Using Machine Learning for Crime Prediction
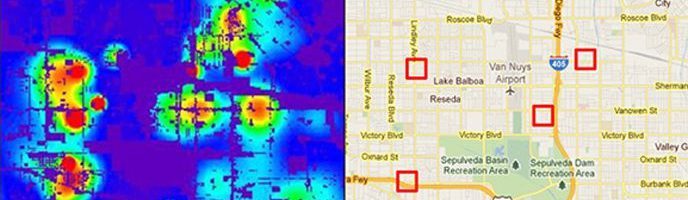
Police departments are increasingly using predictive algorithms to determine "hot spot" potential crime areas.
Machine learning is transforming the way that governments prevent, detect, and address crime. Around the country, police departments are increasingly relying on software like the Santa Cruz-based PredPol, which uses a machine learning algorithm to predict “hot spot” crime neighborhoods – before the crimes occur. Police forces can use the software to plan officer patrol routes, down to half-city blocks.[i] PredPol does not publish a complete list of the cities it serves, but is currently in operation in over 60 cities in America, including Atlanta and Los Angeles.
PredPol utilizes commonly-understood patterns for when, where, and how crimes occur, and formalizes those patterns using an algorithm that predicts locations where a crime is likely to occur in the near future. Studies show that that crime tends to be geographically concentrated, but that these “hot spots” are often dispersed throughout a city.[ii] Using a machine learning model originally built to predict earthquakes, PredPol uses location, timing, and type of crime as inputs (see below for an example output).[iii]
PredPol Operator view tile[iv]
PredPol offers a police department the ability to glean insight from enormous amounts of data quickly and cheaply (police departments pay between $10,000 and $150,000 for access to PredPol’s software).[v] When police forces have limited resources, a tool that can quickly make data-informed recommendations about how to allocate those resources represents a step-change in a department’s efficiency. In 2012, the Police Executive Research Forum surveyed over 200 police departments and reported that 70% intended to increase its use within the next five years to predict the location of “fragile” neighborhoods.[vi]
Over the next few years, PredPol plans to expand to new cities. On October 25, 2018, PredPol announced a partnership with Caliber, an online platform used for public safety and jail management.[vii] As PredPol usage increases, the predictive power of the algorithm should continue to improve.
Planning police routes is far from the only application for machine learning in criminology. PredPol’s algorithm currently focuses on violent crime, but with small tweaks could be used to predict white collar crimes like mortgage fraud. PredPol could also use its technology to analyze police body camera footage, determining which behaviors predict positive or negative encounters with citizens. Adding crowd-sourced footage of citizen-police could also help police departments improve and create a powerful tool for citizen engagement.
Or, with only slightly different inputs, the technology could predict the neighborhoods where social services are most needed. What if PredPol were used to pinpoint city blocks that were vulnerable to potential violence, and then utilized to provide targeted social services? Predicting crime is not the same as preventing it, and fully integrating this technology could fundamentally change how cities deliver end-to-end services.
However, relying on predictive algorithms to identify potential offenders raises concerning ethical questions. Although ProdPol insists that it only uses time, location, and type of crime as predictors, similar predictive software (e.g., tools used to predict recidivism risk)have been found to have significant racial bias[viii]. Even without race as an explicit input, it is not difficult to imagine that a system that over-samples black neighborhoods will continue to predict crime in those neighborhoods. In December 2017, New York City Council became the first in the country to pass an “algorithmic accountability bill,” which established a task force to study how agencies use algorithms and whether they have encoded biases.[ix] Given the growing demand for predictive algorithms, platforms like PredPol will become cheaper and more readily available in the future. As reliance increases, how should agencies think about implementing increasingly “smart” technologies, while themselves understanding when the platforms are doing more harm than good?
(Word count: 799)
[i] Ellen Huet. “Server and Protect. Predictive Policing Firm PredPol Promises to Map Crime Before It Happens.” Forbes, March 2, 2015. https://www.forbes.com/sites/ellenhuet/2015/02/11/predpol-predictive-policing/#6a3d89084f9b. Accessed November 12, 2018.
[ii] Weisburd, D. (2018), Hot Spots of Crime and Place‐Based Prevention. Criminology & Public Policy, 17: 5-25. https://onlinelibrary.wiley.com/action/showCitFormats?doi=10.1111%2F1745-9133.12350 Accessed November 12, 2018.
[iii] Hardyns, W. & Rummens, A. European Journal on Criminal Policy Research (2018) 24: 210. https://link.springer.com/article/10.1007%2Fs10610-017-9361-2 – citeas Accessed November 12, 2018.
[iv] Hardyns, W. & Rummens, A. European Journal on Criminal Policy Research (2018) 24: 209. https://link.springer.com/article/10.1007%2Fs10610-017-9361-2 – citeas Accessed November 12, 2018
[v] Ellen Huet. “Server and Protect. Predictive Policing Firm PredPol Promises to Map Crime Before It Happens.” Forbes, March 2, 2015. https://www.forbes.com/sites/ellenhuet/2015/02/11/predpol-predictive-policing/#6a3d89084f9b. Accessed November 12, 2018.
[vi] Police Executive Research Forum. 2014. Future Trends in Policing. Washington, D.C.: Office of Community Oriented Policing Services. https://www.policeforum.org/assets/docs/Free_Online_Documents/Leadership/future%20trends%20in%20policing%202014.pdf Accessed November 12, 2018.
[vii] Business Insider. “Caliber Public Safety is Excited to Announce its Partnership with PredPol, Inc. And to Be Able to Offer a Predictive Policing Solution to Its Clients. https://markets.businessinsider.com/news/stocks/caliber-public-safety-is-excited-to-announce-its-partnership-with-predpol-inc-and-to-be-able-to-offer-a-predictive-policing-solution-to-its-clients-1027656394 Accessed November 12, 2018.
[viii] ProPublica. “Machine Bias.” May 23, 2016. https://www.propublica.org/article/machine-bias-risk-assessments-in-criminal-sentencing. Accessed November 12, 2018.
[ix] Lauren Kirchner. “New York City Moves to Create Accountability for Algorithms.” ProPublica, December 18, 2017. https://www.propublica.org/article/new-york-city-moves-to-create-accountability-for-algorithms Accessed November 12, 2018.
This was super interesting to read, particularly surrounding the debate around the ethical implications and algorithmic accountability of a tool like PredPol, and how to prevent biases and racial discrimination. I wonder also whether police officers might use Predpol to fall victim to confirmation biases, or even use it to justify any “false negatives” in the data that lead to police brutality or false arrests. As described, it could also help correct for predetermined biases and errors in human judgement based on the proliferation of data that Predpol uses, but ultimately we know that the results of even the most advanced machine learning models are contingent on human decisions made at the end.
This essay rules.
PredPol clearly has significant benefits for police departments that are constantly facing budgetary constrains and resource limitations. It seems like it could help any police force in a big city to plan their routes more effectively. I’m wondering, though, how applicable it is for smaller cities with even less funding. Take Flint, MI, for example. I imagine the department has a pretty good idea of where and when crimes are most frequent, but still struggle to stay on top of it because they are stretched too thin.
As you say, the ethical questions associated with this technology are serious — racial bias especially. New York City’s legislation seems like a great first step. Two thoughts come to mind. First, how much bias and injustice would cities be willing to tolerate from an AI algorithim if the overall impact would produce a more just police presence than exists today in its absence? Second, how might the right to privacy be used to challenge the legality of this tool or limit its dataset?
Good questions Jack, jumping in here just for fun. In terms of biases, I would tolerate zero biases. For example, as soon as the system is establishing that the main driver for a certain threat is the person is from a certain race, I’d stop it immediately. ML systems can release that information if asked to do so. Regarding privacy, I think security comes first. As long as the data is properly handled I’d be happy to lose “a very big portion” of my privacy if it meant that my risk of being robbed or attacked is reduced significantly. But I think it’s a very personal decision and understand that some people would prefer to be less safe and keep their privacy.
Very interesting technology! Providing law enforcement and social services with such substantial data points about different locations could be a valuable tool, especially when making resource allocation decisions. These organizations seem to be constantly stretched and this could prove to be extremely helpful in deciding where individuals could do the most good. In terms of the inherent biases, I definitely agree that this is a very real issue that must be addressed. Could it be possible to give that task force you mentioned some enforcement authority? Potentially allowing them to research agencies and if need be require them make changes to personnel to ensure fair representation.
Really interesting essay. This example of Machine Learning showcases both the immense potential that this technology has to make our society better and the tremendous risks that are come along with it, especially on the biases side.
Regarding your question on how governments should deal with this technology I think that Silicon Valley is kind of trying to reinvent the wheel here: humans have been debating about ethical problems involving new technologies for centuries. I think that once the problem and its risks become evident (and luckily they seem to be very clear here), the solution is to involve policy makers, social scientists, cientists, groups that represent the affected groups and build standards of engagement that guarantee transparency. What NY has done seems like a good first step!
I think that this technology can be tremendously impactful if deployed the right way, and agree with the many concerned mentioned around racial (and other biases). One question i’d like to see PredPol tackle with its partner cities is how to increase data and remove bias in data collection. If the data gathered is all based on police officer observations and crimes reported by civilians, there may be a whole host of crimes that are committed and never reported. By concentrating police efforts in areas with frequent crime reports, the algorithm may bias simply towards areas where residents are more likely to report. To combat this challenge, I would like to see some technology deployed in concert with PredPol, such as ShotSpotter, (https://www.shotspotter.com/), which has been deployed in Chicago to aid in their police analytics efforts.
I greatly appreciate the application of ML to policing and helping departments “police smarter” (particularly within the context of budget constrained departments and personnel constrained shifts); however, thinking about how to implement these technologies, I think it’s extremely important to think about what they rely on: quality inputs. It’s unclear exactly what PredPol is using form a data standpoint, but, outside of using data such as historical incident reports (likely racially and geographically biased), I think it’s important to scrutinize what the algorithms are causally associating with crime. What are these correlations and do they pass the smell test with humans? The reason for my skepticism is if PredPol is simply a data aggregator or actually capable of producing the incredible societal benefits of predictive analytics and, if so, how?
Additionally, while I believe it’s smart to outsource functions such as routing to technology providers (with or without machine learning), I think it’s dangerous for departments to over-index to such solutions, at the expense of police engaging in traditional activities as part of the community and “walking the beat” from time-to-time. Communities are dynamic and it’s unclear what ML judgement edge might have on human judgement as things change and evolve with time. I could be totally wrong, but I worry about “outsourcing” too much to technology providers and having the result be a disengaged police force with a psychology that believes a machine knows what’s best. This shift is psychology is most worrisome.
Not sure if it’s a current input, but one really interesting input I might consider is the input of individuals from the community to both help paint the picture of context in the community as well as simply crowd-sourcing what is actually happening at any given point in time? The combination of human inputs + ML technology seems particularly dangerous and empowering for our public servants. I also think crowd-sourcing conditions on the ground might help not only predict criminal incidents, but also help prevent them by making the likes of PredPol a tool for everyone – not just the police.
The potential application for “PredPol”, both for police forces and for alternate uses, is fascinating. I love the idea of using the predictive software not only to anticipate crime locations, but to deploy preventive resources such as social services. These alternate uses can strengthen communities and build trust in the software in communities.
I am curious about the long-term effects of policing areas predicted by the application. While its current inputs may accurately guide police forces to crime “hot spots”, criminals have proven to be resourceful in their evasion of the law. I would be interested to see how these “hot spots” develop over time. Are police continuously sent to similar locations to keep crime down, or does crime continue to move around cities? Can this application actually prove that it is making cities safer?
Great article and something I’ve always been very interested. As other comments mention – these types of machine learning initiatives have a huge impact on city governments, especially from a budgetary perspective. In that sense – I think they can really help make city governments more efficient.
To your questions though – I am very concerned about how these get implements. First, data in produces that data that comes out and I worry that the data going into these models is biased in some fashion. Second, there has been a huge push recently for community policing where officers are more connected to their communities. I worry that machine learning and “policing through data” removes the human element of crime prevention and can give police departments an excuse to further remove themselves from the communities they serve.
This is a very interesting application of machine learning. One very interesting way of applying this might to focus social policies on these neighborhoods. By focussing in improving some variables in these areas you will see the effect in a few years. For example by investing in education or creating recreational centers in these areas you could decrease crime in the following years. Also, according to the broken window theory you could work on cleaning more often the neighborhood and making investments in light posts, and fixing the broken windows to generate a better atmosphere and reduce the risk for crime.
Given the concerns around biased data that others have mentioned, I would echo Emilio’s point and add that in addition to the “time, location, and type of crime as predictors”, there may be room to introduce more predictive race-agnostic variables into the algorithm, such as the number of broken windows in particular parts of cities (although I admit there may be costs associated with collecting such data if it is not available in public domain ex ante).
In addition, one area I was unsure of was to what extent police would be the sole users of PredPol. It seems like Law Enforcement and Corporations are the main target customers (see http://www.predpol.com/corporate-security/) but I wonder if, e.g., parents who are keen to ensure their children are walking safe routes to and back from school could also use Predpol to suggest to their kids routes to take or avoid? Is there a case to be made for B2C applications?
Really enjoyed reading this! Fascinating topic. Weighing in on the bias discussion, I think one way to mitigate the bias of over-sampling certain neighborhoods (e.g. those with a large percentage of minority or low income residents) is to require that all criminal incidents within a given police district should be included to generate and continuously update the machine learning algorithm. In my view, correlation between the algorithm outputs and demographic attributes does not imply bias – provided that the inputs to the algorithm are non-discriminatory. These inputs need to be strictly regulated.
Another question on my mind is: what happens when humans adapt to this algorithm and changes their criminal behavior patterns to “game the system”? For example, if offenders notice that police cars are patrolling certain blocks in traditionally “bad” neighborhoods more frequently, it’s possible that they will re-locate their activities to less-patrolled blocks in traditionally “nice” neighborhoods – which could generate significant backlash from the residents of those places.
Echo WJB’s comment on the idea of making this tool available to the individuals, not just the police. It is certainly very interesting application of machine learning and trying to solve a very relevant problem in today’ society, but its impact would be much higher if applied to emerging countries where violence and crime levels are much higher. Think of Latin America, where not only crime levels are high but corruption may also affect the effectiveness of local police forces enforcing the law. Empowering individuals to prevent their own exposure to crime and violence seems an interesting alternative in these countries.
Very interesting topic indeed and in my mind these are the “big problems” that come to mind when thinking about how humans should use artificial intelligence and machine learning. I agree that this could result in a huge efficiency as in many countries not just the US, police budgets have been under pressure. Also agree on the ethical issues that you raised – in my view the bigger question is who owns the data? Is it the software company or governments? Owning this type of data can potentially have serious negative consequences. Also found it interesting that this technology can be used in predicting needs for social services – would love to hear more about it!
I wonder what the sources of data for PredPol are. PredPol’s algorithm requires a large number of high-quality data to be accurate and if a lot of the data are from public security cameras, then I wonder if there will a push to have more of these cameras to collect more data. And will more security cameras and more data collected by PredPol infringe on more people’s privacy and cause concerns? Also, in order to scale up quickly, PredPol’s algorithm needs to be able to integrate with the current police department IT system easily. I am curious if there is any challenge related to such integrations.
Thanks so much for your thought-provoking article! I’m really glad that PredPol exists and I’m glad that it’s helping police forces around the US. From a machine learning perspective, the predictive analytics does help police force allocation, but I do worry that criminals understand this and target areas which are typically less prone to crime. Here lies a problem commonly faced with predicitve analytics in that in some cases, it becomes easier to game the system. I worry about how this technology assesses for outliers and I hope that more crime does not exist due to this technology.
I think that it is okay if the systems pick up neighborhoods with similar race as predictive systems aren’t implying causality. For me, it is more important to have a system which can pick up likely areas with crime than it is to sort for race issues. If there is more work done to educate the police force on racial bias, I believe that the predictive analytics systems will improve in step.