An A.I. Odyssey: How Fox is Using Machine Learning to Market Movies
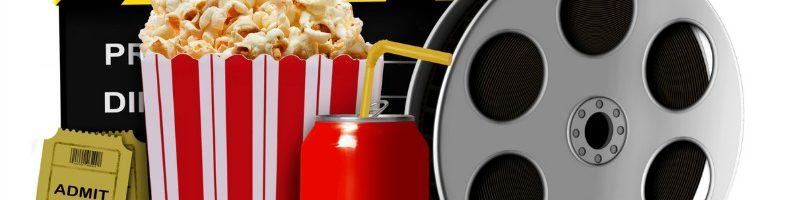
Movie studios have historically relied on experience to predict movie budgets and their allocations. In recent years, they have begun leveraging machine learning to improve marketing efficiency. Fox has been at the forefront of these efforts through its collaboration with Google Cloud. While studios have made great progress in using machine learning to better manage their marketing, there is still a lot of white space for technology to help inform the production process. However, movie-makers have to be careful not to let data crush creativity.
Why the Movie Industry is Ripe for Machine Learning Disruption
- Wide range of returns highlights difficulty of getting it right
Stephen Follows analyzed 29 blockbusters with an average production budget of $150mm and their average profit was only ~$14mm. In fact, an oft-cited rule of thumb is that ~50% of movies make money while ~50% lose money. In a follow-up, Stephen demonstrated that half the money earned from profitable ventures in recent times came from the top 6% of movies and half the money lost came from the bottom 6%. [1] [2] New technologies can help studios both move the profitability curve upwards and shed the right tail seen in Figure 4.
Figure 1. Illustrative Movie Studio’s Revenue Streams
Figure 2. Average Blockbuster Revenue Breakdown Figure 3. Average Blockbuster Cost Breakdown
Figure 4. Profit Dispersion for 279 Movies
- Downside risk in dollars increasing as costs are increasing
Movies are increasingly expensive as studios have been concentrating on big bets. According to Eric Buchman, “Hollywood is no longer in the business of hitting singles. They now swing for the fences almost every time they step to the plate […]” After adjusting for inflation, 45 of the 50 most expensive movies ever made were released in the last decade or so [3]. By concentrating their bets, studios increase the chances of large returns in dollar terms but also increase the chances of large losses, so they seek every advantage to minimize downside risk.
Figure 5. # Movies Released per Year Costing $100mm+
How Fox Leverages Machine Learning to Inform Decisions
Fox has been at the forefront of using machine learning to match stories and audiences. Historically, studios employed a “spray and pray” approach: show many ads and hope for the best [4]. Fox’s A.I. project began when the studio realized it was promoting “The Greatest Showman” to the wrong audience; instead of appealing to musical lovers, it was a hit with those interested in characters shunned by society [5]. Since then, Fox has partnered with Google Cloud to develop a learning program, called Merlin, that tries to identify the basic patterns in audiences’ preferences for different types of movies through trailer analysis [6]. Initially, the team loaded Merlin with customer data, film synopses and trailers to build its pattern recognition abilities.
Figure 6. Merlin Architecture Flow Diagram
The first step in a new analysis is for Merlin to tag all the elements in a trailer.
Figure 7. Merlin Tagged Labels for Logan’s Trailer
Then, Merlin feeds the information it has into a very complex custom neural network that learns over time and produces a current movie attendance probability for the audiences of prior movies.
Figure 8. Merlin Prediction Model Process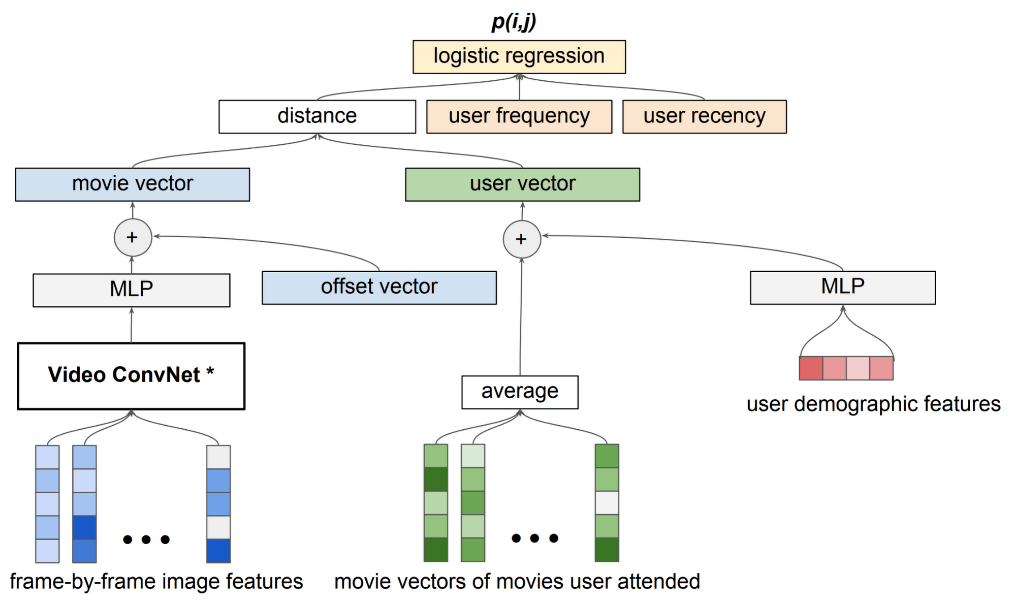
The result is a prediction of audiences from prior movies that are most likely to be interested in the current movie, i.e. target audiences. On a weekly basis, the team gets a scorecard that compares Merlin’s predictions to actual results so it can close the loop and have Merlin improve over time.
Figure 9. Merlin Accuracy Scorecards
Potential Next Steps
Currently, Fox seeks to integrate Merlin Video with Merlin Text, a script analyzer, into one framework that leverages both methods to produce better predictions. Fox will likely also be focusing on building out tools that allow it to reach those audiences through targeted advertising, a complex task as explained by Matt Marolda of Legendary Entertainment [7]. On the production side, while Merlin may help provide some broad thematic insights, there is still a lot of white space to use machine learning to help make decisions, such as with casting choices. Another exciting avenue is the use of facial biometrics by Disney to track & predict audience reactions on a scene-by-scene basis, helping film-makers understand in advance the impact a scene may have on their target audiences [8]. Such technologies can help Fox grow Merlin from a marketing optimizer to an analytics platform that provides significant value throughout the entire lifecycle of a production.
Key Considerations
One of the key issues for Fox is the balance between data-driven decision-making and creativity. By copying what has worked well in the past, it can kill innovation by only iterating on existing ideas instead of taking risks on new ones. For example, Avatar altered the movie landscape in 2009 through its use of 3D technology, but a machine learning algorithm would likely have struggled to predict its success. How can movie studios best combine technology & creativity to produce movies that are not only profitable, but also define and inspire generations?
(780 words)
References:
[1] Stephen Follows. How movies make money: $100m+ Hollywood blockbusters. Stephen Follows: Film Data & Education (July 10, 2016).
[2] Stephen Follows. Do Hollywood movies make a profit? Stephen Follows: Film Data & Education (July 25, 2016).
[3] Eric Buchman. Why are movies more expensive than ever when tech makes them easier to make? Digital Trends (October 12, 2014).
[4] Michael Krigsman. Moneyball for movies: Data science and AI in Hollywood ZDNet (February 20, 2018).
[5] Brent Lang. How Mobile Phones Are Giving Hollywood a Better Understanding of Its Audiences Variety (March 22, 2018).
[6] Miguel Campo-Rembado & Sona Oakley. How 20th Century Fox uses ML to predict a movie audience Google Cloud (October 29, 2018).
[7] Matthew Marolda & Michael Krigsman. “Moneyball” for Movies: Data and Analytics at Legendary Entertainment CXO Talk (February 9, 2018).
[8] Disney Research. Neural nets model audience reactions to movies Phys.org (July 21, 2017).
I think your question around data-driven decision making vs. creativity is critical for the entertainment industry, but I don’t think they necessarily have to be at odds. As studios’ machine learning capabilities continue to increase, I think they’ll be able to identify the high-level factors that make a movie successful (e.g. story line construction, types of actors/actresses, etc.) while still leaving plenty of room for creativity in the scripting and production processes.
As you mentioned above, there’s such a massive range of financial outcomes for movies today, and I think machine learning can help studios avoid negative returns while still leaving room for the creation of hugely innovative movies like Avatar.
Very interesting article! One thought that immediately came to mind was if machine learning may be able to play a larger role in the future in the script-writing process. You mentioned the script analyzer that exists, and it seems like there may be some promise at the very top of the funnel at the conception and script-writing phase, instead of only focusing this technology on the marketing phase.
Thanks to Mr. Gauthier for sharing some great thoughts on this subject! In contrast to certain studios taking massive swings with a fewer number of films (a la the Marvel canon), I am curious to see how production companies with a different model can utilize machine learning.
For example, Jason Blum at Blumhouse Productions supports a larger number of films that are less expensive to create. By doing things like focusing on scripts with minimal set changes and compensating big time actors with more variable compensation, Blumhouse limits the downside risk on each film while pursuing massive upside (e.g. “Paranormal Activity”). This ends up creating a portfolio of many small bets that looks more like that of a VC shop than a typical studio or buyout shop.
It would be really interesting to see if machine learning can help Blumhouse refine its understanding even further of what makes a “successful movie.” Perhaps machine learning can help Blumhouse process a massive number of scripts at the ‘top of the funnel’ so that the company can see an even wider set of investment opportunities. This might allow scripts to hit the final screen that might not have otherwise made it if the screenwriter couldn’t get the right meeting.
Thanks JP, great article! It is interesting to the process of how Merlin feeds the data, runs analysis and predicts target audience. This approach of machine learning reminds me of how online reading apps news feed, where algorithm pushes articles to readers based on their past preferences and viewing time. However, film production is indeed turning into almost making bets these days. With such big investments, it makes perfect sense to have a more accurate understanding of how return is going to look like. On the other hand, I would be interested in learning: similar to reading app news feed, would producing and recommending films largely based on audience preference limit their exposure to other genres, among which the audience might be interested in? Does it hurt the chances of the less mainstream movie production?