How machine learning will disrupt mineral exploration
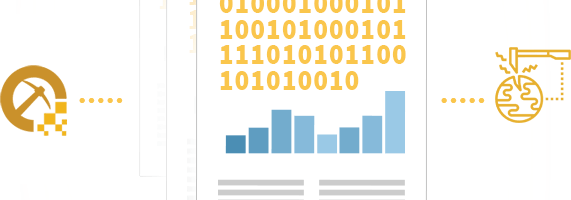
Goldspot Discovery Inc. is revolutionizing the mining industry by exploring and discovering mineral deposits with the aid of machine learning techniques.
Context
The mining industry is dedicated in the extraction of mineral resources formed by natural occurrences in the earth’s crust. Finding a mineral deposit however, is the first step of any mining business and is a task that has become extremely challenging over time. Most shallow and exposed to the surface deposits have been mined for centuries by humans, and many of these are today reaching depletion. Finding a new, rich mineral deposit requires a multidisciplinary team of geoscientists analyzing and interpreting multiple layers of information of a geographical location in order to find deeper, covered mines. Digitalization of long dating geospatial databases and increased computing power is allowing machine learning techniques to assist geoscientist in the quest for the next mineral discoveries [1].
The Approach
Goldspot Discovery’s business model involves partnering with resource extraction companies to access their proprietary geological databases, which are used to train and refine machine learning algorithms to predict the location of mineral occurrences. By collaborating with a large amount of mining companies, national geological services and research institutions, Goldspot can combine multiple layers of geological, geochemical, geophysical and other geospatial information and feed their algorithms to identify patterns and relationships underlying the formation of deposits, which then enable the “machine” to predict the location of new mines. The algorithms train by “mining” the various databases to try locating existing mines; Goldspot was able to identify 86% of the gold formations in the Quebec Atibiti region, using only 4% of the total regional area [2].
Although still far from general adoption by established miners, machine learning has the potential to drastically change the decreasing trend in mineral deposit discoveries. In the case of gold, the mining industry spends several billions every year in global exploration, reaching a peak of six billion dollars in 2012. However, the amount of new gold mines discovered has plummeted from nearly 20 a year to only 1 or 2 per annum over the last five years. Factors driving the decline include increased depth, extensive cover material and more remote locations in new mineral occurrences, which make the task more complex and expensive for traditional geologists. Machine learning techniques are used to find anomalies [3] among wide datasets that today human scientists are not able to see on their own. Goldspot is bullish that their proprietary machine learning technology will unlock many new unfound resources, and general adoption of this approach by the natural resources industry is a matter of just a few years.
Going Forward
In the short term, Goldspot is dedicated to increase its data bank by working with more clients and partnering with more research institutions, as algorithms need to be further trained and tested before they are able to make predictions of acceptable levels of confidence. In order to attract more third parties to provide access to their own geological databases, Goldspot offers and array of services to their customers, including the digitalization of old geospatial records (e.g. old documents and reports, scanned map images, excel spreadsheets, etc) into state of the art formats for database management. One thing that Goldspot could do to complement this type of services and boost data acquisition is using machine learning techniques also to interpret the images in these documents and translate them into digital records to build workable databases. For some of the largest mining companies who have operated in five continents for more than a hundred years, there is significant value added in “reviving” their vast legacy archives lying somewhere at the bottom of their headquarter offices.
In the medium term, Goldspot will move from a purely services offering business into investing. As their algorithms provide predictions with higher levels of confidence, Goldspot aims to start making equity invests in their partner companies to fund drilling of specific targets generated by their machine learning algorithms. However, investing in resource discovery and development is inherently risky and requires having a strong balance sheet to endure volatility shocks. Goldspot should consider as part of their strategy using other non-dilutive structures to retain upside, such as royalty agreements on any discovery made by drilling targets generated by their algorithms.
Open Question
One of the key limitations of Goldspot’s approach is the fact that acquisition of geospatial data still has to be carried out by traditional means. Sampling rock chips or measuring the magnetic response of the earth requires conducting exploration campaigns on the field, which in many cases is an expensive investment. Upon depletion of shallower mines, finding deeper and more remote deposits may require acquiring and analyzing new sets of data. How can Goldspot keep hunting for gold once they reach the boundaries of available out of data to mined?
(772 words)
[1] Desharnais, Guy . “How machine learning will disrupt mining” CIM Magazine, February 15, 2018 | http://magazine.cim.org/en/voices/how-machine-learning-will-disrupt-mining-en/
[2] Goldspot Discovery Inc. | https://goldspot.ca/about-us/
[3]