Zone7: Future of AI in Football (Soccer)
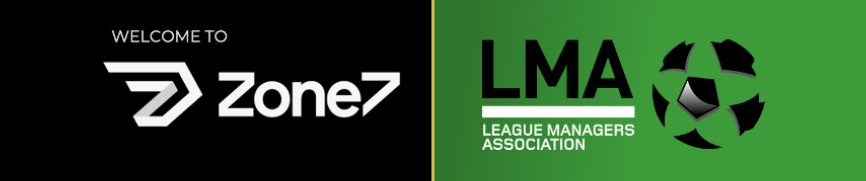
Injuries in high-performance teams can be very expensive for management. Zone7 seems to have the solution
Context
Injuries are a huge concern of high-performance football players and their clubs. Although many injuries cannot be foreseen (e.g., a bad fall in a game) there are some types of injuries that could be prevented if teams had the ability to project the risk based on a varied set of data.
This is where Zone7 comes in. Its system offers daily injury risk forecasting based on available data sets and AI-driven technology.
To evaluate its algorithm and create its value proposition, the company has performed tests with 11 different professional football teams that compete in Europe and North America. The primary focus of this type of A/B testing was to demonstrate the company’s ability to forecast the risk of injury and injury type and compare it to the actual injury incidences.
How does this work?
- Each team was required to have data for at least one whole competitive season between 2019 and 2021.
- Data inputs to the model considered workload (e.g., training and games), historical injury records per player, age of the players, and their competition schedule amongst other variables.
- The database focused on injuries sustained during the team’s in-season timeframe, including pre-season. It excluded goalkeepers from the analysis and any non-sport related injury/illness
- Specific models were created for each team considering their dataset came from different systems and technology providers
- Workload analysis was performed by Zone7 and it generated an injury risk forecast.
- The company’s algorithm was not trained on any clubs before this data analysis and forecast test
- The AI algorithm identifies risk patterns and forecasts risk injury for the following 7 days and would be updated every day with workload data and other data.
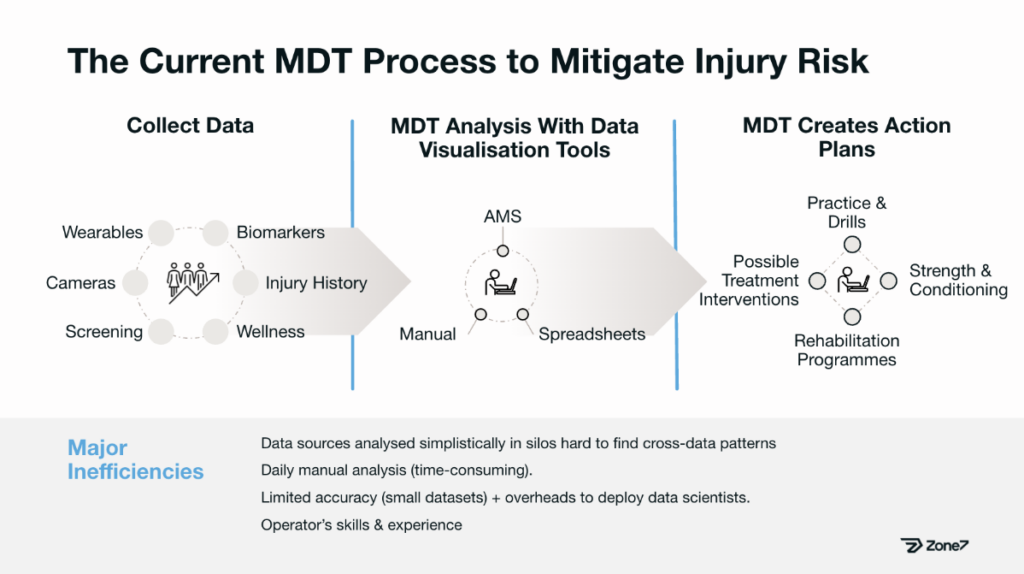
What did the company find?
A total of 423 injuries were suffered across the 11 teams, and Zone7 forecasted increased risk prior to the occurrence of 306 of these injuries (72% accuracy). 56% of these injuries were categorized as high risk and 16% as medium risk.
Although there was some variance in the accuracy across the 11 teams (4%) the system performed consistently across the different environments. The company also included an injury-type projection, but due to data size and variability, the results were interesting but not statistically significant.
Opportunities
In my opinion, this technology has huge opportunities not only in football. Having an injured player in a high-performance team does not only mean it’s a big psychological challenge for the player but it’s also highly costly for the management team. The team must keep paying huge amounts of money due to their contract and at the same time, allocate resources to take care of the players’ recovery through physical therapy and surgery in some cases. There are clear monetary benefits to installing such technology in high-performing teams and football has been implementing so much technology that it is now a very rich data space, especially in high-competing leagues like in Europe. There are other positive externalities to implementing such technology, for example, there could be higher engagement from players that feel taken care of and consistency in their in-game strategy since you can count on your most crucial players.
Challenges
I can think of two main challenges up until now.
- Monetization and Value Capture: Similar to the “Intenseye” case, the fact that this technology’s monetary and intrinsic benefits are hard to quantify because it’s a “preventative” technology makes it hard to put a price on it. Also, the range of teams/sports and geographies this could be implemented on means there is a very different willingness to pay and different priorities for Zone7 depending on whom they want to target.
- Scalability: The fact that all teams use different platforms and are at different levels of technology implementations means that this system might not be quite as scalable outside the high-performing, deep-pocketed teams.
- Copycats: Some deep-pocketed teams might want to build this technology in-house since hiring Zone7 would mean sharing data about their players and training.
Main Thoughts…
From its inception to now, Zone7 has worked with more than 50 teas in various leagues in North America and Europe, even the Premiership Rugby.
Even though the company has huge challenges ahead, this technology could change the landscape of injuries in high-performance teams. The company still has a long way to go in terms of its go-to-market strategy, but they have recently secured $8 million in Series A funding to expand its reach in sports.
This company is a clear case of separating AI prediction from human judgment since Football, and most sports, come with an inherent risk of injury, but at the time of a decision, there is a thin balance between being risk-averse and risk-aware vs. performance requirements.
Sources:
- https://www.forbes.com/sites/timcasey/2021/07/22/zone7-raises-8-million-in-series-a-round-to-expand-artificial-intelligence-platform-for-predicting-sports-injury-risks/?sh=26ebf17d255f
- https://zone7.ai/validation-study-injury-risk-forecasting-with-zone7-ai/
- https://www.wsj.com/articles/how-ai-could-revolutionize-sports-trainers-tap-algorithms-to-boost-performance-prevent-injury-11654353916
This is fascinating! (and timely for the World Cup!) I find your discussion of the 3 challenges particularly illuminating. The company certainly needs to scale to keep growing but as you said, it is challenging to make its service compatible with different record-tracking systems at different teams (or even outside of soccer). Very curious to see how the company does in the near future!
That’s an exciting use case of analytics in in sports! Just recently I attended a meeting with the Harvard Sports analytics association where we had the chief data of Manchester City as a guest speaker. It was super interesting! However, he was mainly focusing about the valuation and early scouting of youth players. We discussed that compared to baseball or american football, soccer just has a few more unknown variables, which has so far prevented the adoption of data analytics at the scale we are used to in the US. But I absolutely agree that by evaluating biological data of soccer players, the load in training and competition can be controlled much better and thus injuries can be better prevented. However, I have some doubts in the data set at this time. Since the reference data has been collected between 2019 and 2021, several games took place without spectators. On an athlete’s level, this takes away a certain amount of stress, as the perceived mental pressure in the stadium is not quite the same (for a penalty kick for example the heart rate is way lower). Therefore, it could be that the model is possibly somewhat biased for the time after.
Super exciting use case, thank you!