The D^3 Institute recently convened the Generative AI Collaborative Working Group in order to build collective knowledge around the subject of equitable AI implementation. This global, interdisciplinary group of experts gathered weekly to discuss the ways in which generative AI will likely continue to impact various sectors and societies. Through a series of interactive discussions and systems thinking exercises, a non-exhaustive list of recommended best practices was developed, and is now being publicly shared as a free tool to help organizations ensure that they are using AI solutions equitably, ethically, and effectively.
Written by Kelsey Burhans, based on interdisciplinary discussions with the D^3 Institute’s GenAI Collaborative Working Group. The Institute extends its sincere thanks to the group’s esteemed members for lending their perspectives to these very impactful conversations: Marily Nika, Daniel Favoretto Rocha, Tommaso Davi, Alsa Khan, Ketaki Sodhi, Zara Muradali, Paula Garuz Naval, Nelly Mensah, Val Alvern Cueco Ligo, and Ivana Vukov.
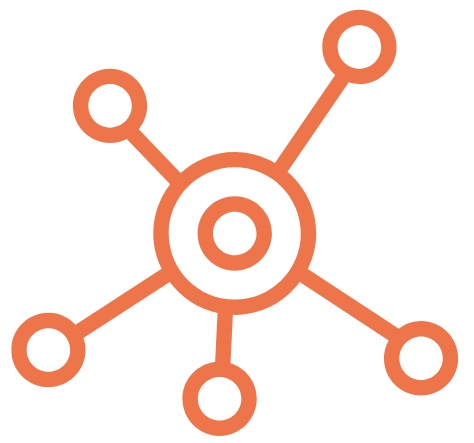
Foundational Data Principles
Certified Data Imperative
Given the potential impact and sensitivity of certain generative AI applications and sensitive use cases, there’s a growing consensus on the need for certified or pre-approved datasets. These curated datasets ensure that the AI operates within defined ethical and accuracy parameters, preventing inadvertent biases and enhancing the reliability of generated outputs.
Design Defines Success
To ensure the success of any generative AI initiative, it is imperative to meticulously define and design the experiment. This not only sets clear objectives and boundaries but also dictates the quality of outcomes.
Data Determines Precision
The importance of collecting pertinent data cannot be overstated. The right dataset serves as the foundation, ensuring that the AI system is trained effectively, accurately reflects the nuances of the task, and delivers meaningful results.
Evaluation Drives Adaptability
Utilizing historical data for continuous evaluation and enhancement of the model is fundamental to ensuring the robustness, adaptability, and relevance of generative AI systems across domains.
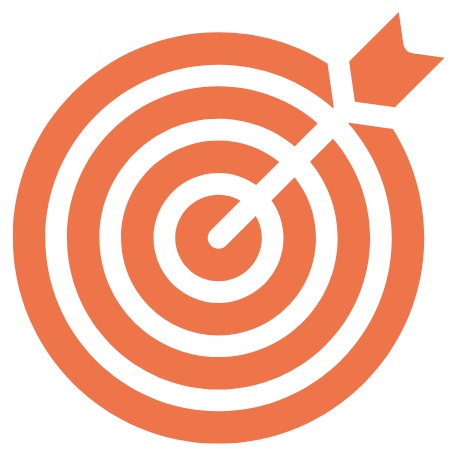
Continuous Feedback and Impact Evaluation
Values-driven Feedback Loops
Establish core values early and often, performing ongoing impact analysis to ensure the product is in alignment with those goals. Gather qualitative and quantitative data from users, feature interactions, and platforms about how tools are being used. Incorporate these findings into product iterations and policy adaptation.
Iterative Implementation Strategies
Consistent red-teaming evaluations, combined with ongoing production testing and meticulous documentation of issues, are vital for identifying potential risks and formulating mitigation strategies in generative AI systems. These periodic reviews should be designed to assess a variety of trends, from efficiency and bias in the model itself to sociological shifts in areas such as corporate culture or public perception of the tool. If tested and identified in a timely manner, the organization can readily adapt to these changes.
Big Picture Visualization
Aggregate feedback across use cases and stakeholders to better understand the strengths and vulnerabilities of the generative AI ecosystem as a whole. Be sure to include input from both direct users and those indirectly affected by the product and its outputs, as these form a more complete picture of any tool’s societal impact. Collaboration and knowledge sharing across AI-driven organizations is key to creating this type of systems thinking.
Examining the Entire GenAI Pipeline
There are many human and environmental inputs to generative AI products. Assessing the underlying infrastructure, mineral resources for hardware, energy and water use in computation, labor rights, land use (and possible community displacement) involved in the development process are all aspects which can often be overlooked. The costs of these inputs also factor into the economic and technological accessibility for consumers and the long-term viability of the product, so it is imperative for both the AI organization and society that they are taken into account.
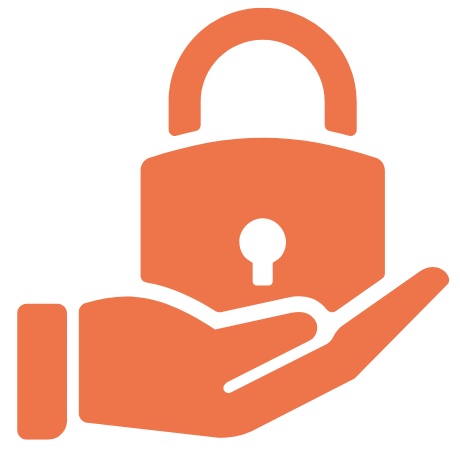
Privacy Considerations
Strategies to Protect Personal Rights
Clear guidelines, consent protocols, and robust encryption methods are essential to ensure that data collection doesn’t infringe on personal rights or become susceptible to misuse. Although many countries currently have data privacy regulations, it is in the best interest of the AI product owners to establish substantial internal processes which meet or exceed those requirements.
Discernable Data
Users should be in charge of their data, which entails the use of transparent, clear language about what is being collected, how it will be used, how long it will be stored, and how to opt out of further data usage. Likewise, the company should have clear internal justifications for the data they collect. Wherever possible, only the most relevant and necessary data for a given use case should be collected, and datasets should be continuously evaluated for extraneous sensitive user data.
Build vs Buy
One way to limit liability and ensure data collection is appropriate for its use case is to evaluate whether a new model or dataset is optimal for the success of the application. In cases where pre-established and vetted data is available and well suited to the model, it may be best to source from a trusted vendor. However, if the application relies on unique or novel sources of data, that investment is best attributed to building capable teams and processes who can ethically create the specific model required.
Balancing Biometrics and Privacy
As the need for generative AI expands, so does its appetite for diverse data, including biometrics. Biometric data, derived from real-time behaviors, can enhance the depth and realism of generated outputs. However, the collection of such personal and sensitive data presents undeniable privacy concerns. It becomes paramount to strike a balance between harnessing the potential of biometric data for generative AI and safeguarding individual privacy.
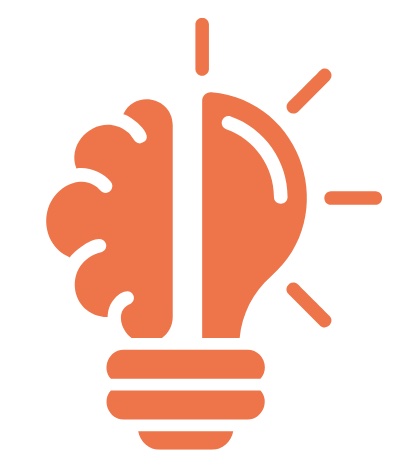
Stakeholders and Skill Sets
Stakeholder Engagement
Participatory processes where stakeholders are engaged at every stage of development have a multitude of benefits. For enterprises, engaging both users and indirectly affected stakeholders mitigates unanticipated negative externalities, establishes a product-market fit by correctly identifying the problem and meeting it with the right solution, and creates an audience of adopters by establishing trust and dialogue from outset to implementation. For stakeholders, this offers the opportunity to shape solutions and feel some ownership of how they interact with the world through new technologies.
Interdisciplinary Insights
Generative AI, and AI as a whole, will continue to have far-reaching impacts that span many fields and applications. It is crucial that input from a wide range of disciplines is incorporated, rather than limiting the perspectives shaping these trends to those of a select group of technologists. Sociologists, environmental scientists, legal experts, and many other practitioners have valuable insights that can contribute to the efficacy and longevity of AI.
Skill Development
Framing AI as a tool which can help practitioners do their jobs more effectively, and offering equitable and accessible training opportunities to upskill employees, can make change management more seamless. One of the strengths of AI should be increased usability, including for those with less technical acumen, so taking steps to establish trust and competency among the workforce will be mutually beneficial to individuals and the larger organization.
AI-Assisted Evaluation
In cases where AI is used in hiring processes or employee evaluation, it is critical that evaluations are a) specific to the role, level, industry, etc. in which they are implemented, b) include rigorous anti-discrimination safeguards so as not to perpetuate pre-existing biases. Human oversight continues to be a crucial component in ensuring fair outcomes of AI-assisted evaluation processes.
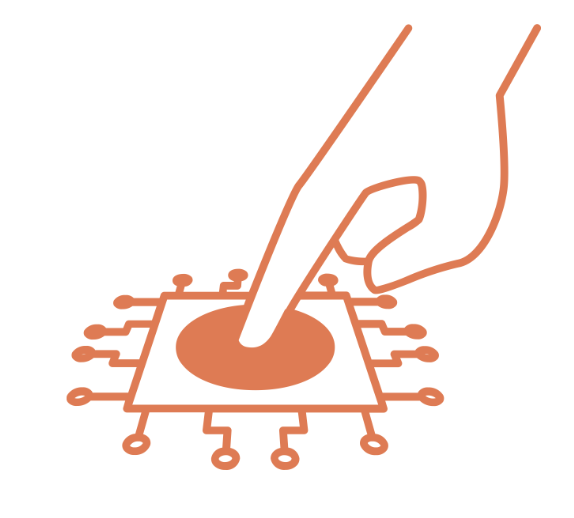
Human-Computer Collaboration
Joint Decision-Making
Practitioners must remember that, at its core, AI is meant to help humanity. While AI can assist in achieving goals and completing tasks, humans must still have the final say in what. Getting the right supervision agents and decision-makers involved for relevant use cases ensures that AI can function effectively and ethically as an informative tool that can then be acted upon by humans.
Transparent Outputs
In addition to closely examining the inputs that guide generative AI models, it is important to include upfront labeling of outputs which highlights the role AI played in their creation. Digital watermarks and blockchain identification are some emerging examples of how to preserve the fidelity of AI-generated works.
Enabling Oversight
While not every negative use case can be accounted for prior to implementation, there should also be a clear evaluation and decision process for flagging, reporting, assessing, and addressing malicious activity.
Establishing Trust
Generative AI enterprises have an immense responsibility to build trust with the public, and in doing so must acknowledge the emotional role of adoption. Stakeholders will have more confidence in an AI tool if the organization takes steps to model human-computer interactions to ensure they are fruitful and productive, and establishes processes which demonstrate accountability, safety, and alignment with stated values throughout.
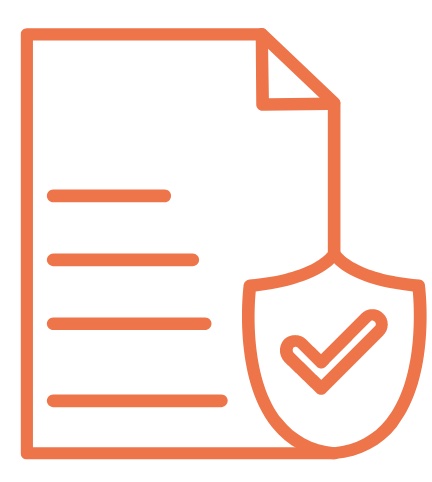
Regulation – Internal Enterprise Policy and External Legislative Oversight
Bounding Conditions
Although generative AI is a powerful tool, it is not the right solution for every problem. As society explores its potential, it is important to also regulate the types of use cases where AI can ethically be used, and identify the edge cases where its involvement is unacceptable. In instances where AI is deemed appropriate, internal and external policies must be applied while the product is being developed so as to anticipate and manage side effects.
Informed Oversight
As with development and implementation, the involvement of a variety of stakeholders in regulation, in addition to the tech leaders driving product creation, will help ensure all possible outcomes are accounted for. Regulators should also be educated on system inputs and outputs in order to better understand the near- and long-term impacts that legislation would seek to address.
Equitable Inputs
Much attention is already being paid to intangible inputs, such as unbiased model training and the protection of user data. There are also many tangible inputs deserving of regulators’ attention, such as infrastructure requirements, energy and resource use (land, water, minerals, etc.), and human labor, all of which play a role in successful generative AI implementation. Protections are especially crucial in regions where technological development has previously created gaps in equality by exploiting local resources and communities, as these issues have the potential to be exacerbated by fast-moving, computationally intensive projects like those in the generative AI sphere.
Equitable Outputs
Both internal AI enterprise policy teams and external regulatory bodies should include mechanisms which ensure generative AI outputs are creating societal benefits rather than societal harms. There are a variety of strategies to address potential risks, including regulatory sandboxes, guards against misinformation such as blockchain verification or deepfake detection, clear takedown procedures, committees to audit and enforce compliance, and many more. In order for generative AI to function in the public interest, its outputs must be safe, accessible, affordable, and trustworthy, all of which are foundational to an equitable AI future.