, these systems can quickly arrive at near-optimal allocations without exhaustive questioning. In the researchers’ experiments, their AI proxy reached 75% efficiency in just two interactions with bidders, compared to around 10 interactions for traditional methods.
Key Insight: Bridging the Gap Between Theory and Practice
“Our LLM-powered proxy design mitigates [substantial cognitive burdens] through natural language interactions and inference, leading to more human-centric and efficient auction mechanisms in increasingly complex markets.” [3]
The team’s research addresses a critical disconnect between theoretical auction designs and practical implementation. While existing methods are mathematically sound, they often falter in real-world scenarios due to the cognitive burden placed on bidders. By introducing natural language processing and AI inference, this new approach makes complex auction mechanisms more accessible and user-friendly. The study demonstrated consistent performance across three diverse auction scenarios, including electronics, gourmet food items, and transportation equipment.
Key Insight: Robust and Adaptable AI Modeling
“We find that the preferences created by the LLM simulation pipeline are coherent. […] We find that the valuations given are precise. The variation for a given bundle is tight and the overall shape satisfies desirable properties.” [4]
A key concern with AI systems is their reliability and consistency. Huang’s team rigorously tested their AI models, demonstrating that the preferences generated were coherent and aligned with economic principles. The researchers validated their results across multiple AI models, including GPT and Google’s Gemini, showing consistent performance and interpretation of bidder preferences.
Why This Matters
For business leaders and executives, this research opens up new possibilities in resource allocation, procurement, and sales. The ability to conduct complex auctions more efficiently and with less friction could lead to significant cost savings and better outcomes in various industries. By making these processes more intuitive and less burdensome, companies can engage in more sophisticated market mechanisms without requiring extensive training or expertise from their staff. As markets become increasingly complex and fast-paced, tools that can quickly and accurately capture and act on nuanced preferences will be invaluable for strategic decision-making and maintaining a competitive edge.
References
[1] David Huang, Francisco Marmolejo Cossío, Edwin Lock, and David Parkes, “Accelerated Preference Elicitation with LLM-Based Proxies”, arXiv preprint arXiv:2501.14625 (January 4, 2025): 1.
[2] Huang et al., “Accelerated Preference Elicitation with LLM-Based Proxies”, 9.
[3] Huang et al. “Accelerated Preference Elicitation with LLM-Based Proxies”, 9.
[4] Huang et al., “Accelerated Preference Elicitation with LLM-Based Proxies”, 9.
Meet the Authors
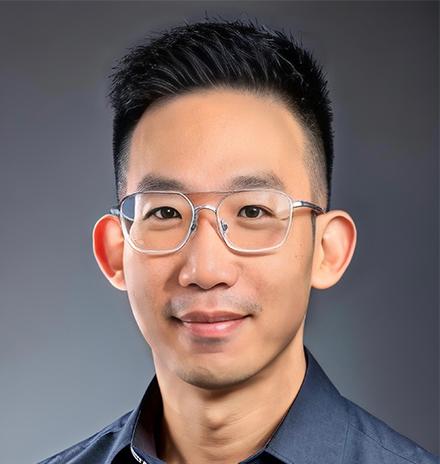
Ta-Wei (David) Huang is a PhD candidate in Quantitative Marketing at Harvard Business School. His research integrates causal inference and machine learning to address methodological challenges and unintended consequences in targeting, personalization, and online experimentation. David holds a BS in Quantitative Finance from National Tsing Hua University, Taiwan, and an MS in Statistics from National Taiwan University.
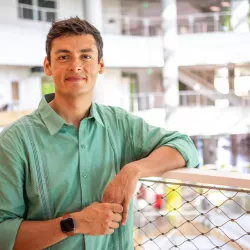
Francisco Marmolejo Cossío is an Assistant Professor of Computer Science at Boston College. He is currently the Director of Partnerships at the EAAMO research initiative, a Postdoctoral Fellow at the Harvard School of Engineering and Applied Sciences, and a Research Fellow at Input Output Global. He holds a DPhil in Computer Science from the University of Oxford, and a BA in Mathematics from Harvard University.
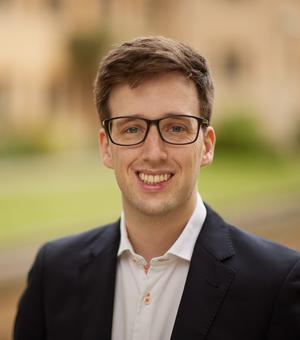
Edwin Lock is a postdoctoral researcher at the Department of Computer Science, Oxford University and an associate member at Nuffield College.
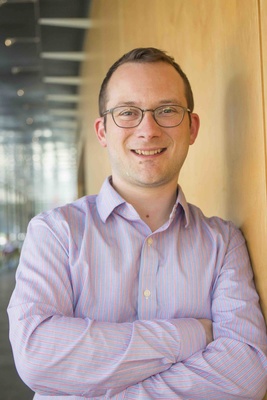
David Parkes is a Professor of Computer Science and Dean of Harvard John A. Paulson School of Engineering and Applied Sciences (SEAS) where he founded the EconCS research group. He is also a Faculty Co-Director at the Laboratory for Innovation Science (LISH) at Harvard. His current research interests are in market design, information and preference elicitation, modeling behavior, machine learning and economics, and computational social choice. Parkes has an MEng (first class) in Engineering and Computing Science from Oxford University and a PhD in Computer and Information Science from the University of Pennsylvania.