DeliveryHero: Managing over 150,000 restaurants in 40 countries using machine learning
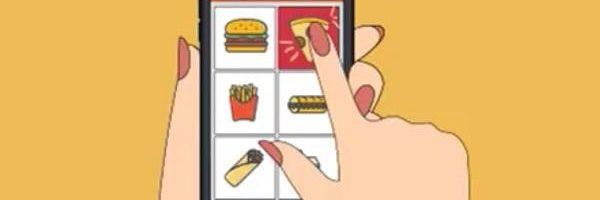
Managing sales and account management teams using data and machine learning
Online food ordering has been growing extremely fast in the last years with many players entering the market and some others establishing themselves as market leaders in the respective regions. Some of bigger companies/holdings are Grubhub, DeliveryHero and JustEat. [1]
Most of them operate a marketplace model, meaning they don’t have to deal with the last mile delivery of food. Marketplace sounds like an easy way to have a scalable business without the complexity inventory can cause, but that comes with its own challenges.
- How do you manage over 150,000 restaurants across 40 countries when you are not directly responsible for their quality or delivery time but you are directly penalized by the customer for recommending them a bad restaurant?
- How do you decide which restaurant should the sales people go after and in which areas?
- How do you know which restaurants have the biggest potential to grow so you can support them with a dedicated account manager?
- How do you identify restaurants that are just churning your customers and therefore you would rather no longer list them on the platform?
The restaurant acquisition challenge:
There are multiple factors to consider when creating a lead list for the sales team to go after such as: How many and what type of restaurants are available on a specific area, what is the rating of the restaurant or whether the restaurant is available for delivery at a competitor’s website. This process is complex and can have a big impact on costs (sales bonuses) and customer experience.
To improve this process, Deliveryhero is using Machine learning that helps combine data from a variety of sources and create a lead list for the sales team with target restaurants to sign. Leads are graded based on the potential they have to generate incremental orders. To grade leads, some of the sources used are: previous sales history, google reviews, social media, competitor listing pages and performance of a specific cuisine and restaurant type that specific area. The algorithm runs every two months and updates the lead list with new leads as well as updates the grade for the existing leads as conditions may have changed and therefore a lead graded as “Α” quality before might not be not as relevant now.
Thoughts non addressed points:
Time needed to sign a new restaurant varies depending on how big a restaurant is (ie. a big chain requires significantly more time compared to a family owned restaurant). There are also a lot of synergies with regards to to the signing process that can occur (ie. a sales representative has signed the same restaurant in another country or area, therefore combining this effort can have higher chances of success). This information can be found online and also in the internal sales management software. Adding this info in the grade algorithm can increase significantly the sign up success rate and also help identify synergies in a global organisation that are often difficult to spot.
The restaurant management challenge:
Once a restaurant is online, it is important to ensure it is performing well and drives customer satisfaction. For a company with over 150,000 restaurants, assigning an account manager to each restaurant is not economically sustainable and therefore it is important to identify which restaurants should get one and which should be managed in a more “automated” way. Also actions within these groups can vary. For example restaurants that have high order volume but low operational performance should have a different recommended action plan compared to those that have great operational performance but have not yet reached their full order potential or restaurants that are doing great in both areas but have a low margin for the company.
Machine Learning algorithms are used in this areas of the business too to understand what drives restaurant performance and customer satisfaction and produce action plans for each restaurant. Some of the recommendation can be about improve operational performance, increase commission, run a free delivery deal or improve menu assortment.
Thoughts non addressed points:
The biggest challenge when implementing this algorithm is identifying business impact. Increasing the commission of a restaurant has a clear impact on Revenues but how about improving the order rejection rate? Though rejection rate is highly correlated to customer satisfaction it is challenging to understand what is the direct business impact. Usage of data mining to understand this better would be very helpful for business leaders to focus on the right priorities for their teams.
Open question:
Machine learning algorithms are essential in identifying important tasks for the sales team but how happy would sales employees be to work for a company that doesn’t take into consideration their personal judgment regarding their area of expertise?
(word count: 786)
Sources:
[1] https://thespoon.tech/3-5-billion-invested-in-food-delivery-startups-this-year/
You did a great job of clearly articulating the challenges to each stakeholder in the process. Additionally, I really appreciated the use of questions and examples throughout. I would be curious about what you think the challenges are over the next year or so? And what you think the challenges are longer term?
What an interesting read! I agree with you about the challenge of empowering sales employees when data and algorithms are driving the decision-making. There needs to be a delicate balance of data and intuition, applied together. I think that it’s important for companies to establish guidelines on certain instances when the latter can override the former.
Awesome post! Your essay, and in particular your open question at the end, led me to think about the use of machine learning as a spectrum. Does using machine learning to identify leads mean the algorithm has to output exactly the list of restaurants Deliveryhero would go after? What kind of process could Deliveryhero put in place that would allow the machine learning algorithm to supplement the work of their employees, but not completely eliminate it if it’s not additive to the business?
Great article @EmperorOfJapan! One additional area that the article/algorithms mentioned do not identity is the ability of improving the experience of a customer by providing them food options that they are “most likely to enjoy”. This can definitely be done by combining multiple data sets (e.g., typical target customers for restaurant, customers background/order pattern, etc.).
Thank you for sharing these great thoughts!
Your post made me re-think the delivery ordering experience. It sounds like DeliveryHero’s use of machine learning will make its sales employees’ lives easier, and allow them to allocate their time more efficiently. However, I wonder if the machine learning algorithms would be able to identify new-concept restaurants that don’t fit previous trends for an area. The oversight could result in another delivery service signing the restaurant first, and a loss of future revenue for DeliveryHero. I think that human intuition and judgment could still be relevant and necessary in such cases, and DeliveryHero should make sure that their sales reps don’t stop acting on these kinds of intuition.
Thanks for an interesting article! Different from most of papers or articles are about how to use machine learning as an engineer or the leader of the company, it is really interesting to think about the issue as a sales manager of food delivery services that has valued the insight and knowledge of people on what food/restaurant consumers might love. I don’t think machine learning algorithms would make sales employees demotivated about their roles. Of course, some of AI technologies would replace sales employees, but still, ‘Food’ is strongly linked with each person’s preference. Sales team can use their time on analyzing and finding the opportunities for hidden charms in local area and delivering the best message to its customers. It is up to the company’s decision on how to use just the ‘right’ level of machine learning technology on the context of the business.