Collaborative autonomy in the Operating Room: Verb Surgical and Democratized Surgery
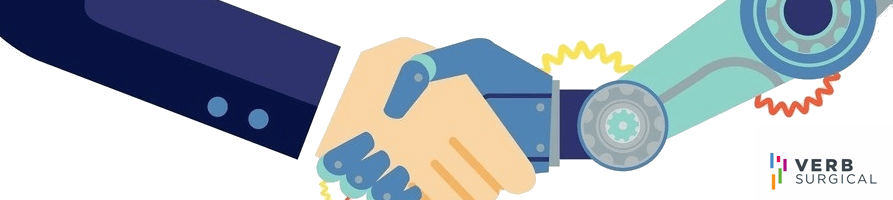
Can Verb Surgical use advanced robotics and machine learning to deliver high quality surgical care to the 5 billion [16] people around the world who desparately need it?
Machine Learning (ML) and Surgery 4.0
Recent advancements in ML for medicine have enabled physicians to leverage predictive tools for disease diagnosis (such as lung nodule detection [1]; and diabetic retinopathy screening [2]) and early intervention. However, most ML advancements have strayed away from interventional care, such as surgery, because insufficient data exists to benchmark existing and gold standards of care [3].
This gap is alarming, as medical error is the third leading cause of death in the US– upwards of 440,000 deaths occur annually [4], of which 17% are preventable (operator-induced) complications that occur during surgery [5].
Enter minimally invasive robotic surgery (MIRS), which promises to improve surgeon performance and patient safety — by enabling better anatomical access, instrument control and visualization through miniature incisions within the body. Robotics represents Surgery 3.0 (Figure 1) – a leapfrog technology that promises safer, higher quality-of-life post-operative outcomes compared to open surgery or laparoscopy [6]. However, MIRS is still competency/skill dependent and complex to learn [7]. Hence, the broader issue of surgeon-to-surgeon variability and its implications on patient outcome persist.
Verb Surgical aims to deliver surgery 4.0 to the 5 billion people [16] worldwide who desperately need care. To push this new mode of surgery into the operating room, Verb aims to develop a digital surgery ecosystem (DSE) around end-to-end patient care (Figure 3).
Industry and Clinical Partners for Data and Annotation
The answer lies in partnerships. Verb Surgical is a strategic partnership between Google and Johnson and Johnson (J&J). Google provides annotation frameworks that can be leveraged to train predictive models [1], while J&J provides the distribution channels within various hospital networks to acquire data at scale. However, no hospital or physician will adopt a system that does not provide clinical value today.
To address this issue, Verb Surgical is targeting procedures that enable surgeons to visualize anatomy in novel ways, and robotically operate on these structures if they so desire [10]. In many ways, they are benchmarking to Surgery 3.0, while delivering incremental DSE benefits to drive data-driven decision making for the surgeon – before, during and after a procedure [10]. Surgeons have been receptive to this strategy [10], and to Verb’s benefit, represent an early set of clinical adopters that will push data and annotation aggregation for broader surgery 4.0 adoption.
Verb’s biggest challenge to scale beyond early adopters is to overcome the heavy switching costs associated with acquiring DaVinci surgical users in the US (a robotic system manufactured by Intuitive Surgical®, with 88.8% market penetration [11]) and to sell their system to price-sensitive international users at a low capital cost [12]. The latter customers are currently inaccessible to Intuitive Surgical® (each system costs upwards of $2M USD [13]) but are high-value data opportunities for Verb (by surgical volume, these markets are 7x larger than the US [14]).
A Netflix Model for Surgery 4.0
How can Verb capture price-sensitive, high volume users quickly? Netflix may provide a clue. Hospitals typically take months to perform a cost-benefit-analysis before making a large capital purchase decision. What if hospitals got the robot for free, and paid an annual subscription after the first year of use? This enables purchasers to develop an economic value proposition unique to their hospital system, with no capital downside. This is an emerging model garnering broader adoption across other device companies [15], and to Verb, presents a unique long-term monetization opportunity synergistic with surgery 4.0 (Data Products, Figure 3).
2030 and Beyond – Collaborative Autonomy
Fast forward a decade from now. Verb has every high-volume surgical market annotated, constantly refining predictive models that know how to execute the perfect procedure for any patient context. Surgeons in rural China, with the help of intelligent guidance systems (Figure 4), can perform nephrectomies (removal of cancerous kidney) as well as their academic counterparts abroad.

Is this paradigm of care attainable? Can sensors be placed within the body to characterize instrument and anatomic interactions with sufficient accuracy to warrant robust predictive power from a machine? (800 Words)
References
[1] Nam, Ju Gang, Sunggyun Park, Eui Jin Hwang, Jong Hyuk Lee, Kwang-Nam Jin, Kun Young Lim, and Thienkai Huy Vu et al. “Development And Validation Of Deep Learning–Based Automatic Detection Algorithm For Malignant Pulmonary Nodules On Chest Radiographs”. Radiology, 2018, 180237. doi:10.1148/radiol.2018180237.
[2] Gulshan, Varun et al. 2016. “Development and Validation of a Deep Learning Algorithm for Detection of Diabetic Retinopathy in Retinal Fundus Photographs”. JAMA 316 (22): 2402. American Medical Association (AMA). doi:10.1001/jama.2016.17216.
[3] Fecso, Andras B. et al. 2017. “The Effect of Technical Performance on Patient Outcomes in Surgery”. Annals of Surgery 265 (3): 492-501. Ovid Technologies (Wolters Kluwer Health). doi:10.1097/sla.0000000000001959.
[4] Makary, Martin A & Daniel, Michael. 2016. “Medical error—the third leading cause of death in the US”. BMJ: i2139. BMJ. doi:10.1136/bmj.i2139.
[5] La Pietra, L. 2005. “Medical errors and clinical risk management: state of the art.”. Acta Otorhinolaryngol Ital. 25 (6): 339-346. PubMed. https://www.ncbi.nlm.nih.gov/pubmed/16749601.
[6] Roh, Hyunsuk Frank; Nam, Seung Hyuk & Kim, Jung Mogg. 2018. “Robot-assisted laparoscopic surgery versus conventional laparoscopic surgery in randomized controlled trials: A systematic review and meta-analysis”. PLOS ONE 13 (1): e0191628. Public Library of Science (PLoS). doi:10.1371/journal.pone.0191628.
[7] Mazzon, Giorgio et al. 2017. “Learning Curves for Robotic Surgery: a Review of the Recent Literature”. Current Urology Reports 18 (11). Springer Nature. doi:10.1007/s11934-017-0738-z.
[8] Huennekens, Scott. 2018. “Verb Surgical”. Twitter.com. https://twitter.com/verbsurgical/status/1008674056546242561.
[9] Vedula, S. Swaroop & Hager, Gregory D. 2017. “Surgical data science: the new knowledge domain”. Innovative Surgical Sciences 2 (3): 109-121. Walter de Gruyter GmbH. doi:10.1515/iss-2017-0004.
[10] Ezez. 2018. “Johnson and Johnson Q2 Analyst Presentation”. Edge.media-server.com. https://edge.media-server.com/m6/p/trmhmyqo.
[11] Webb, Marionn. 2018. “Market Intel: Rivals Catching Up To Intuitive Surgical In Fast-Growing, Fast-Innovating Robotic-Assisted Devices Market”. MedTech Insight | Pharma Intelligence. https://medtech-pharmaintelligence-informa-com.ezp-prod1.hul.harvard.edu/MT121751/Market-Intel-Rivals-Catching-Up-To-Intuitive-Surgical-In-FastGrowing-FastInnovating-RoboticAssisted.
[12] Udwadia, TehemtonE. 2015. “Robotic surgery is ready for prime time in India: Against the motion”. Journal of Minimal Access Surgery 11 (1): 5. Medknow. doi:10.4103/0972-9941.147655.
[13] Ho, Chuong Thanh. 2011. Robot-assisted surgery compared with open surgery and laparoscopic surgery. Ottawa, Ont.: Canadian Agency for Drugs and Technologies in Health = Agence canadienne des médicaments et des technologies de la santé.
[14] Rose, John et al. 2015. “Estimated need for surgery worldwide based on prevalence of diseases: a modelling strategy for the WHO Global Health Estimate”. The Lancet Global Health 3: S13-S20. Elsevier BV. doi:10.1016/s2214-109x(15)70087-2.
[15] Chandrasekaran, Brahadeesh. 2018. “Transformation Of The Medical Device Industry”. Frost And Sullivan. https://ww2.frost.com/frost-perspectives/transformation-medical-device-industry/https://ww2.frost.com/frost-perspectives/transformation-medical-device-industry/.
[16] Alkire, Blake C, Nakul P Raykar, Mark G Shrime, Thomas G Weiser, Stephen W Bickler, John A Rose, and Cameron T Nutt et al. 2015. “Global Access To Surgical Care: A Modelling Study”. The Lancet Global Health 3 (6): e316-e323. doi:10.1016/s2214-109x(15)70115-4.
I have no doubt that the paradigm of care (surgery 4.0), as described is attainable. However, my contention is that the rate of adoption of these technologies will be slower than most anticipate.
The adoption of advances that are beneficial to patients, can be stalled by surgeons when interests are not aligned. Figure 1 in the article references surgery 2.0 which included laparoscopic and minimally invasive surgery. However, these advances although beneficial for patients were resisted by the general surgery community because of their effect on reducing the learning barrier to performing some operations. You no longer needed a general surgeon to perform the procedure, radiologists and others became as capable of delivering the treatment (1). Surgery 4.0 attempts to reduce the learning barrier and I fear may suffer a similar reaction, if insufficient care is placed in its promotion.
In a world where access to laparoscopic struggle is still a challenge in developing countries (2), driven largely by equipment costs and a lack of personnel, I struggle to imagine mass impact from the developments in surgery 4.0. Although the pricing mechanism you describe improves the trialability of the technology, its long-term affordability is key for price-sensitive regions. Rather than delivering surgery to the 5.0 billion people that desperately need care, I would contend that it is likely to remain the preserve of innovative surgeons at leading academic centres for some time to come.
2030, is just over a decade from now – a brief flicker of time in the medical world, and in my view too short a time-frame to expect a dramatic change in care delivery without significant focus on addressing the major barriers to healthcare innovation.
1. Herzlinger RE. Why innovation in health care is so hard. Harv Bus Rev. 2006;84(5):58.
2. The role of laparoscopic surgery in developing countries: A review – SAGES Abstract Archives [Internet]. SAGES. [cited 2018 Nov 13]. Available from: https://www.sages.org/meetings/annual-meeting/abstracts-archive/the-role-of-laparoscopic-surgery-in-developing-countries-a-review/
Ollie, great insight and thanks for your comment.
I would argue that standardization, and low barriers to trailability and adoption associated with collaborative autonomy, coupled with an attractive pricing model, affords an opportunity for surgery 1.0 countries to leapfrog to surgery 4.0. To elaborate more on the pricing model, one can evision a scenario where free upfront costs, coupled with discounted subscription fees for emerging markets, are offset by other markets where the willingness to pay is higher.
On your point about barriers to healthcare innovation, I would like to clarify what exactly collaborative autonomy means to me in 2030 – it’s not necessarily robots performing tasks autonomously, but also “guard-railing” surgeons from deviating from the norm (i.e. damaging critical anatomy, by enforcing virtual force constraints). The latter scenario seems a lot more tenable in a decade in my mind, and the FDA and MIRS companies have been receptive to this mission (in the R&D sphere) – at least through the last decade.
This is a very well written vision and I love the clear infographics. You note that your two biggest challenges for implementation are data and annotation. However, I believe the biggest challenges you will face in adoption will be outcomes evidence and time savings. Right now, having witnessed robotic colorectal surgery, there is still significant negative opinion among physicians and surgeon regarding whether surgical robotics are worth the investment. Right now, many have expressed the sentiment that it mainly has built PR for hospitals to attract high paying customers, which is a concern that will need to be addressed. Talking to them, they have said they do not believe that patients have better outcomes with robotic surgeries when compared to manual surgeries, and the cumulative additional setup time causes the surgeries to take more time and reduce the ability of surgeons to see as many patients. These are are not insurmountable problems, but I would recommend highly prioritizing innovation effort to directly respond to them as much as possible.
Best of luck!
I really enjoyed reading this article. I remember watching the movie Prometheus a number of years ago and wondering why humanity couldn’t build something like their artificially intelligent surgical robotics system, where the system rapidly diagnosed and performed surgical intervention. My biggest question is simply technical efficacy, and my guess is that this will take a long time to resolve. I think you are quite right that data and practice is a good way to ‘feed’ this system, I just wonder if you could get close enough to the ‘real thing’ to have the learning apply. I do like your proposals for no downside risk to the hospital system that is trying the device. Great read!