Analysis of financial disclosures for publicly traded companies can be a time consuming and costly process these days. In the study, “Scaling Core Earnings Measurement with Large Language Models”, Matthew Shaffer, an Assistant Professor at USC’s Marshall Business School, and Charles C.Y. Wang, the Tandon Family Professor of Business Administration at Harvard Business School, explore how large language models (LLMs) can be leveraged to estimate core earnings from corporate 10-K filings. Their research demonstrates the potential of AI to revolutionize financial analysis, offering a scalable and cost-effective approach to assessing firms’ persistent profitability.
Key Insight: With Proper Guidance, LLMs Can Effectively Estimate Earnings
“Our results offer empirical support for anecdotal claims that these models can fail when used ‘out of the box,’ on complex tasks without sufficient guidance; but can perform remarkably well when properly guided.” [1]
The researchers tested two approaches: a “lazy analyst” method, with minimal guidance, and a “sequential prompt” strategy that broke down the task into structured steps. Shaffer and Wang found that when given minimal instructions (the lazy analyst), LLMs often made conceptual errors in estimating core earnings. However, when provided with a structured, step-by-step approach (sequential prompt), the models produced valid core earnings measures that outperformed traditional metrics in predicting future earnings.
Key Insight: AI-Generated Core Earnings Measures Show High Persistence and Predictive Power
“[T]he sequential LLM prompt’s core earnings measure and Compustat’s OPEPS emerge as the top performers, with the highest predictive coefficients and R²’s. However, notably, when we extend the prediction horizon to average net income over the next two years, the sequential LLM-based measure surpasses all other measures.” [2]
Shaffer and Wang found that the AI-generated core earnings measure using the sequential-prompt approach better captured the persistent components of earnings that are reflected in market valuations over longer horizons. When predicting stock prices two years ahead, the Sequential Prompt Core Earnings per Share measure achieved an adjusted R²1 of 0.7585, outperforming both Compustat measures and GAAP net income. This alignment between market valuations and stock prices seen at longer horizons, shows that the information available through the sequential prompt approach could be effectively utilized by investors when determining future stock prices.
Key Insight: LLM-Based Core Earnings Estimates Are Highly Cost-Effective
“The performance of this LLM-based measure — based on an API call costing less than one dollar and one minute of compute time on average per firm — is striking, particularly given the time- and cost-intensive processes associated with the alternatives.” [3]
One significant advantage of the LLM-based approach is its efficiency and cost-effectiveness. The authors note that their method produces core earnings estimates at a fraction of the cost and time required for traditional approaches, which could greatly reduce the expenses associated with processing and analyzing financial disclosures. The authors highlight that the advancements in ChatGPT-4 were pivotal in enabling the completion of an analysis like this one, as its predecessor, ChatGPT-3.5, lacked the necessary analytical capacity. They further suggest that, if the capabilities of LLM models continue to progress as anticipated, leveraging LLMs for such analyses could become a standard practice.
Why This Matters
Shaffer and Wang’s findings highlight the transformative potential of AI in financial analysis, particularly for decision-makers navigating the growing complexity of corporate disclosures. The AI-powered approach to core earnings estimation offers a scalable, cost-effective solution that delivers high-quality insights at a fraction of the time and cost of traditional methods. This innovation could be especially valuable for smaller firms and individual investors who lack access to expensive financial services, leveling the playing field in a data-intensive industry. Moreover, the AI-generated measures’ strong predictive power and correlation with market valuations suggest their utility in investment decisions, strategic planning, and performance evaluation.
However, the study emphasizes the importance of careful implementation when adopting AI tools for financial analysis. The stark difference in performance between the “lazy analyst” and “sequential prompt” approaches underscores the need for well-designed, structured prompts to harness the full potential of these technologies. As AI continues to evolve, it presents an opportunity for business leaders to integrate these tools into their processes, in order to enhance efficiency.
Footnotes
(1) R2 (r-squared), also known as the coefficient of determination, is a statistical measure that represents the proportion of the variance in a dependent variable that is explained by an independent variable or variables in a regression model. R2 normally ranges from 0 to 1: an R2 of 0 indicates that the model does not explain any of the variance in the dependent variable, an R2 of 1 means that the model perfectly explains all the variance in the dependent variable. That is to say, generally, the closer the R2 arrives to 1, the stronger the relationship between earnings predictions and future actual earnings and market valuation.
References
[1] Matthew Shaffer and Charles C.Y. Wang, “Scaling Core Earnings Measurement with Large Language Models”, Available at SSRN (October 8, 2024): 1-45, 6.
[2] Shaffer and Wang, “Scaling Core Earnings Measurement with Large Language Models”, 4.
[3] Shaffer and Wang, “Scaling Core Earnings Measurement with Large Language Models”, 5.
Meet the Authors
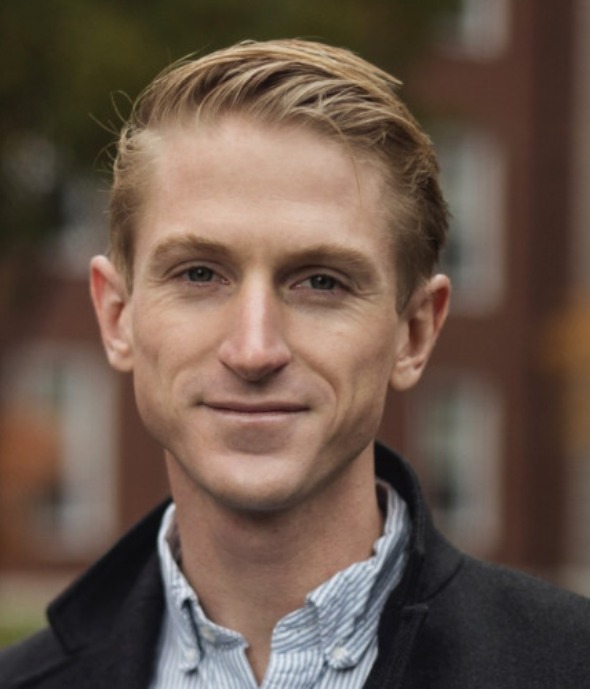
Matthew Shaffer is an Assistant Professor at USC’s Marshall Business School. He received his doctoral degree from Harvard Business School and his bachelor’s degree from Yale. His research focuses on valuation and corporate governance, especially valuation practice in institutional settings such as M&A. His work has been published in the Journal of Financial Economics, and presented at leading conferences in accounting, finance, and law.
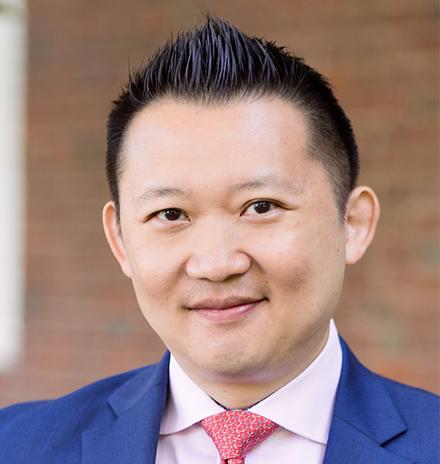
Charles C.Y. Wang is the Tandon Family Professor of Business Administration at Harvard Business School. He is a research member of the European Corporate Governance Institute (ECGI) and an associate editor of Management Science and Journal of Accounting Research, two leading management journals. His research and teaching focus on corporate governance and valuation.