Broad use of Machine Learning at Ford
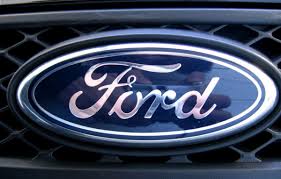
Players in the automotive industry are getting prepared for major disruptions to come in the next 10 to 15 years. Urban mobility (car/ride/bike sharing) and autonomous vehicles1 are disruptive trends that heavily rely on machine learning.
A concern for traditional OEMs (Original Equipment Manufacturer) is how to acquire capabilities that will enable them to maintain their relevance in this new competitive scenario. Tech companies are important newcomers in the industry, being the first ones to start product developments, such as Google autonomous vehicle initiative in 2005. In the mobility market, the asset light, fast to scale business model reduced the barriers to entry and put even more pressure on traditional manufacturers. In response to the new competitive players, OEMs are following two paths to acquire AI (Artificial Intelligence) skills: partnering with software companies (Ford invested $1 bi in Argo AI in 20172) and developing internal capabilities.
Taking a step back from the sole focus on the automotive megatrends, Ford decided to spread the use of big data and machine learning throughout the company. Established in 20153, Ford Global Data Insights and Analytics (GDI&A) is a department inside Ford that works for the business partners to drive evidence-based decision-making both in process improvement and product development4. Regarding process improvement, the GDI&A works on:
- Manufacturing and supply chain – applying advanced analytics to initiatives in scheduling, logistics, supplier health and manufacturing.
- Credit – develops analytical methods to offer, service, and fund loans to consumers and business .
In terms of product development, the GDI&A works on:
- Product and sustainability – leverage cutting-edge machine learning, optimization, and simulation methods to drive product development and sustainability initiatives.
- Business and sales planning – developing analytical methods to forecast and understand market response to vehicle and technology offerings.
- Customer – develops and integrates a wide range of market and consumer analytic workstreams.
Machine learning can be very useful for corporations that have access to large amount of processes and product development data. However, one main challenge to the widespread use of machine learning in the short term is that the data needs to be aggregated and organized. Otherwise, the results of machine learning analyses might be biased in an incomprehensible way or lead to an unintended conclusion. Due to the lack of big data sophisticated tools until recently, many companies still have to aggregate and structure large databases that are suitable for machine learning analyses. At Ford, the GDI&A plays an important role in the preparation for machine learning usage. By working on data analytics projects across the organization, the GDI&A team not only delivers insights that are readily applicable to process improvements or product development, but also lays the ground for future machine learning projects by having the data sorted.
An example of a process improvement project that GDI&A has engaged on, with support of Rutgers School of Engineering, is the improvement of the Helium Leak Test, a critical process in the transmission manufacturing. By developing methods to detect trends in the manufacturing data based on predictable machine failure patterns and root causes involved, the team could predict when failures are about to happen and then get repair in a timely manner. This project used a large array of manufacturing data from Ford production around the world, including cycle time, throughput, warnings, faults, maintenance, shift notes, product quality and more.5
Another example of process improvement is the recently conducted study about the use of machine learning to make a more in-depth analysis of a person’s likelihood of paying back a loan. Ford Credit Chairman and CEO Joy Falotico said in a statement. “The study showed improved predictive power, which holds promise for more approvals, enhanced customer experiences and even stronger business performance, including lower credit losses.”6
Establishing a department that creates insights for the whole organization using data analytics and machine learning tools was a great move from Ford management. One question is how to expand this department globally? Solving this challenge can unlock huge potential in developing countries, where big data and machine learning usage are far more limited than in developed countries.
Coming back to the autonomous vehicles megatrend, a main concern not only to Ford, but also to all OEMs is the competition of tech companies. How should manufacturing companies face the competition scenario regarding software development? Is in-house development or partnership with tech companies the best approach? (726 words)
Sources:
1 Roland Berger. Automotive disruption. https://www.rolandberger.com/en/Insights/Global-Topics/Automotive-Disruption/, accessed November 2018
2 New Atlas. “Ford bets big on artificial intelligence for autonomy”. https://newatlas.com/ford-self-driving-ai-investment/47887/, accessed November 2018
3 ZD Net. “How Ford democratizes the use of data throughout the enterprise”. https://www.zdnet.com/article/how-ford-democratizes-the-use-of-data-throughout-the-enterprise/, accessed November 2018
4 Ford. Ford Global Data Insights and Analytics (GDI&A). https://corporate.ford.com/careers/departments/global-data-insight-analytics.html, accessed November 2018
5 Industrial & Systems Engineering at Rudgers. Newsletter Fall 2018. https://ise.rutgers.edu/sites/default/files/uploads/ise-newsletter_fall2018_web.pdf, accessed November 2018
6 Investor Place. “Ford To Use Machine Learning For Credit Approvals”. https://investorplace.com/2017/08/ford-credit-scores-f/, accessed November 2018
Really great post! It’s very interesting to think about how large, legacy companies like Ford can use machine learning to stay competitive in a changing landscape and world. Currently, given the uncertainty with machine learning to date, I think that Ford is best to partner with existing companies, rather than developing software solutions in-house. Over time, as their business model may shift due to the changing transportation landscape, it may make more sense for them to invest in an in-house solution.
Data scientists are now a scarce resource in the job market. To acquire enough to build out a sophisticated AI technology team might be a lofty goal. I believe partnership with AI specialists may be the best route for Ford to take. Many car companies have now embedded similar (though not identical) operating software systems into their cars. This makes data aggregation, standardization and analysis slightly easier. However, specialist skills still need to be applied to this data in order to be able to extract the most important insights. This capability is relatively commoditized nowadays with the myriad of AI start ups that have been founded. Ford should partner closely with a few of these companies to obtain the insights, and invest its own resources into areas it knows best – embedding these insights to make cars better.
Excellent read about Ford’s GDI&A team! I would lean towards partnering given the immediate benefits that Ford can reap from such a relationship. With the complexity required to make sense of so much data and ensure that it isn’t littering with biases that lead to skewed conclusions, I believe tech companies would be much better suited to take on this role.