Machine Learning in Airline Loyalty Programs
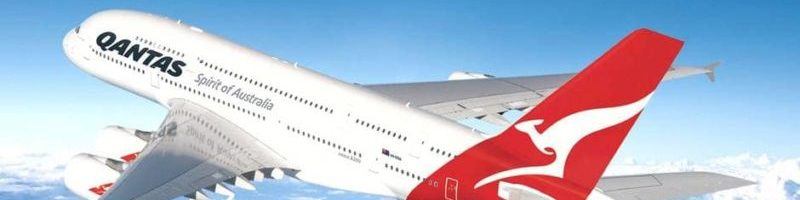
Airline loyalty programs (also called Frequent Flyer Programs) have become a new source of high margin income for traditional airline companies. Meanwhile, loyalty programs also generate massive amount of data on customer behaviour as people spend and collect their points. Applying machine learning tools to understand the preferences of those frequent flyers, most of whom have strong spending power, will enable an airline loyalty program to monetize on areas beyond travel. Qantas Australia is the leader in adopting a data-centric approach to manage their loyalty business.
Commercial aviation is a tough industry to be in. Airlines have been haunted by rising costs, lower margins and by increasing competition from low cost carriers (“LLCs”). American Airlines, for example, recorded net loss for in 11 out of the past 20 years(1). During the remaining profitable years, net margin had been in single digits. However, the emergence of airline loyalty programs has provided a new source of high margin income.
Airline loyalty programs generate most of their income from selling frequent flyer mileages (or “points”) to their coalition partners (i.e. credit card companies) and collected cash upfront from selling those points. Meanwhile, it takes a long time for a customer (i.e. credit card holder) to redeem those points. Some customers may never redeem (or points expired), resulting in 100% margin for the airlines. Higher margins can also be made from redeeming ancillary service awards such as baggage fees, ticketing change fees, extra leg-room seats (i.e. safety exit rows). Take United as an example, When United Airlines filed for bankruptcy in 2002, its mileage program posted profit margins as high as 45%(2).
The time difference between airlines collecting cash upfront and customers redeeming awards created huge benefits, as the upfront cash could allow airlines to invest early into other profitable areas, such as buying new aircrafts that are most cost-efficient.
Loyalty programs enable win-win partnerships among coalition partners. A credit card holder may want to spend more to accumulate points and would prefer to fly the program airline. According to American Express, their partnership with Delta Skymiles will yield $4 billion in revenue per year by 2021, rising by more than $300 million annually. The partnership also accounted for ~7% of American Express’s worldwide billed business in 2016 and was responsible for ~20% of worldwide card-member loans(3).
As loyalty programs can record data on customer behavior, using machine learning to predict customer preferences is the perquisite to generate incremental revenue for both airlines and their coalition partners. Qantas Loyalty is one of the forerunners in building a data-centric loyalty program by leveraging customer insights to offer non-airline products such as insurances and to deliver marketing services to other industry partners. In 1Q 2017 alone, Qantas Loyalty generated A$400m of incremental spending(4).
To accelerate build-up of machine learning capabilities, Qantas acquired Data Republic and Taylor Fry analytics. In addition to extracting customer insights from existing data, Qantas is launching its own-Qantas branded credit cards. In the next few years the loyalty program will be able to understand customers’ spending habits outside of travel through the branded credit card transactions.
In the long-term, Qantas plans to transform its loyalty program into a marketing platform that could serve not only the airlines but also all coalition partners. Frequent flyers are usually those with deep pockets. Qantas loyalty will use machine learning tools to understand the preferences of those customers and help its coalition partners to better market their services. For instance, Jason usually redeems a ticket from NY to San Francisco once he accumulates 15,000 points. After arrival he would rent a car for at least two days. With such insights, the loyalty program could provide targeted advertisement for car rental companies when Jason’s loyalty account is hitting the 15,000 threshold.
Transforming a loyalty program into a data-driven business requires a different set of managerial expertise. As a result, separating the loyalty business unit and bringing out-side partners or investors with machine learning expertise could be particularly helpful. Virgin Australia, the 2nd largest airline in Australia, separated their Virgin Velocity loyalty business and invited Affinity Partners, a private equity company, to own a significant minority stake in Virgin Velocity in 2014. Affinity Partners has since helped Velocity acquire data analytics firms and brought in management talents to propel Velocity’s expansion.
Qantas also needs to streamline the process of award redemption. Building a robust customer behavior database requires customers so use their points frequently. A Smooth redemption process is necessary to make points a desirable “currency”. The more people are willing to use and collect the points, the more coalition partners will be incentivized to join the loyalty platform. For example, when Jason arrives in San Francisco, he then spends in a wine store and at a local restaurant, both businesses are coalition partners. Machine learning can then understand the type of food and wine Jason enjoys, and help the wine store and the restaurant attract more members in the loyalty program like Jason.
However, maximizing the benefits of machine learning requires ALL coalition partners to share their customer data with the airline. Why would American Express share their transaction data if the data is so valuable? What would be the right mechanism to encourage platform-wide data sharing?
(Total word count: 782)
Quotations:
[1] Capital IQ, data compiled from company filings
[2] Hugo Martin, “Frequent flier programs generate profits for airlines and frustration for travelers”, Los Angeles Times, Sep 15, 2017, http://www.latimes.com/business/la-fi-frequent-flier-programs-20170914-story.html
[3] Jennifer Surane, “JPMorgan Sweetens United Card Rewards After Airline’s Complaints”, Bloomberg, June 1, 2018, https://www.bloomberg.com/news/articles/2018-06-01/jpmorgan-sweetens-united-card-rewards-after-airline-s-complaints
[4] Qantas Australia 2017 Annual Report, Investor presentations, Qantas Group, Annual Report as of Dec 31, 2017, https://investor.qantas.com/investors/?page=annual-reports
Interesting article! I agree that encouraging platform-wide data sharing will be extremely tricky to accomplish given many companies finding significant value and privacy in their transaction data. However, I think a larger question that is not to be overlooked is whether we, the customer, would want such platform-wide data sharing? In theory, such data sharing could become so large that it would track a majority of steps we take (traveling or not) such that we are guided by the coalition partners to make certain choices through advertising and similar. In considering whether to encourage or allow such platforms we must first understand the limitations this puts on our privacy and our decision making processes, as the latter would be less free or independent than we would like to think they are.
It’s fascinating to see that Qantas has made acquisitions in the field of data science. Beyond detecting spending patterns of individuals, and using those to later target the same individual, how is Qantas using machine learning to make predictions about their entire customer base? What features will they identify as most critical to their models? A regular business traveler expensing all costs will exhibit very different behavior from a sporadic-leisure traveler who signed up during a loyalty promotion. In order to build useful predictive models, Qantas will need sufficient data from all customer segments. For this reason, I wonder if they are pursuing look-alike modeling, where they generalize characteristics of a customer’s behavior to increase the relevant targeted audience; for example, Jane traveling from LA to DC may be surfaced a rental car ad because of Jason’s prior purchasing behavior, if Jane is deemed to “look like” Jason. I expect that key features to predictive models at Qantas will be length-of-travel, weekday of travel (as a proxy for business or leisure), average airfare, fare class, and frequented cities. Will Qantas be able to use patterns from their loyalty program to target new visitors to their site?