Regulatory agencies like the Occupational Safety and Health Administration (OSHA) and the state agencies it oversees have significant responsibilities in ensuring safe working conditions in millions of workplaces nationwide. However, these agencies face significant challenges due to limited resources, and inspect less than 1% of workplaces annually. In their article, “Making Workplaces Safer Through Machine Learning,” Matthew S. Johnson, Assistant Professor of Public Policy and Economics at Duke University’s Sanford School of Public Policy; David I. Levine, Eugene E. and Catherine M. Trefethen Chair in Business Administration at University of California Berkeley’s Haas School of Business; and Michael W. Toffel, Senator John Heinz Professor of Environmental Management at Harvard Business School and Principal Investigator in D^3’s Climate and Sustainability Impact Lab, propose that machine learning can help these agencies make the most of limited resources and increase the effectiveness of inspections.
Key Insight: The Impact of Workplace Inspections
“Our study examined tens of thousands of workplaces across 28 states and found that OSHA inspections reduced injuries by 9 percent, broadening the implications of our earlier study […] that found California workplaces experienced a 9.4 percent decline in injury rates.” [1]
Inspections have proven to be a powerful tool in reducing workplace injuries, with quantifiable results. Large studies in many states indicate a consistent reduction in injury rates post-inspection.
Key Insight: Machine Learning Doubles Potential Gains
“OSHA could prevent as much as twice as many injuries – translating to up to 16,000 fewer workers injured and nearly $1 billion in social benefits over the decade.” [2]
Traditional inspection strategies prioritize workplaces with historically high injury rates, but these may not align with current risk profiles. Working within existing budgets, machine learning prediction algorithms can offer a more dynamic approach, forecasting where inspections will yield the most significant reductions in injuries by targeting workplaces predicted to be most responsive to inspections and those with the highest current predicted injury rates, rather than focusing only on workplaces with a previous history of injuries.
Key Insight: Pros and Cons of Smarter Tools
“[G]ains must be balanced against concerns about transparency and fairness that can arise given the ‘black box’ nature common to many machine learning prediction algorithms.” [3]
The potential for enhanced effectiveness extends beyond OSHA and workplace safety. Recent research highlights how machine learning can boost the efficiency of inspections in diverse fields, including restaurant hygiene and water pollution. However, while machine learning offers immense potential, its use presents challenges, for example, the opaque nature of some algorithms can lead to concerns about bias or unfair targeting by regulatory bodies.
Why This Matters
For policy makers, the insights from this research present a dual opportunity: enhancing workplace safety and managing the cost of inspections. Given inspection agencies’ limited budgets, policy makers should strongly consider adopting machine learning as a tool to enhance regulatory effectiveness, particularly in the realm of workplace safety. This approach, if implemented responsibly and transparently, has the potential to significantly improve worker well-being and optimize the allocation of limited regulatory resources. These advantages might also apply beyond OSHA workplace inspections, to other areas where safety is paramount, such as food safety and environmental monitoring inspections.
References
[1] Johnson, Matthew S., David I. Levine, and Michael W. Toffel, “Making Workplaces Safer Through Machine Learning”, The Regulatory Review (February 26, 2024). https://www.theregreview.org/2024/02/26/johnson-levine-toffel-making-workplaces-safer-through-machine-learning/
[2] Johnson, Levine, and Toffel, “Making Workplaces Safer Through Machine Learning”. https://www.theregreview.org/2024/02/26/johnson-levine-toffel-making-workplaces-safer-through-machine-learning/
[3] Johnson, Levine, and Toffel, “Making Workplaces Safer Through Machine Learning”. https://www.theregreview.org/2024/02/26/johnson-levine-toffel-making-workplaces-safer-through-machine-learning/
Meet the Authors
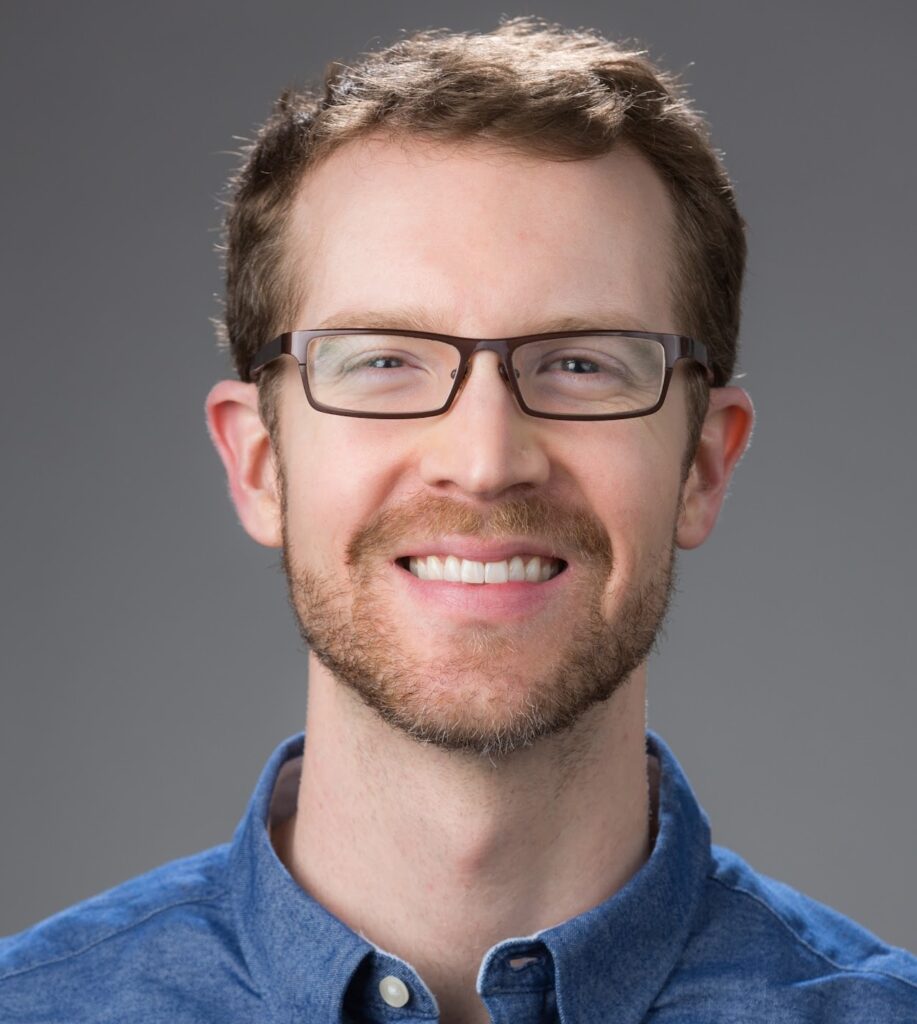
Matthew S. Johnson is an Assistant Professor of Public Policy and Economics at Duke University, with a primary appointment at the Sanford School of Public Policy and a secondary appointment in the Department of Economics. He is also a Faculty Research Fellow at the National Bureau of Economic Research.
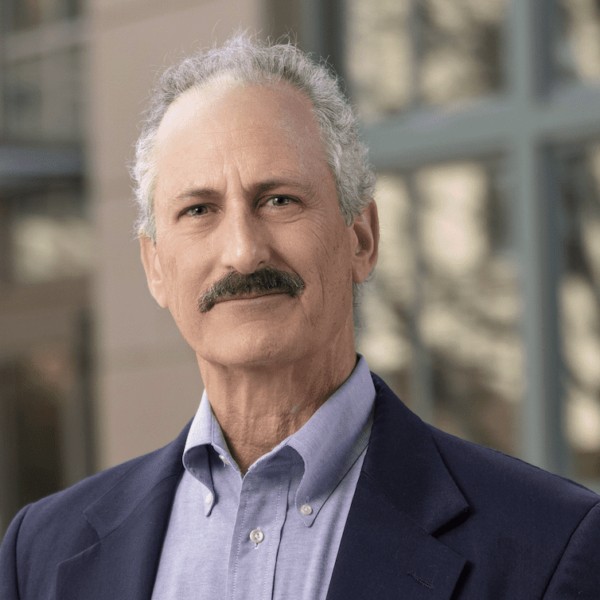
David I. Levine is the Eugene E. and Catherine M. Trefethen Chair in Business Administration at University of California – Berkeley’s Haas School of Business. Levine’s research focuses on understanding and overcoming barriers to improving health in poor nations. This research has examined both how to increase demand for health-promoting goods such as safer cookstoves and water filters, and how to change health-related behaviors such as handwashing with soap.

Michael W. Toffel is the Senator John Heinz Professor of Environmental Management. His research examines how companies are addressing climate change (especially decarbonization) and other environmental and working condition issues in their operations and supply chains. He co-founded and hosts the HBS Climate Rising podcast, which covers a range of business and climate change topics, and co-created the HBS Working Conditions in Supply Chains microsite to summarize evidence-based academic research on that topic.