Artificial Intelligence Could Become Your Surgeon’s Best Friend: Why Should You Care?
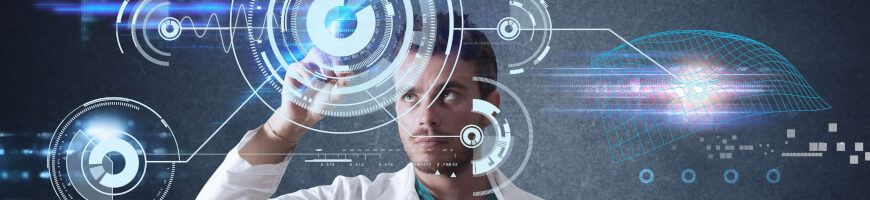
Machine learning can improve the quality of surgical care while reducing the cost – how is this possible and what should you expect in the next decade?
In the United States, 1.5 million patients develop postoperative complications and 150,000 die within thirty-days after surgery every year.(1-3)In the healthcare value equation defined by Porter, postoperative complications not only decrease quality but significantly increase costs.(4)
Figure by Bill Coyle, https://www.pm360online.com/how-do-you-define-value-in-healthcare/
At the University of Florida (UF), Bihorac et al. developed an integrated system for applying machine learning to preoperatively predict postoperative risk.(1)The system predicts postoperative mortality at 1, 3, 6, 12, and 24 months after surgery and it additionally estimates risk of postoperative acute kidney injury, intensive care unit admission for greater than 48 hours, mechanical ventilation for greater than 48 hours, wound complications, cardiovascular complications, neurologic complications, sepsis, and venous thromboembolism.
Figure from Annals of Surgery, Bihorac et al. 2018
Other organizations such as the American College of Surgeons (ACS) previously developed preoperative algorithms, including the ACS National Surgical Quality Improvement Program Risk Calculator (https://riskcalculator.facs.org/RiskCalculator/).(5) External calculators are helpful but they exist outside of the integrated EHR for most hospitals and require clinicians to interrupt their usual workflow and manually enter patient information into these separate entities. The unique features of the MySurgeryRisk platform developed at UF are seamless integration into the clinical electronic health records (EHR).
Understanding why this seamless integration is so important requires assessment of the trajectory of machine learning (ML) and artificial intelligence (AI) in healthcare. Fundamentally, ML can be simplified into 1. Input 2. Transformation Function 3. Output. With electronic health records, the 4 V’s of big data: volume, velocity, variety, and veracity are the Inputs. Transformation Functions can range from simple statistics (regression) to more flexible shallow machine learning models to deep learning with convolutional neural networks. Currently, MySurgeryRisk uses a machine learning generalized additive model. Finally, the Output can be delivered as both prediction and explanation. For now, MySurgeryRisk provides only prediction.
Figure by Author
MySurgeryRisk gathers structured data fields and unstructured free text notes from the institutional EHR and public databases. The next step in the development of this platform will be improvements on the Inputs with integration of Sensor data, Omics (everything from the genome to the microbiome), unstructured imaging data in the form of Videos and Images, and large publicly available datasets. Simultaneously, the Transformation Function of the MySurgeryRisk platform will need to be updated to keep up with the progress in deep learning achieved for dermatology, radiology, ophthalmology, and pathology.(6-9)In addition, the Output of the MySurgeryRisk will need to provide explanation of the predictions made by the algorithm so that clinicians have increased transparency into the factors used by MySurgeryRisk for prediction as well as their relative impact in generating the estimated prediction.
In the long-term, if MySurgeryRisk can evolve to include the advancements noted above, widespread use of such a system could significantly improve postoperative outcomes without requiring clinicians to depart from the usual surgical workflow. Imagine a system like MySurgeryRisk that runs in the background and automatically pulls data from the EHR, from picture archiving and communication systems (PACS), from hospital Omics repositories, and from publicly available sources. The system continuously generates and updates predictions and explanations for each patient’s postoperative trajectory as the data for the patient builds up in the form of intake demographic forms, clinical evaluations by nurses, physicians and other healthcare providers, laboratory tests, and imaging. Whenever clinicians are ready to make an assessment and plan for the patient, they access the system from directly within the EHR and examine the patient’s predicted risk without having to manually type in any information or navigate to external platforms. In fact, with seamless integration, such a system may be able to deliver insights simultaneously into drafts of clinical documents and help to pre-populate the documentation required for each patient, creating additional time savings for clinicians who can then spend more time engaging with patients. Critical decisions about the timing of surgery, invasiveness of surgery, intraoperative management, and preoperative planning for the expected postoperative course could be improved by generating insights for clinicians in real-time.
Additional difficulties that remain for widespread adoption of MySurgeryRisk more broadly include the diversity of data organization from one health center to another. Within the United States, will MySurgeryRisk be able to generalize from a large healthcare center to another or will the system need to be re-trained for the idiosyncrasies of every new hospital and organization? How will the system cope with systems both inside and outside the United States that rely on a combination of paper based and EHR documentation? Finally, even if this system is accessible, clinicians will need training to understand the most appropriate and efficient uses and who will be responsible for delivering training that is helpful for learners of all backgrounds?
—
Aditya V. Karhade, akarhade@mba2020.hbs.edu
References:
- Bihorac A, Ozrazgat-Baslanti T, Ebadi A, Motaei A, Madkour M, Pardalos PM, et al. MySurgeryRisk: Development and Validation of a Machine-learning Risk Algorithm for Major Complications and Death After Surgery. Annals of surgery. 2018.
- Weiser TG, Regenbogen SE, Thompson KD, Haynes AB, Lipsitz SR, Berry WR, et al. An estimation of the global volume of surgery: a modelling strategy based on available data. Lancet (London, England). 2008;372(9633):139-44.
- Weiser TG, Haynes AB, Molina G, Lipsitz SR, Esquivel MM, Uribe-Leitz T, et al. Estimate of the global volume of surgery in 2012: an assessment supporting improved health outcomes. Lancet (London, England). 2015;385 Suppl 2:S11.
- Porter ME. What is value in health care? The New England journal of medicine. 2010;363(26):2477-81.
- Bilimoria KY, Liu Y, Paruch JL, Zhou L, Kmiecik TE, Ko CY, et al. Development and evaluation of the universal ACS NSQIP surgical risk calculator: a decision aid and informed consent tool for patients and surgeons. Journal of the American College of Surgeons. 2013;217(5):833-42. e3.
- Esteva A, Kuprel B, Novoa RA, Ko J, Swetter SM, Blau HM, et al. Dermatologist-level classification of skin cancer with deep neural networks. Nature. 2017;542(7639):115.
- Gulshan V, Peng L, Coram M, Stumpe MC, Wu D, Narayanaswamy A, et al. Development and validation of a deep learning algorithm for detection of diabetic retinopathy in retinal fundus photographs. Jama. 2016;316(22):2402-10.
- Chilamkurthy S, Ghosh R, Tanamala S, Biviji M, Campeau NG, Venugopal VK, et al. Deep learning algorithms for detection of critical findings in head CT scans: a retrospective study. The Lancet. 2018.
- Obermeyer Z, Emanuel EJ. Predicting the future—big data, machine learning, and clinical medicine. The New England journal of medicine. 2016;375(13):1216.
Word Count: 784
Featured Image from The Medical Futurist: https://medicalfuturist.com/the-technological-future-of-surgery
This is fascinating. From your questions, it seems to me that the key issues facing MySurgeryRisk are scalability, as integration and training in new hospital systems and new contexts requires immense upfront costs. I give a lot of credit to the centralization of EMRs to making this technology possible though. While they’re not perfect, without centralized EMRs make the input data much cleaner and more consistent than they’d otherwise be.
Without clean, consistent input data, implementation just at the local level would be quite difficult, and scalability would seem infeasible.
Insightful write up, Aditya.
I’d like to elaborate more on your point about data veracity, since I think that its a crucial part of training your model with the correct inputs. In my article, I discuss about the impact of surgeon performance on post operative complication [1], which roughly equates to 50% [2] of the 150,000 deaths quoted in your article. All too often, many people in the EMR space who have tried to build comprehensive outcomes-based predictive models have suffered from bad data (or inaccurate data) that looses precision and accuracy as it scales to larger patient populations and other markets.
In this stride, I think a lot of caution needs to be taken into curating the kind of hospital system and surgeon data being fed into training data sets for MySurgeryRisk.
[1] https://d3.harvard.edu/platform-rctom/submission/collaborative-autonomy-in-the-operating-room-verb-surgical-and-democratized-surgery/
[2] https://www.ncbi.nlm.nih.gov/pmc/articles/PMC2639900/
Aditya, does this means MySurgeryRisk requires open source information in medical field as prerequisite? This model works well only when all medical history can be collected and stored into one place. However even we are trying to digitalize our medical information, most of them are kept confidential and independent from each hospital or clinics.
I agree with Zoey and believe that the key problem here is to get access to all the data from all the patients in order to provide a decent input to the machine learning algorithm to get a right output. Moreover, the algorithm would require approval/correction/disproval of type of decision /prediction it has made and knowing that in many way doctors could be subjective, I struggle with understanding of how the algorithm would learn what is right/what is wrong. Say, you run experiment in one clinic with limited number of patients and only one doctor “trains” the algorithm, then it will provide one type of output after some time. Same test but run un a different clinic guided by the doctor with the different opinion on some cases, could potentially lead to the different output from the algorithm after a while.
You make a very good point about how AI and Machine Learning can be applied to healthcare and how one of the key challenges is applying it uniformly across different platforms (hospitals, labs, physicians offices, etc.). I would be interested to see if physicians are able to “dig into” the output that MySurgeryRisk generates – for example, if the algorithm spits out a positive outcome, would a physician be able to see (or even update) the different factors that go into the algorithm in order to make it more precise? I’d be curious to see if this is an option and how physicians and their inputs can improve the MySurgeryRisk algorithm over time.
Well done, Didi! I liked your diagram and how you highlighted the nuances between the type of analyses that can be done with the data. I definitely think this is the way of the future in healthcare, though there are abundant barriers to adoption. I wonder what the process might look like if the doctor felt he needed to override the AI recommendation? Another piece that seems pertinent is what legalities are involved when you have AI making recommendations.