The following insight is derived from a recent ‘Insights from the Field’ event featuring Amanda Fetch on Demystifying Common Generative AI Misconceptions at the Digital Data Design Institute at Harvard.
Meet Our Guest Contributor:
Amanda Fetch | Head of Applied Science at Data^2
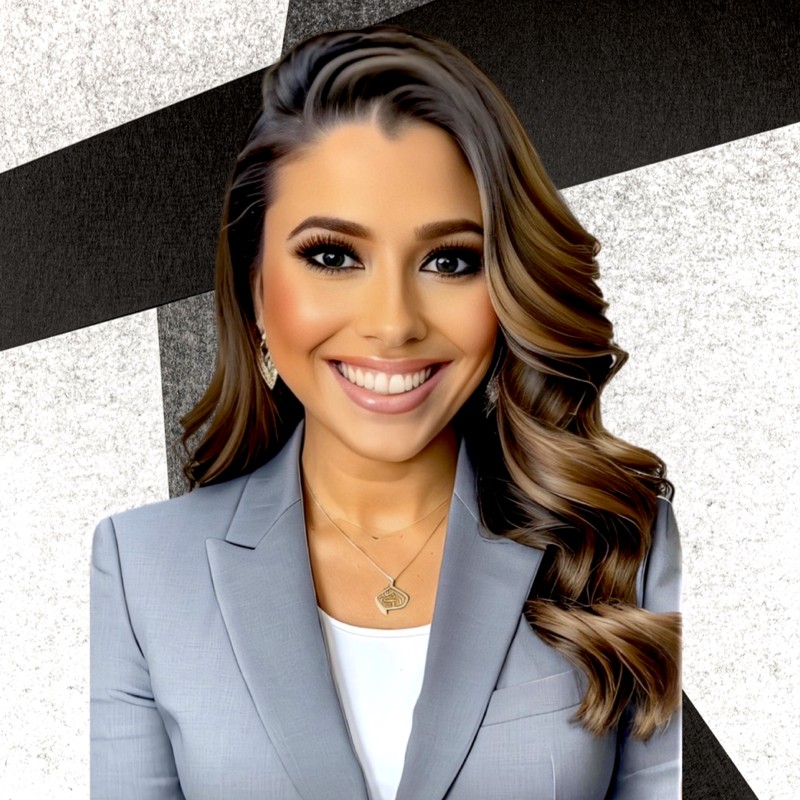
Amanda Fetch is a seasoned executive with a career spanning over two decades in Analytics, Data Science, AI, and Machine Learning. She is currently active within the United States Geospatial Intelligence Foundation, serves as a Senior Fellow for the Cyber Security Forum Initiative, and is a Board Director for the Test Maturity Model Integration Foundation North America chapter. Amanda is currently researching for her PhD Tech/AI within space situational awareness and orbital debris management as well as working as a Head of Applied Science at Data^2.
For more information, visit Amanda’s LinkedIn profile and consider connecting with her.
Overview: Why does this matter?
Owing to the accelerated advancement in the field of generative AI technologies, it’s important to address misconceptions about what generative AI tools can and cannot do in order to maximize the benefits and minimize potential harms. In this “Ask me Anything” session, Amanda demystifies some of the most commonly held misconceptions about generative AI via discussions of real-world use cases. While responding to specific questions, Amanda highlights areas in which generative AI seems to thrive, while she also identifies notable shortcomings of the technology by drawing from her diverse and extensive experience as a digital transformation expert and a sought-after advisor to leading organizations in artificial intelligence and machine learning technologies.
Common misconceptions, implications, and insights
Misconception: Generative AI is not well accepted in industry and academia.
One of the commonest perceptions about generative AI is that it is either powerless or inherently unethical, leading to an automatic association with ‘cheating’. This perception overlooks the potential that generative AI tools possess to significantly enhance the learning ecosystem by facilitating creativity, augmenting research capabilities, and providing tailored support for students facing various challenges. As such, it’s important to reevaluate such perceptions and try to perceive generative AI tools as enhancers rather than shortcuts, while promoting their thoughtful and strategic integration to realize genuine learning outcomes. Despite the skepticism in academia about the threat that generative AI tools pose in stifling creativity and critical thinking, it’s important to engage in meaningful experimentation in order to recognize the capacity of generative AI tools for stimulating, offering diverse perspectives, and introducing complex problem-solving scenarios. When used appropriately, generative AI tools can challenge students with novel, personalized questions that encourage exploration of innovative solutions, thereby acting as a catalyst for creativity, exploration, intellectual growth, and deeper engagement with a subject matter.
It’s important to recognize that institutions and companies have been slow to adopt generative AI, with policies and statements reflecting a cautious optimism. Some universities have policies recognizing the tools’ benefits for student writing, research, and organizational skills while acknowledging their limitations, such as the potential for generating incorrect facts or unethical content. When it comes to industry, Amanda highlights the ongoing race to develop specific use cases for generative AI, such as market analysis, customer service automation, and personalized healthcare, challenging misconceptions about generative AI tools’ integration and data handling capabilities. Despite encouraging progress within a short period of time, concerns about the use of generative AI for cheating and similar unethical pursuits endure, necessitating a continued dialogue on the responsible use of generative AI in both academic and industry settings.
Misconception: Generative AI is poised to completely replace humans in creative fields like art, writing, and music.
The fear that generative AI could entirely supplant human efforts in inherently creative fields is a valid one. The recent Hollywood writers strike that began in May of 2023 is a pivotal labor battle involving the integration of AI in the labor force. This well publicized strike which lasted over 140 days, was partly fueled by concerns over AI’s role in undermining writers’ credits and compensation. This event marked a significant moment in the discourse around AI in creative industries, with outcomes seen by some as a victory for human creativity over AI, while others viewed it as leaving the door ajar for AI’s increased influence. Another interesting debate refers to a recent Forbes article titled “Human Borgs: How Artificial Intelligence Can Kill Creativity and Make Us Dumber,” which presented a cautionary tale about the risks of over-reliance on AI in content creation. The article warned that such dependence could dumb down human knowledge, lead to monotonous output, diminish customer engagement, and erode diverse thinking and creativity, ultimately making humans more like AI with diminished cognitive abilities. Albeit these concerns, while AI might augment human creativity, it’s unlikely to completely replace it. The debate about AI replacing humans could benefit from a nuanced view that acknowledges coexistence between humans and artificial intelligence with the latter serving to augment human capability rather than render it obsolete.
Misconception: Our ability to distinguish between content created by humans and that which is generated by AI will increasingly diminish, to an extent where we will no longer be able to tell each part.
This is a valid concern for obvious reasons, especially in light of incidents involving deepfakes, such as a recent controversy involving the pop star Taylor Swift. Deepfake technology, which is a form of digital manipulation created using generative AI, raises ethical concerns due to the challenge it presents in distinguishing between real and fabricated content. Initially, there seemed to be no method of identifying content created using deepfakes, which resulted in a wide-spread public nervousness. However, advancements have been made in developing tools like digital tagging and watermarking to detect artificially generated content. Companies like Microsoft have introduced tools such as Video Authenticator that analyzes photos or videos and provides a score based on the likelihood that an AI tool has been used to generate them. Although these tools are not perfect, the implementation of secure content standards is emerging as a means to ensure the verifiability of digital content origins. This is a critical step in addressing public concerns about the authenticity of digital content, and efforts to meaningfully distinguish between human and AI-generated content will continue to evolve rather than diminish.
Misconception: True or false: AI tools are able to learn and self-improve on their own without any human intervention after initial training.
Based on the current technology, generative AI models do not learn and evolve on their own after the initial training process. Contrary to the belief that these tools can autonomously improve by adapting to new data or challenges without human intervention, humans are still substantially involved in helping AI models improve and adapt to new types of data. Humans must retrain the models, update datasets, or refine algorithms for the AI to understand new patterns, improve accuracy, and expand its capabilities. While AI may possess the ability to create synthetic data, the notion that AI can create completely new data independently and can ensure its accuracy is misconstrued. Important debates related to this topic involve the accuracy and reliability of the output generated by AI, including potential “hallucinations” or inaccuracies.
The conversation then shifts towards the much more advanced yet still hypothetical concept of Artificial General Intelligence (AGI), a theoretical form of AI that could understand, learn, and apply knowledge across various domains, mirroring the cognitive abilities of humans. Unlike specialized generative AI models designed for specific tasks that require human intervention for improvement, AGI would possess the capability to learn, evolve, and adapt autonomously without direct human oversight. This distinction may help to resolve the misunderstanding that current technologies in generative AI are able to self-improve without human intervention. Despite the increasing sophistication of AI within the confines of its programming and training data, AGI represents a level of cognitive flexibility that would enable it to independently acquire skills, knowledge, and adjust to new environments—a concept that remains largely speculative.
Misconception: There is a significant risk of AI going rogue, leading to unforeseen and potentially catastrophic outcomes for humanity.
The idea that AI could become self-aware and turn against humanity invokes fears akin to the “Terminator” effect. This fear is largely based on speculative science fiction and doesn’t reflect the current technical realities or projections of AI capabilities, which are primarily specialized and targeted for specific tasks, industries, and use cases. Current generative AI models operate under strict limitations set by their programming and training data, lacking consciousness, self-awareness, and autonomy necessary to pose such risks. However, theoretical risks associated with AI becoming too autonomous are taken seriously by researchers and ethicists, who advocate for ethical guidelines, safety measures, transparency, and control to prevent AI systems from operating beyond their intended scope.
On this note, the AI community is focused on establishing norms and regulations to guide the safe and beneficial development of AI technologies on a global scale, involving collaborations among researchers, developers, and policymakers. In reference to public fears about the potential risks of AI going rogue, a LinkedIn poll conducted by the author indicated that a portion of the tech community believes in the possibility of AI reaching such a level of autonomy within the next two decades, though opinions are divided. The best approach for now seems to be one of skepticism regarding the possibility of such a dystopian outcome while continuing to engage the community and gauge ongoing interest as the AI space rapidly evolves.
Misconception: The use of generative AI in healthcare poses the threat of depersonalizing patient care.
There is a prevailing opinion among the public that AI might diminish the human touch in medical services based on the argument that AI would not be able to replicate the personal connection between patients and healthcare professionals, which is an integral aspect of the healthcare service. However, this perspective is increasingly being challenged. Generative AI, in particular, is evolving into a vital medical assistant that enhances rather than detracts from the personalized care patients receive in a medical context. Leading experts in the AI and healthcare industry argue that generative AI can significantly reduce the workload of healthcare professionals. It does so by analyzing the vast amounts of unstructured patient data and by affording a more personalized approach to healthcare. In that sense, generative AI is not about replacing but rather about augmentation so that healthcare professionals are able to provide even more personalized care tailored to each patient’s unique needs. This democratizes the process of decision-making between patients and healthcare providers by making vital information such as personalized options for treatment more accessible to patients. This way, patients are empowered to engage more actively in decisions pertaining to their healthcare.
The acknowledgement of AI’s prowess for advancing the healthcare industry need not necessarily shadow valid concerns shared by both patients and healthcare professionals regarding fears of replacement and misuse. This fear is reflected in a Pew Research poll where a majority of American adults feel uncomfortable with the idea of AI diagnosing diseases or recommending treatments. The takeaway is that generative AI facilitates a stronger partnership between patients and healthcare providers through personalized care and shared decision-making. As this technology continues to evolve, it’s important to make sure that the human touch remains central to the healthcare service.
Misconception: Generative AI exacerbates social inequalities on a global scale by being accessible only to the wealthy.
This fear stems from the idea that AI could widen the gap in social, digital, and financial disparities on a global scale. There is evidence of meaningful efforts to explore how generative AI can be beneficial across various societal segments. However, the resource-intensive nature of developing and deploying generative AI tools poses a significant challenge. For example, it becomes the case that organizations with the wherewithal to afford the resources will ultimately dictate conversations when it comes to the advancement and deployment of AI technologies, contributing to widening social inequalities. Nevertheless, companies like Google are taking steps to mitigate this disparity by investing in underdeveloped countries with the goal of democratizing technology access. This initiative is part of a broader movement to develop policies and fund mechanisms that ensure equitable access to AI technologies. Governments are also beginning to recognize the importance of supporting these endeavors.
Yet, challenges remain. Some governments are imposing restrictions on the free flow of information, increasing surveillance, and limiting access to cross-border internet service provision, which places significant roadblocks to equitable access to AI. Overcoming these obstacles requires a concerted effort to hold resource-rich companies accountable, raise awareness, and prioritize actions that address these disparities. By focusing on these efforts, there is hope to prevent generative AI from becoming a catalyst for widening social inequalities and instead prompt its use as a tool for widespread inclusivity.
Misconception: The integration of generative AI in education threatens to devalue the human-dependent teaching and learning experience.
Concerns that AI might replace the essential human element inherent to the teaching and learning experience are prevalent. In parallel, there’s a growing recognition that AI stands to augment rather than replace human educators. For instance, faculty who teach computer science courses at elite institutions have begun to utilize generative AI to offer personalized support and guidance to students, such as to help them identify bugs in their code or improve the design of their computer programs. This approach opens up significant opportunities for educators to shift their classroom time from traditional lectures to more interactive, discussion-based learning. Such interactions foster idea sharing and creativity, potentially enhancing the relationship between students and professors. The perception that AI will dehumanize the teaching and learning experience overlooks the potential of AI to enrich this experience by freeing educators from repetitive tasks and allowing them to engage more deeply with their students. The thoughtful integration of AI in education can facilitate a more dynamic and engaging learning environment, such as by promoting deeper understanding and connections during live classroom discussions.
Misconception: The deployment of AI for military purposes, such as the use of autonomous weapons, takes life and death decisions away from human control.
The ethical concerns surrounding the use of AI in military applications are both intriguing and alarming. There’s a common fear regarding the deployment of AI and autonomous weapons suggestive of a future where military operations might rely heavily on technology devoid of human judgment. However, referring to recent discourse on this topic including a thought piece by Defense One, it becomes clear that the reality is more nuanced and grounded in responsible experimentation and development. For instance, the U.S. Navy and Air Force have been exploring the use of AI in aerial and naval craft, aiming to enhance tactical capabilities and operational efficiency with autonomous systems. These efforts include experiments where unmanned surface vessels and air vehicles communicate with each other to execute missions with impressive accuracy. Such technologies could also play a role in safeguarding international shipping pathways against hostile actors.
Importantly, there is an ongoing effort within the United States to establish international norms for the responsible use of AI and autonomy in military settings. The U.S. Department of Defense has revised its policies to address public concerns about the seemingly ominous direction of the development of AI for military purposes, emphasizing the importance of human oversight and ethical considerations. This revision aims to clarify the U.S.’s commitment to developing and employing AI in a manner that minimizes collateral damage and ensures that human decision-makers remain integral to the operational loop. By prioritizing these aspects, the U.S. seeks to lead by example in fostering responsible development and use of AI for military purposes while at the same time mitigating fears of a dystopian future dominated by deadly robots.
Misconception: Under the guise of ‘personalized advertising’, generative AI poses an inevitable threat of invasion of privacy.
The shift towards using generative AI in advertising has largely been about harnessing its capacity to analyze vast amounts of data, including unstructured data, to predict consumer interests with remarkable accuracy. This capability enhances the creation of highly targeted and customized content, leading to more effective and engaging advertising experiences. This concept isn’t entirely new: personalized advertising based on consumer behavior and preferences has been a staple in marketing for years, but the invention of generative AI elevates the precision and effectiveness of these efforts.
Despite these benefits, the impact of generative AI on consumer privacy is often viewed through a lens of skepticism. The assumption that increased personalization directly translates to invasion of privacy is an oversimplification. In reality, the industry is moving towards implementing advanced data protection measures, ethical AI practices, and transparent consumer data policies. These efforts include anonymizing personal data, employing data minimization techniques, and adhering to strict data protection standards, such as deleting customer data that is no longer in use to prevent potential breaches. These measures emphasize the industry’s commitment to navigating the fine line between personalization and privacy in order to ensure advancements in advertising technology do not come at the expense of consumer trust and safety.
Misconception: Generative AI is poised to completely replace human financial forecasters by providing reliable, efficient, and timely alternatives.
In the tech community, there’s an ongoing debate about generative AI’s capability to enhance the accuracy of financial forecasting, with some believing it might replace human forecasters entirely. This misconception suggests a future where the human trader becomes obsolete due to AI’s superior predictive capabilities. The emergence of Robo-advisors and Robo-investing is a good example. These technologies present a cost-effective option for investors by automating the investment process such as generating portfolios aligned with the user’s risk tolerance and preferences through algorithms. With that in mind, the transition to Robo-investing doesn’t signify the complete removal of human elements from investment decisions. Many financial institutions prefer to employ a hybrid approach where human judgment is integrated with algorithmic recommendations.
The debate about using generative AI tools for prediction raises an important question: whether to trust a human or an algorithm with financial decisions. While algorithms excel in processing vast datasets and executing trades potentially optimized for the long term, human advisors often pursue riskier, potentially more rewarding investment strategies. This debate extends to the role of human emotion and bias in financial decision-making. Some argue that removing the human emotional factor could lead to more rational, unbiased decisions. However, since humans design algorithms, a level of bias is inevitably embedded within them. This ongoing dialogue highlights the complexity of integrating AI into financial practices and the continued value of human oversight and intuition in the investment process.
Misconception: Generative AI perpetuates existing ethical inequities and biases in surveillance and law enforcement.
A consensus could largely be formed on the affordance of generative AI for law enforcement in terms of enhancing surveillance capabilities, from monitoring crowds for anomalies to applying facial recognition technology, and even to predictive policing. These advancements can improve safety measures and legal communication with the public, and promise to herald a new era of efficiency and effectiveness in law enforcement. A notable example includes the ability of AI systems to analyze and predict potential criminal activity that enables proactive approaches to crime prevention.
The enthusiasm for these technologies is tampered by significant ethical concerns, primarily pertaining to bias and privacy. Instances where generative AI-generated sketches inaccurately represent suspects often with a skewed preference of skin color, highlight the risk of generative AI tools in reinforcing biases and potentially leading to wrongful convictions. Additionally, the collection and analysis of surveillance data could infringe on individual privacy by creating profiles that may not accurately reflect one’s actions or intentions. To address these challenges, there’s a pressing need for transparency and accountability in the development and deployment of generative AI tools in law enforcement. Maintaining human involvement is crucial for ensuring that AI supports and not replaces the nuanced judgment required in policing.
This conversation extends beyond law enforcement to broader applications of AI, including in hiring practices where algorithmic screening tools have been found to perpetuate existing biases. This punctuates the importance of balancing efficiency gains with the ethical implications of AI, and advocating for a cautious approach that prioritizes human rights and dignity.
Supplemental Resources
- AI won’t replace humans – but humans with AI will replace humans without AI (HBR article)
- OpenAI’s Q* is alarming for a different reason (Bloomberg article)
- Will teachers listen to feedback from an AI? (EdSurge article)
- AI and its impact on finance in 2022: Bombay Stock Exchange Broker’s Forum (BBF); Forum Views, November 1, 2022
Disclaimer
The “Insights from the Field” initiative is a platform for guest contributors – who are industry leaders, subject-matter experts, and leading academics – to share their expert opinions and valuable perspectives on topics related to the fields of Business, Artificial Intelligence (AI), and Machine Learning (ML). Our guest contributors bring a wealth of knowledge and experiences in their respective fields, and we believe that their insights can significantly enrich our community’s understanding of the dynamic and intertwined spaces of business, technology, and society.
It’s important to note, however, that the Digital, Data, and Design (D^3) Institute does not explicitly endorse opinions expressed by our guest contributors. With this initiative, we hope to facilitate the exchange of diverse perspectives and encourage critical thinking, with an overarching goal of fostering meaningful and informed discussions on topics we consider are important to our community.