This series, produced by Harvard’s D^3 Institute in collaboration with the Responsible AI Institute, gathers experts in the fields of responsible AI implementation and healthcare to explore how executives are transforming healthcare organizations through Generative AI. The content below includes articles, webinar recordings, and exclusive interviews in which we discuss key insights on operationalizing GenAI while minimizing risks, as well as the different guardrails to consider across various healthcare sectors.
Session 1: Realizing Healthcare’s AI Potential
The following insights are derived from a recent session on GenAI in Healthcare.
On November 15th, the D^3 Institute’s Generative AI Observatory hosted the first session in its GenAI in Healthcare series, produced in collaboration with the Responsible AI Institute. Speakers Satish Tadikonda (HBS), Nikhil Bhojwani (Recon Strategy), Var Shankar (Responsible AI Institute), and Alyssa Lefaivre Skopac (Responsible AI Institute) discussed key insights on operationalizing GenAI while minimizing risks, as well as the different guardrails to consider across various healthcare sectors. Below are some of the session’s key takeaways, which will be explored further in subsequent sessions.
Many Stages of AI Adoption in Healthcare
The healthcare industry already contains many AI use cases, and GenAI represents the next stage of potential adoption. There are multiple examples where such tools are already being used to measure and evaluate, aid in decision-making, or execute treatment plans. However, organizations are currently at different levels of maturity in how they approach AI implementation, with many unsure of how best to approach this type of change. A sample poll of our audience showed that 37% were just starting out on their AI journey, and 30% felt they were headed in the right direction but not in the realm of industry leaders. With the exception of 4% who felt they were in that frontrunner category, the rest expressed uncertainty with how their organization would undertake AI adoption.
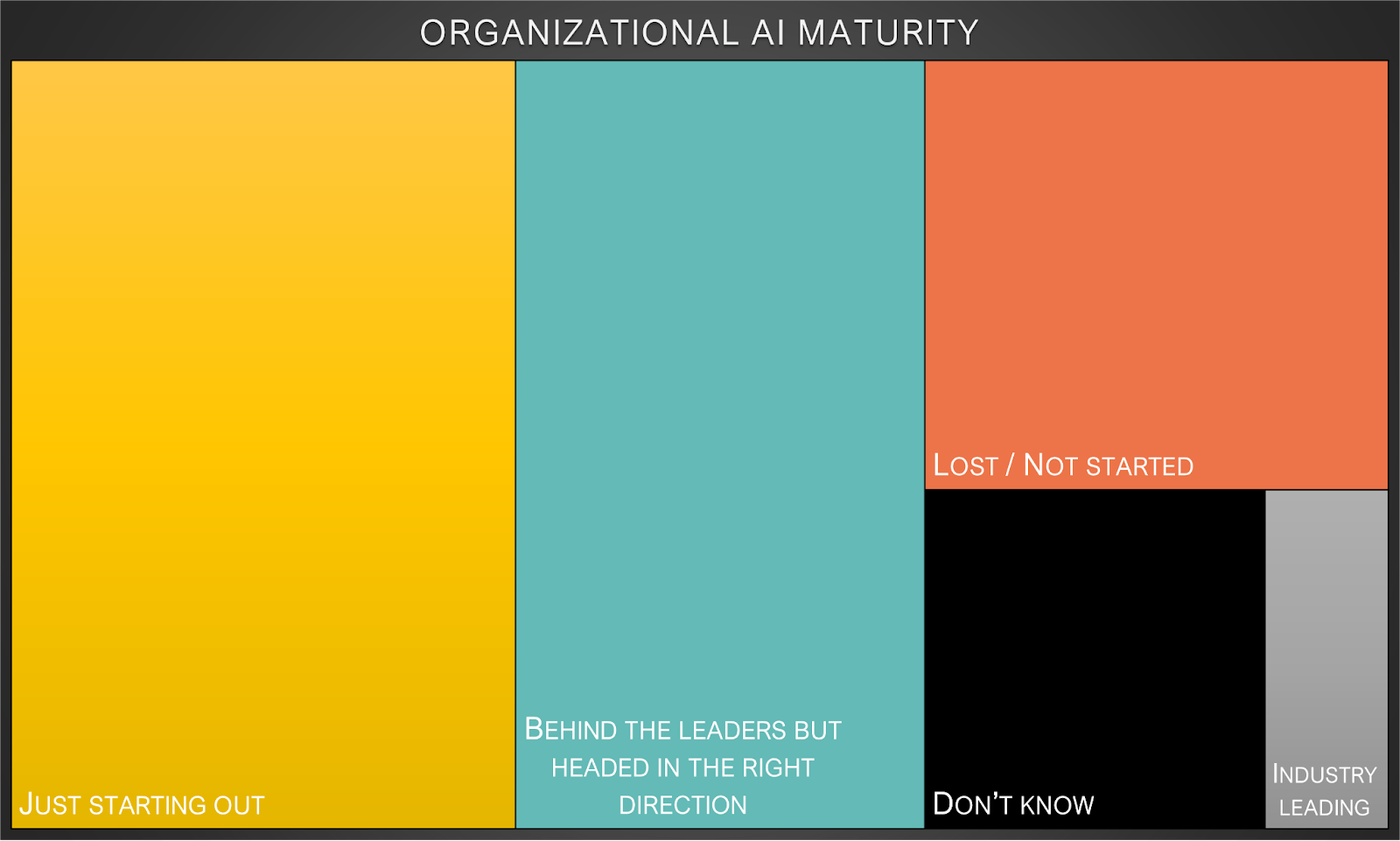
Broad, Scaleable Impact – If Approached Correctly
The world is rapidly coming to terms with how impactful AI will be, particularly with growing advancements and acceptance of new tools like those powered by GenAI. In order to ensure those impacts are positive, however, there are underlying concerns which must be addressed before such methods can be achieved at scale. This is especially true in the healthcare industry, where relying on the right data and decision-making process is extremely vital. As with the risks listed below, failing to responsibly address AI concerns can lead to dire outcomes, and should be approached with a great deal of care.
Responsible AI Commonalities and Considerations
The concerns represented in the left column above can be applied to a wide array of industries and use cases, as represented by examples in pharmaceutical and AI-powered skin disease detection during the first GenAI in Healthcare session. For that reason, organizations like the Responsible AI Institute and their partners are working to develop actionable frameworks, tools, and best practices which can offer guidance on how best to embark on the AI implementation journey.
While there are common elements of responsible AI, there are also specific considerations for each healthcare sector. In the next GenAI in Healthcare sessions, we’ll dive deeper into sectors such as Biotech, Pharma, Digital Health, and more, sharing specific case studies and insights from industry leaders about how to utilize powerful AI innovations responsibly in healthcare.
Session 2: Scaling AI in Biopharma
In the second session of the Generative AI in Healthcare series, speakers Satish Tadikonda(HBS) and Nikhil Bhojwani (Recon Strategy) led a discussion about current AI developments in the biopharma sector. The session featured exclusive interviews with experts from 5 leading organizations – Anna Marie Wagner (SVP Head of AI, Gingko Bioworks), Abraham Heifets (CEO, Atomwise), Michael Nally (CEO, Generate Biomedicines), Andrew Kress (CEO, HealthVerity), Stéphane Bancel (CEO, Moderna Therapeutics) – who shared their unique insights on how AI is being used today and how it will shape our shared future.
Current Use Cases
During the session, the speakers outlined a wide range of use cases for artificial intelligence (AI) across the biopharma value chain, including in Research and Development (R&D), Supply Chain, and Commercial.
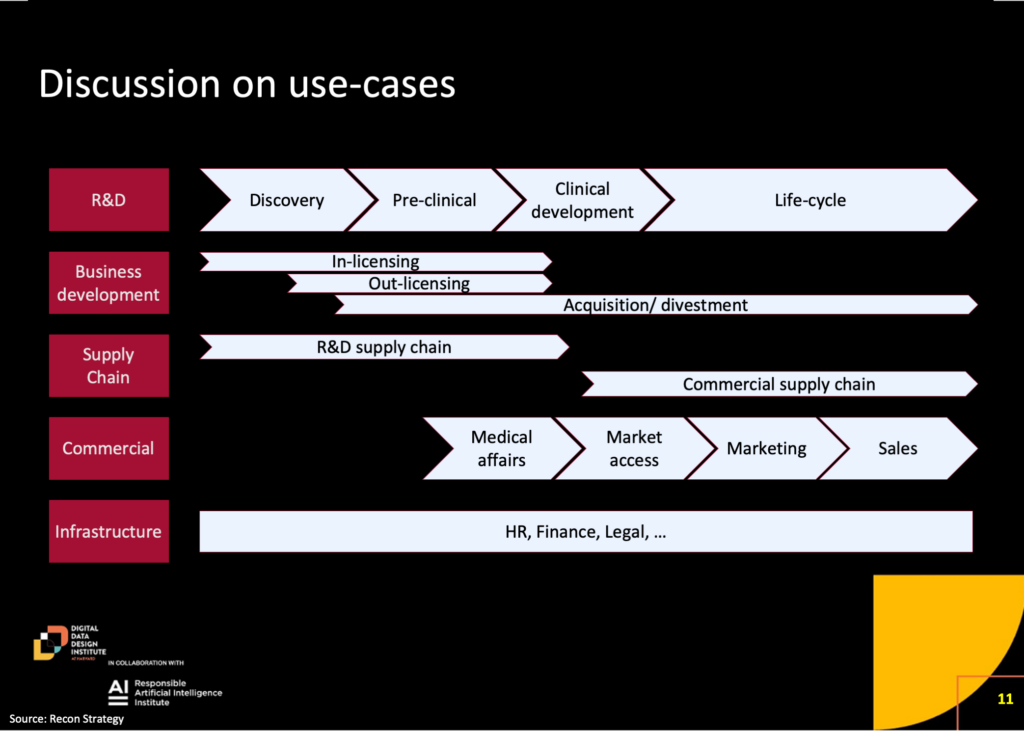
AI is being employed to accelerate and enhance drug discovery and to improve every step in development including better designed clinical trials and more efficient and less biased patient recruitment. Anna Marie Wagner emphasized the impact on drug discovery, stating that “very often the winning design, the best performing protein as measured in a lab, looks almost nothing like what the best scientists designed as a starting point, you know, might have 20% similarity to that original protein.” Both she and Mike Nally also shared that they view hallucinations as a feature rather than a bug, given that those previously unseen deviations generated by AI can sometimes lead to breakthroughs that scientists might not have otherwise found due to the complexity and computational burden of that analysis. The technology’s role encompasses tasks like generating documentation, drafting regulatory submissions, and aiding in medical writing. Stéphane Bancel talked about how Moderna is using its own version of ChatGPT, called mChat, to overhaul how medical and regulatory content is created. On the commercial side, AI applications span medical affairs, market access, sales, and marketing, with examples ranging from identifying rare disease patients to automating content generation for marketing purposes.
Our guest experts highlighted the transformative potential of AI across the biopharma value chain, noting that the use of large language models to streamline appropriate tasks showcases AI’s potential to significantly enhance efficiency and decision-making across the biopharma industry.
Associated Risks
The second segment centered around the potential risks associated with the widespread adoption of artificial intelligence (AI) in the biopharma industry. Our experts outlined a range of biopharma specific risks driving the need for responsible AI.
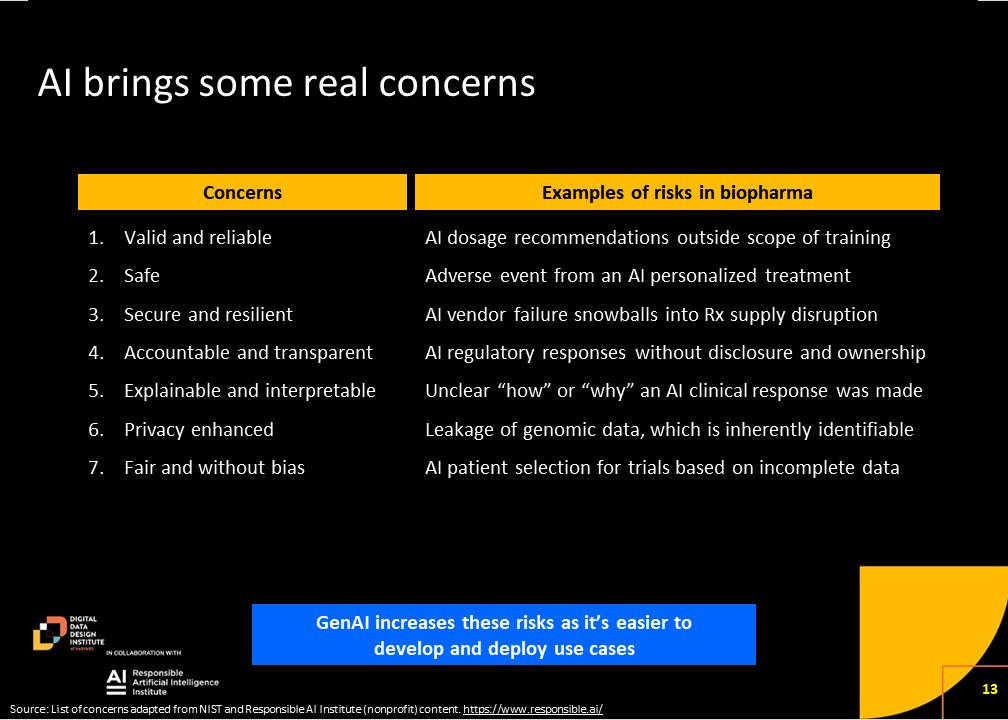
One notable concern is the scalability of regulatory processes, especially in clinical trials. The conversation highlights the challenges posed by an exponential increase in the number of new molecules entering clinical trials, potentially overwhelming regulatory bodies like the FDA. Andrew Kress also pointed out that “for biopharma, the risks that you would observe are the same as they would be in many other areas with one additional note, which is that because it’s such a regulated industry, there is a need for work that’s done to be both transparent and reproducible. One of the challenges that you would envision occurring here is the ability to look at a study or look at data or a conclusion and be able to go back and say, how did we get to that conclusion? And can we recreate that answer?”
Another significant concern revolves around responsible AI use and the broader ecosystem. The speakers delved into the need for regulations that go beyond the AI tools themselves, extending to the environment in which they operate. They underscored the importance of addressing not only the potential misuse of AI tools but also the broader biosecurity implications. The rapid advancement of AI technology, coupled with its accessibility, poses a dual challenge—excitement for innovation but also fear of potential misuse. The call for building a comprehensive ecosystem, including biosecurity measures, highlights the necessity of a cultural shift in understanding and addressing the risks associated with AI in the biopharma industry.
Envisioning the Future
Finally, the speakers discussed a future where the time required to analyze molecular data was drastically reduced, fundamentally transforming the workflow of scientists and clinicians. They reflected on a transformative vision for the biopharmaceutical industry, where AI acted as a catalyst for change rather than a supplementary tool. Their vision centered on biology becoming instantly programmable, marking a shift from the traditional trial-and-error approach to a more predictable and programmable paradigm.
Abe Heifets brought this home with a vivid example in which he described running a safety panel using AI rather than a couple of weeks saying, “You go get your coffee, you get back see what the results are, and then you design the next one…the work of the medicinal chemist isn’t replaced by the AI …the AI has replaced the assay.”
Mike Nally shared a vision reaching far into the future. “I think the next century will be the biology century, and it’s going to be through augmented intelligence – humans plus computational power – that will ultimately help us understand the mathematical underpinnings of biology in a way that allows us to increasingly change the probabilities of success in programming biology to improve human health.”
The potential of AI was seen by all of our speakers as a solution to long-standing challenges in research productivity and medication accessibility. Shared among them was the recognition that biology, augmented by artificial intelligence, has the potential to become a central information technology, shaping advancements in human health for many decades to come.
Stéphane Bancel summed it up succinctly, “I believe it (AI) is as big a disruption as the desktop computer.”
Session 3: Scaling AI in Digital Health
Insights from the January 31st, 2024 session on Gen AI use cases in the Digital Health sector
In the third session of the Generative AI in Healthcare series, speakers Nikhil Bhojwani(Recon Strategy) and Satish Tadikonda (HBS) provided a thought-provoking overview of the current digital health landscape, followed by an engaging panel discussion led by Alyssa Lefaivre Ĺ kopac (Responsible AI Institute). Panel speakers Payal Agarwal Divakaran, Reena Pande, and Andrew Le shared their valuable insights as investors, physicians, and executives at the forefront of AI in digital health.
Current Landscape
Nikhil Bhojwani kicked off the session with a presentation outlining the intersection of digital technology, artificial intelligence (AI), and healthcare. Bhojwani provided an overview of the pervasive influence of digital components across healthcare domains, illustrating the various ways in which AI was employed, including supporting, augmenting, and substituting human work. Examples were presented, ranging from AI synthesis for electronic health records to AI symptom checkers for diagnosis, prompting dialogue on the implications and ethical considerations of AI integration in healthcare tasks traditionally performed by humans. The conversation emphasized the need for a nuanced understanding of AI’s role in healthcare delivery and management, laying the groundwork for further exploration of its ethical, practical, and regulatory dimensions.
Opportunities in Digital Health
RAI’s Alyssa Lefaivre Škopac then initiated a discussion about the opportunities in digital health, particularly focusing on AI integration. Payal Divakaran elaborated on the different perspectives in adopting AI in consumer, physician, and enterprise-oriented use cases, and the importance of trust in AI adoption. Payal noted the lag in enterprise adoption compared to consumer-facing applications, queuing up Andrew Le to share his perspective on the benefits of consumer-facing AI applications, citing examples of how AI can empower consumers by processing complex healthcare data and providing user-friendly interfaces. Andrew expounded upon the transformative potential of AI in enabling consumers to make sense of healthcare data and navigate the healthcare system more effectively, and the significance of AI in optimizing back-office operations in healthcare was echoed amongst the panel members. Reena Pande also noted the importance of focusing on fundamental problems and considering the provider experience alongside patient outcomes and cost. She highlighted opportunities for AI to streamline administrative tasks, improve diagnostic capabilities, and augment patient treatment, urging for careful consideration of where AI can be responsibly applied. Reena underscored the need for a nuanced approach to AI deployment that serves the interests of all stakeholders.
Risks and Limitations
The panelists expressed a mix of skepticism and optimism regarding the integration of AI as a co-pilot in healthcare, acknowledging the need for trust-building and careful consideration of the nuances in healthcare. They discussed the challenges of ensuring responsible AI deployment, including issues of validity, safety, security, accountability, transparency, explainability, data privacy, and bias. Additionally, they highlighted the importance of addressing these risks to foster confidence and ensure the ethical and effective use of AI in healthcare, including the significance of transparent disclosures and establishing clear accountability frameworks within healthcare institutions. They also responded to keen observations from the audience regarding the need for cultural competency to ensure the unique and varied needs of each user, from patients and physicians to administrators and regulators, are being met by their AI solutions. In order to address these challenges, the panel reiterated the need for ongoing dialogue and collaboration between stakeholders to navigate the complexities of AI integration responsibly.
Accountable AI in Healthcare
The panel discussion then delved into the emerging guidance from regulatory bodies like the FDA on managing the integration of AI in healthcare. Participants engaged in a robust conversation about the accountability, transparency, and interpretability of AI systems in healthcare decision-making processes. They explored the traditional role of clinicians as the ultimate decision-makers and discussed the challenges and opportunities in distributing responsibility among various contributors, including AI systems.
As the session concluded, the moderators and panelists stressed the importance of establishing clear accountability frameworks and guardrails to mitigate risks associated with AI deployment in digital health. They emphasized the need for scalable access to data and partnerships between tech giants and healthcare incumbents to foster trust and manage risks effectively. The discussion underscored the complexity of evaluating AI tools and the necessity of ongoing dialogue and collaboration to responsibly address the evolving landscape of AI integration in healthcare.
Session 4: Scaling AI for Hospitals and Healthcare
Insights from the March 5th, 2024 session on Gen AI use cases among Healthcare Providers
In the fourth session of the Generative AI in Healthcare series, speakers Nikhil Bhojwani(Recon Strategy) and Satish Tadikonda (HBS) outlined the role of generative AI for hospitals and healthcare providers, followed by an engaging panel discussion with guest experts Marc Succi, Alexandre Momeni, Frederik Bay, and Timothy Driscoll, who shared their perspectives on current and future AI applications in the space.
Current Landscape
Nikhil Bhojwani discussed the potential applications of AI within the platform of health systems, emphasizing its role in various areas including clinical work, education, research, patient interaction, revenue cycle management, interoperability, and general organizational functions. Even within the complexity of health systems, there exist a multitude of opportunities for AI to augment, substitute, or support human activities across different departments and functions. Nikhil and Satish encouraged further exploration of specific use cases within each domain and invited the panelists to share their perspectives on the practical applications of AI in the context of hospitals and providers.
Opportunities in Digital Health
Marc Succi discussed various opportunities within Mass General Brigham, ranging from low-risk to high-risk endeavors, with differing timelines for implementation and adoption. While certain initiatives like streamlined prior authorization are already being implemented, more disruptive concepts such as clinical workflow and decision support are expected to take longer. Succi emphasized the importance of ensuring equity, enhancing patient experience, and addressing healthcare worker burnout in the implementation of AI technologies. Alexandre Momeni of General Catalyst further elaborated on three ways health systems can utilize AI: for innovation, transformation, and efficiency. He discussed the regulatory frameworks surrounding AI in clinical decision support and highlighted the potential for AI to significantly impact healthcare workflows. Boston Children’s Hospital’s Timothy Driscoll also outlined the institution’s numerous applications of AI, including operational efficiency, clinical decision support, research, education, and patient care, stressing the importance of responsible AI development and governance structures to maximize its benefits in healthcare settings. Frederick Bay also discussed Adobe’s focus on patient engagement and digital marketing expertise, utilizing generative AI to overcome traditional barriers to adoption in healthcare systems. He highlighted opportunities for personalized engagement and document management, including faster document creation, insights extraction from existing data, as well as image tagging and labeling, but clarified they were not currently focused on the clinical side of radiology due to security and legal considerations.
Strategic AI Implementation
Timothy Driscoll then described his strategic approach to the AI portfolio at Boston Children’s Hospital, focusing on objectives such as demonstrating AI’s impact on care quality, ensuring ethical and sustainable use, and driving efficiency and expertise. The hospital holds itself to key principles of diversity, fairness, accountability, and robust governance, fostering a commitment to inclusive and transparent AI development. Driscoll also discussed specific areas where AI drove value, including diagnostic support models and synthesizing complex patient data for frontline staff. He noted a phased approach to implementation, building foundational capabilities, defining prioritization frameworks, and rapidly scaling high-impact use cases. When asked about the use of synthetic data and compliance, Driscoll explained his team’s focus on leveraging actual patient data, but acknowledged scenarios where synthetic data was used for intelligent automations, such as resume scanning. Marc Succi also shared Mass General Brigham’s approach to AI adoption, outlining the importance of research and validation through their data science office. He noted the challenges of FDA approval versus actual adoption in patient care, which raises the need for socialization and education within the healthcare community. Succi discussed the deployment of low-risk tools to familiarize users with AI concepts and mentioned ongoing investigations into clinical decision support algorithms, noting the impact on operational use cases in clinical settings.
Risk and Responsibility
To close out the session, Nikhil Bhojwani shared some of the unique risks related to irresponsible AI use in healthcare, referring to the Responsible AI Institute’s framework to categorize risks. Among the examples Bhojwani gave were inaccuracies in AI-generated notes by scribes, safety concerns regarding AI-driven drug delivery systems, resilience issues with predictive models like sepsis detection, accountability challenges in AI recommendations, explainability difficulties, privacy risks from de-anonymization of data, and fairness concerns due to biases in training data. These use cases illustrate the multifaceted nature of AI risks in provider systems and underscore the need for robust solutions to ensure responsible implementation.
Momeni added that trust in AI systems is of utmost importance and suggested three key considerations: the degree of automation, benchmarking and evaluation methods, and the establishment of industry standards. Bay noted that transparency and governance processes are also key to establishing trust in AI development, while Succi and Driscoll both emphasized the importance of checks and balances to ensure responsible use. As an example, they mentioned existing practices where physicians review AI-generated notes and reports, driving home the consensus that human accountability remains crucial, especially with potential concerns about over-reliance on AI. The panel agreed that with robust accountability mechanisms in place, such tools could be used to vastly improve the experience of both patients and providers.
Session 5: Lessons in Applying Responsible AI
In the capstone session of the Generative AI in Healthcare series, Satish Tadikonda (HBS) spoke with Responsible AI Institute experts Manoj Saxena, Var Shankar, and Sabrinah Shih as they outlined current applications of generative AI in healthcare through the lens of two case studies. The conversation included exclusive interviews with Veronica Rotemberg (Memorial Sloan Kettering Cancer Center) and Rakesh Joshi (Skinopathy), who shared their perspectives on responsible AI implementation, and closed with a broader discussion on how these lessons can be applied in a variety of AI contexts.
Responsible AI as a Foundation for Positive Impact
Satish Tadikonda and Manoj Saxena kicked off the session by discussing the key questions at the core of this series – can generative AI have a positive impact in healthcare, and if so, how can such tools be responsibly deployed? Tadikonda summarized the prior sessions with a resounding yes, that AI can indeed produce positive impacts in healthcare, but that it is crucial to do so responsibly due to societal concerns and the varying adoption levels across healthcare domains. Saxena affirmed that enterprises are applying AI with cautious excitement, and outlined a framework anchored in NIST guidelines to operationalize responsible AI. He illustrated examples of potential AI harms, such as incorrect dosages and data privacy breaches in an NHS project involving a generative AI chatbot, advocating for a comprehensive approach to ensure AI’s positive impact. He also underscored the need for responsible AI governance from the top levels of organizations, integrating principles and frameworks early in AI design to mitigate risks effectively.
Applied Use Case – Memorial Sloan Kettering Cancer Center
Sabrina Shih then took the helm to explain the use of skin lesion analyzers in detecting cancerous lesions, outlining their integration into patient diagnosis journeys through self-examination support and aiding clinicians. Shih highlighted the benefits of such analyzers, including convenience and triaging support, while also discussing the advancements in generative AI for skin cancer detection. In an interview, Dr. Veronica Rotemberg, Director of the Dermatology Imaging Informatics Group at Memorial Sloan Kettering Cancer Center, discussed the institution’s approach to evaluating an open-source, non-commercial dermatoscopy-based algorithm for melanoma detection. She delved into the characteristics of trustworthy AI and emphasized the need for large collaborative studies and efficient validation processes to improve the accuracy and efficiency of AI algorithms. She also addressed concerns regarding biases and the challenges in evaluating AI performance in clinical scenarios, stressing the importance of multidisciplinary collaboration and thoughtful consideration of potential harms to ensure patient safety and well-being.
Applied Use Case – Skinopathy
In the second case presented, Dr. Rakesh Joshi, Lead Data Scientist at Skinopathy, discussed the company’s patient engagement platform and app called “GetSkinHelp.” The app leverages AI to provide remote skin cancer screening for patients, facilitate scheduling options, and enable triaging of cases for clinicians. Dr. Joshi emphasized three key characteristics of trustworthy AI platforms: consistency of results, accuracy against benchmark data sets, and error transparency. He explained Skinopathy’s approach to addressing these characteristics, including ensuring reproducible results across different skin regions and types, benchmarking against open-source data, and being transparent about system limitations and biases. Dr. Joshi also noted the importance of randomized clinical trials to validate the platform’s reliability across diverse demographics, and the need for ongoing recalibration and data collection to maintain accuracy, particularly in underrepresented geographic areas or demographic groups. Additionally, he highlighted the company’s careful consideration of patient privacy and the collaborative decision-making process involving stakeholders such as patients, physicians, and ethicists in determining the platform’s features and data usage. He also discussed strategies to mitigate biases in clinical decision-making, such as presenting AI results after clinicians have made their assessments. He asserted that the aim of the tool is to support clinical decision-making rather than replacing it, and to maintain simplicity for patients while providing detailed information to clinicians.Â
Transferrable Takeaways
The throughline of these case studies was that AI applications are duty-bound to prioritize safety, accuracy, and transparency in developing solutions for patient care. Var Shankar closed out the presentation by emphasizing the importance of drawing lessons from these examples to develop a responsible approach for organizations seeking to deploy AI in healthcare and other industries. He outlined key steps, including building on existing knowledge, understanding the benefits and pitfalls of AI, defining trustworthy characteristics, investing in testing and evaluation, and ensuring external checks are in place.
In the Q&A that followed, the speakers also delved into the role of culture in applying AI solutions, with Manoj Saxena highlighting the need for leadership in cultivating an environment which supports experimentation and lifelong learning. The conversation further explored the implications of AI regulation, particularly in the context of new global AI laws, and the need for responsibility to be a key facet throughout the AI pipeline, rather than an afterthought once the technology has been built. Saxena also elucidated that responsible AI can be profitable for institutions under financial pressure by separating AI investments into “mundane vs moonshot” buckets, starting with cost-saving projects that boost efficiency before investing in more transformative initiatives, thereby creating benefits for both AI-driven organizations and the audiences they seek to serve.
Bonus Content
Below are additional insights our expert speakers shared during their full-length interviews with the D^3 Institute. Topics include generative AI applications, risks, strategic approaches to responsible implementation, and hypotheses on the future of AI in biopharma.
Bonus Videos
Speakers: Mike Nally, Anna Marie Wagner, Stéphane Bancel
Hosts: Satish Tadikonda, Nikhil Bhojwani
Speaker: Andrew Kress
Hosts: Satish Tadikonda, Nikhil Bhojwani
The Gen AI in Healthcare series is collaboratively produced by Harvard’s Digital, Data, Design (D^3) Institute and the Responsible AI Institute.
About Responsible AI Institute
Founded in 2016, Responsible AI Institute (RAI Institute) is a global and member-driven non-profit dedicated to enabling successful responsible AI efforts in organizations. RAI Institute’s conformity assessments and certifications for AI systems support practitioners as they navigate the complex landscape of AI products. Members include ATB Financial, Amazon Web Services, Boston Consulting Group, Yum! Brands and many other leading companies and institutions collaborate with RAI Institute to bring responsible AI to all industry sectors.