As artificial intelligence systems become more prevalent in our daily lives, ensuring these technologies are fair and representative of diverse populations is increasingly critical. A recent study, “Multi-Group Proportional Representation in Retrieval”, conducted by Flavio du Pin Calmon, an Associate Professor of Electrical Engineering at Harvard’s John A. Paulson School of Engineering and Applied Sciences, and his research group (see the bottom of the page for author details) at Harvard University, introduces an innovative approach to measuring and promoting diversity in AI image retrieval systems. Their work addresses a key challenge in the field: how to ensure retrieved images reflect the true diversity of society across multiple intersecting demographic groups.
Key Insight: Current Approaches Fall Short on Intersectional Representation
“Ensuring representation across individual groups (e.g., given by gender or race) does not guarantee representation across intersectional groups (e.g., given by gender and race).” [1]
The researchers found that existing methods for promoting diversity in image retrieval often focus on balancing representation across a small number of pre-defined groups, typically based on single attributes like gender or race. However, they argue that this approach fails to account for intersectional groups, those defined by multiple overlapping attributes. For example, a system may retrieve an equal number of men and women but still under-represent women of color. The study demonstrates that optimizing for individual group representation does not necessarily lead to fair representation of intersectional groups.
Key Insight: A New Metric for Multi-Group Representation
“We propose a metric called Multi-group Proportional Representation (MPR) to quantify the representation of intersectional groups in retrieval tasks. MPR measures the worst-case deviation between the average values of a collection of representation statistics computed over retrieved items relative to a reference population whose representation we aim to match.” [2]
To address the current representation gap, the researchers developed a metric called Multi-Group Proportional Representation (MPR), which quantifies how well a set of retrieved images represents diverse intersectional groups compared to a reference population. Crucially, MPR can measure representation across a large or even infinite number of overlapping groups, defined by complex combinations of attributes. This allows for a much more nuanced and comprehensive assessment of diversity and representation.
Key Insight: Scalability and Flexibility
“MPR offers a more flexible, scalable, and theoretically grounded metric for multi-group representation in retrieval.” [3]
A key advantage of the MPR approach is its scalability and flexibility. Unlike methods that rely on pre-defined groups, MPR can handle an arbitrary number of intersectional groups defined by complex functions. The researchers provide theoretical guarantees on the sample complexity required to estimate MPR accurately. They also demonstrate how MPR can be efficiently computed for several practical function classes, including linear functions and decision trees. This makes MPR a powerful and adaptable tool for measuring and optimizing representation in large-scale retrieval systems.
Key Insight: Ethical and Practical Considerations
“There are legal and regulatory risks with overreliance on a single metric for fairness, especially if this metric is used to inform policy and decision-making.” [4]
While MPR is a powerful tool, the authors caution against viewing it as a standalone solution. Fairness is multidimensional, and overreliance on a single metric can lead to unintended consequences, such as reinforcing stereotypes or overlooking other forms of harm. Furthermore, the researchers warn that the deployment of MPR by companies could result in “ethics-washing”, where, even when systems exhibit representational harms, firms claim them to be fair based on their use of a fairness metric, like MPR. Finally, to ensure that the results of MPR are diverse, ethical, and fair, the researchers suggest utilizing datasets that are curated to guarantee they represent diverse populations. Failing to do so can result in propagating biases throughout the system.
Why This Matters
For C-suite executives, the introduction of Multi-Group Proportional Representation (MPR) signals a transformative step in aligning artificial intelligence systems with values of fairness and inclusivity. MPR tackles a critical shortfall in current AI practices: the failure to represent diverse intersectional groups in image retrieval and similar applications. By quantifying proportional representation, MPR offers a scalable and actionable framework for mitigating bias while preserving the functionality of the retrieval system.
Adopting MPR isn’t just an ethical responsibility, it’s a strategic imperative. Inclusive AI systems foster trust among consumers and employees, safeguard against reputational and regulatory risks, and enhance decision-making by accurately reflecting the diversity of society. With tools like MPR and the Multi-group Optimized Proportional Retrieval (MOPR) algorithm, organizations can lead in embedding fairness into their technological foundations, transforming inclusivity from a compliance checkbox into a competitive advantage.
References
[1] Alex Osterling, Claudio Mayrink Verdun, Carol Xuan Long, Alexander Glynn, Lucas Monteiro Paes, Sajani Vithana, Martina Cardone, and Flavio du Pin Calmon, “Multi-Group Proportional Representation in Retrieval,” arXiv preprint arXiv:2407.08571 (2024): 1-48, 2.
[2] Alex Osterling et al., “Multi-Group Proportional Representation in Retrieval”, 2.
[3] Alex Osterling et al., “Multi-Group Proportional Representation in Retrieval”, 2.
[4] Alex Osterling et al., “Multi-Group Proportional Representation in Retrieval”, 5.
Meet the Authors
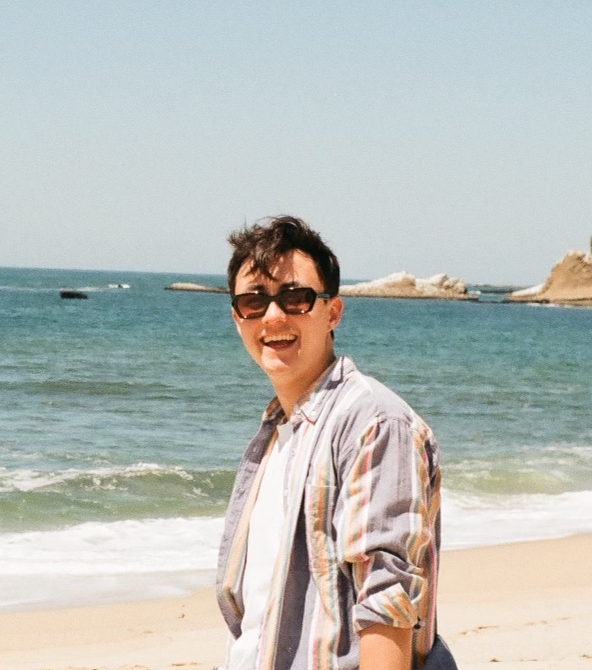
Alex Osterling is a PhD student at Harvard under the mentorship of Dr. Flavio du Pin Calmon and Dr. Hima Lakkaraju and is supported by the NSF Graduate Student Research Fellowship. They are broadly interested in fair, interpretable, and trustworthy machine learning, and their current projects apply information theoretic tools to problems in fairness and representation learning.
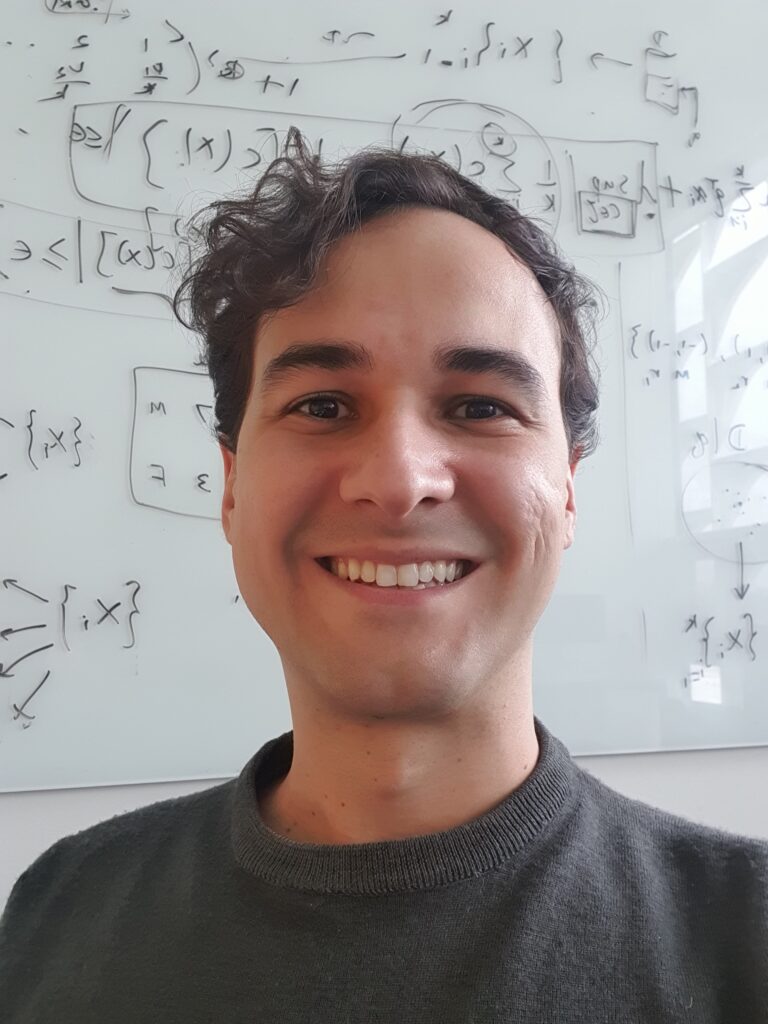
Claudio Mayrink Verdun is a mathematician working with mathematics of AI and machine learning at Harvard’s School of Engineering and Applied Sciences under the mentorship of Flavio Calmon. His research focuses on trustworthy machine learning, exploring concepts such as fairness and arbitrariness, and also on mechanistic interpretability techniques for large generative models.
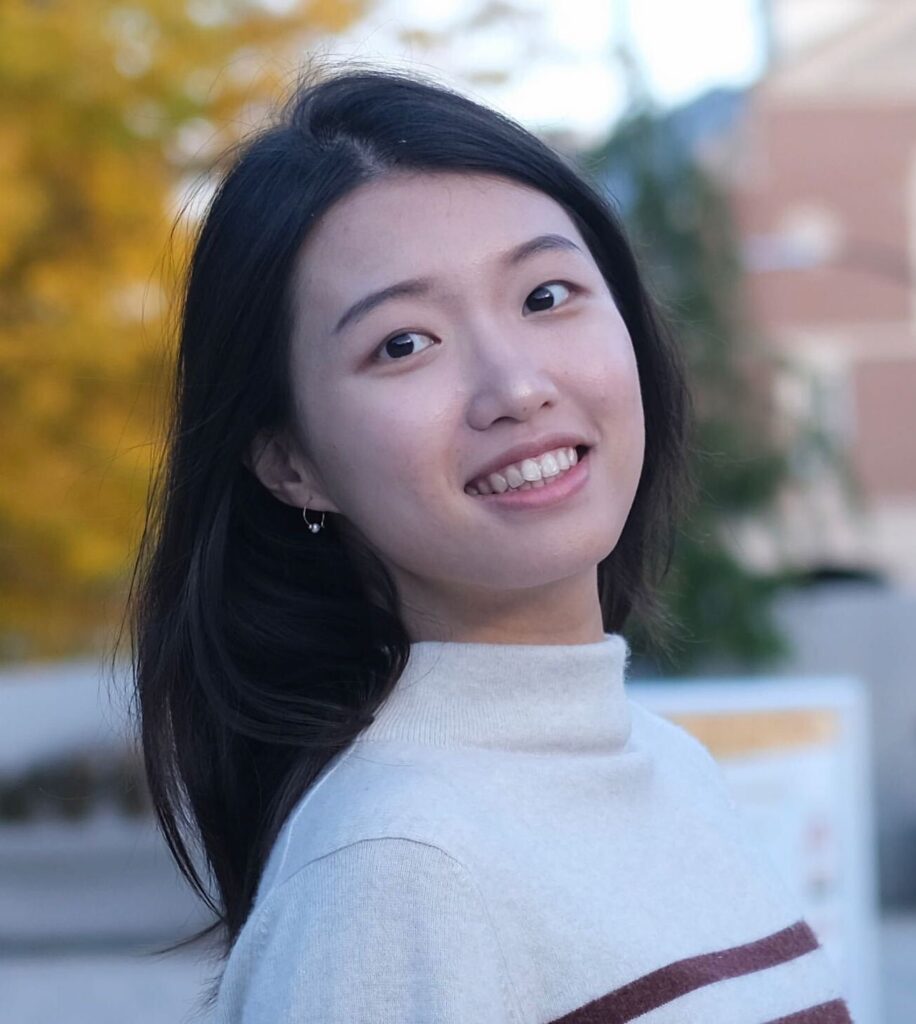
Carol Xuan Long is a 4th-year Ph.D. student at Harvard, advised by Flavio du Pin Calmon. She completed her undergraduate degree in Math and Computer Science at NYU Courant and previously interned at Citadel LLC and Meta. Her research interest lies in Responsible and Trustworthy Machine Learning, and her work spans LLM watermarking, algorithmic fairness, multiplicity, and more.
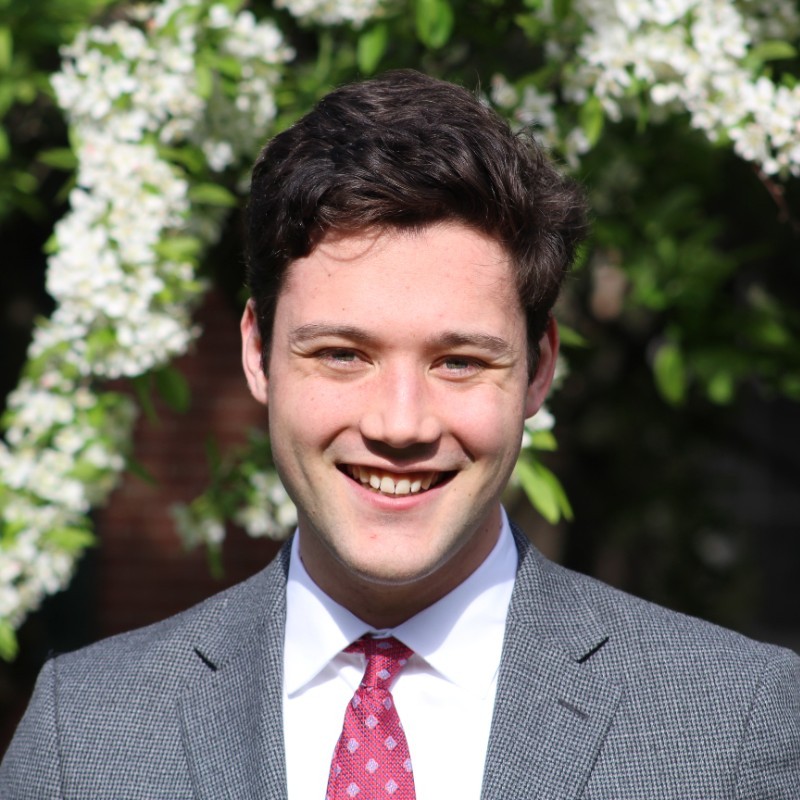
Alexander Glynn has a degree in Applied Mathematics from Harvard University, and completed a research fellowship at the Harvard John A. Paulson School of Engineering and Applied Sciences focused on developing software pipelines to analyze and audit algorithms with novel techniques. He currently works as a Data Scientist at C3 AI.
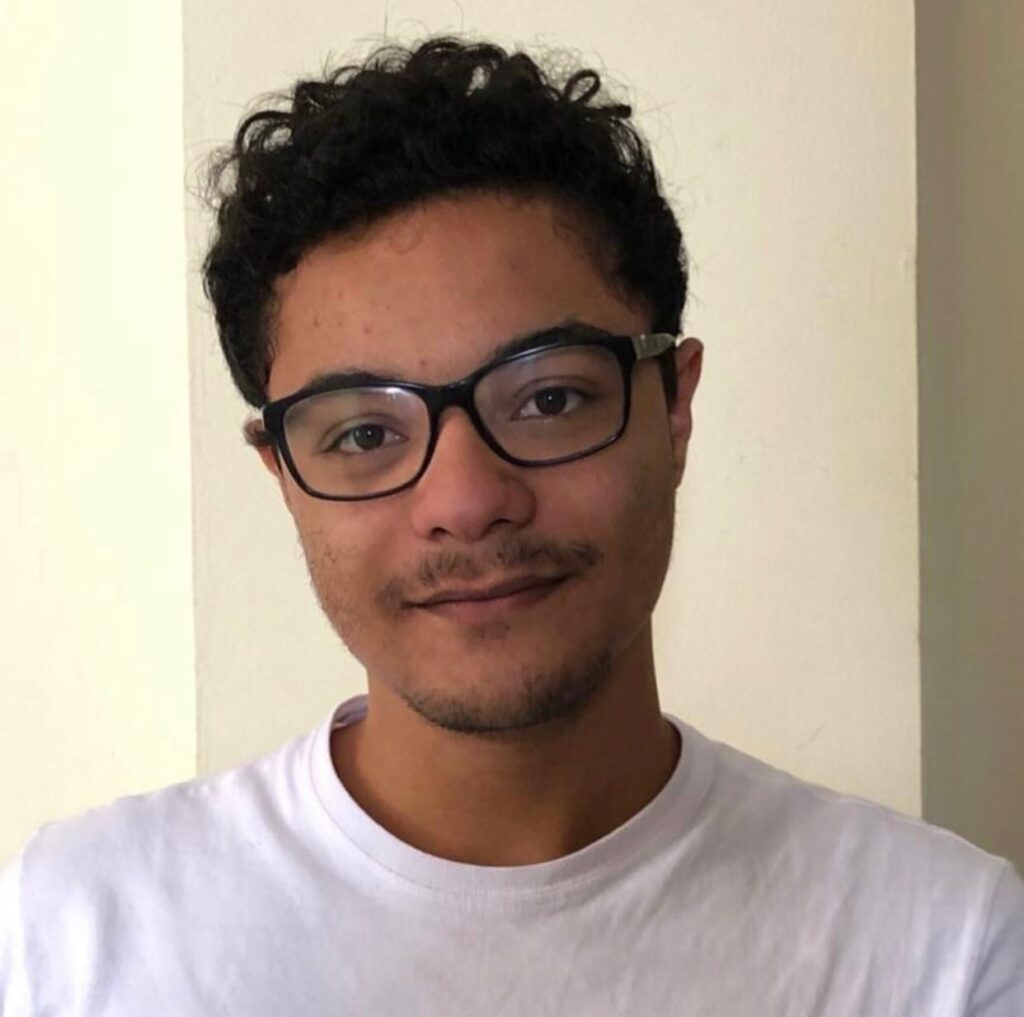
Lucas Monteiro Paes is an Applied Mathematics Ph.D. candidate at Harvard University SEAS working with Prof. Flavio Calmon and a student researcher at Google DeepMind in the Gemini Safety Team. He uses theoretical insights to develop safe and trustworthy AI and ML systems. Their research is driven by the belief that AI and ML systems should not only be accurate and efficient but also transparent, fair, and aligned with human values and societal norms.
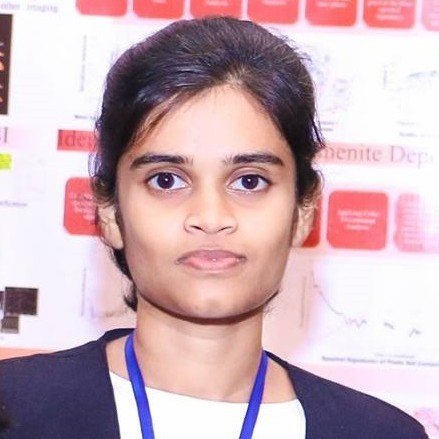
Sajani Vithana is a Postdoctoral Research Fellow at the Harvard John A. Paulson School of Engineering and Applied Sciences. Her research interests include information theory, private information retrieval, and machine learning.
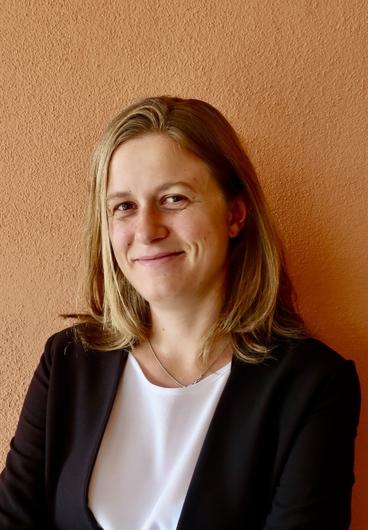
Martina Cardone works in the Electrical and Computer Engineering Department at the University of Minnesota as an Assistant Professor. From July 2015 to August 2017, she was a post-doctoral research fellow in the Electrical and Computer Engineering Department at UCLA Henry Samueli School. She received her B.Sc. and M.Sc. from Politecnico di Torino in 2009 and 2011, respectively. As part of a Double Degree program, in 2011 she also earned a M.Sc. from Télécom ParisTech – EURECOM. She completed her Ph.D. in Electronics and Communications at EURECOM – Télécom ParisTech.
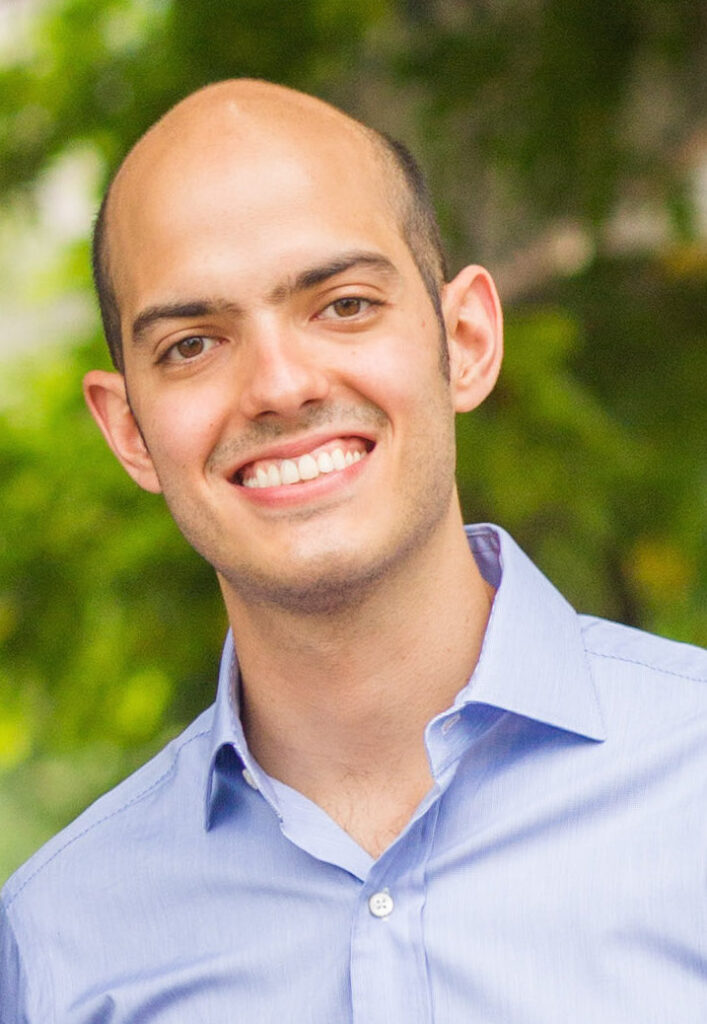
Flavio du Pin Calmon is an Associate Professor of Electrical Engineering at Harvard’s John A. Paulson School of Engineering and Applied Sciences. Before joining Harvard he was a social good post-doctoral fellow at IBM Research in Yorktown Heights, New York. He received his Ph.D. in Electrical Engineering and Computer Science at MIT. His main research interests are information theory, signal processing, and machine learning.