Will IBM’s Deep Thunder Finally be the Key to Accurate Weather Forecasting?
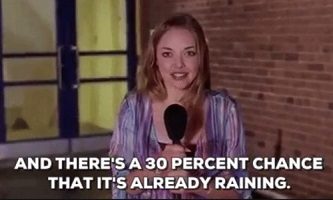
Traditionally, government organizations (NOAA) and private companies (the Weather Company) have collected massive amounts of data via satellites and sensors, but weather forecasting models have had mixed success. IBM’s purchase of TWC in 2016 combines this data with a refined model to provide a hyper-local forecasting system that could revolutionize natural disaster preparation efforts globally.
Limitations of Forecasting Capabilities – Data is NOT the Problem: Bill Belichick, head coach of the New England Patriots, summed up the national sentiment towards weather forecasters well when he stated “if I did my job the way they did theirs, I’d be here about a week.”[1] While the historical error rate has been somewhat overstated (2013 study showed the top three weather providers were ~82% accurate in predicting next day precipitation)[1], the remaining gap doesn’t come from a lack of data. Currently there are ~1,000 weather satellites in space and “hundreds of thousands of government and private weather stations” on the ground, all capturing data on temperatures, wind speeds, cloud patterns, etc.[2] The National Oceanic and Atmospheric Administration (NOAA) collects this data and feeds it into its own public models (Global Forecast System and Numerical Weather Prediction) and makes it available for use of private companies such as The Weather Company (TWC).3 If all this data is available, why are forecasts still inaccurate?
One reason is that the NOAA and TWC haven’t been able to build models to the level of specificity needed for short-term weather prediction. In the case of the NOAA, efforts have been broadly focused on high-impact events such as thunderstorms, tornados, and hurricanes. While the NOAA has begun to use machine learning techniques to fuse model output with greater observation capabilities (storm duration, wind speed, precipitation and aviation turbulence),[4] these models still fail to deliver accurate information at the localized level.[5]
Figure 1: Global Forecast System (GFS) and Numerical Weather Prediction (NWP) Output, www.ncdc.noaa.gov
Figure 2: The Weather Company Interactive Radar Map, www.weather.com
Local Forecasting via IBM’s Deep Thunder: In 1996, IBM attempted to tackle weather forecasting at the ‘hyper-local’ level, aiming to solve for variation within given regions.[6] The theory behind the project, dubbed “Deep Thunder,” was that local surface features (i.e. hilly terrain, bodies of water, urban design, etc.) were not accounted for in NOAA’s data measurement/analysis, but lead to significant variance in measurable weather metrics within small geographic areas.[6] In many cases, this level of granularity is required: for example, Belichick would not care about weather across his county, only the radius around Foxboro Stadium. Similarly, utilities companies don’t care about precipitation across an entire city, just the localized wind speeds (and maximum gusts) that would result in power outages. IBM felt uniquely positioned to create this model given public access to NOAA data and its existing AI platform.
However, Deep Thunder didn’t gain momentum until recently when IBM purchased TWC and gained access to its existing models/private data centers, which in tandem with NOAA account for ~100 terabytes per day.[2] While in the short-term Deep Thunder adapts new daily data to refine its local models (currently as narrow as ~1/5th mile resolution)[7], the company is also rapidly expanding its database to build for the medium-to-long term. Through Weather Underground, a subsidiary of TWC, Deep Thunder now has access to a network of +250,000 personal weather stations (units on consumer homes, commercial buildings, etc.) that feed back into the centralized database.[8] This data is presented to consumers via the weather apps, including the Deep Thunder app, which is pay-for-service to city/state governments and utilities companies (limited monetization to-date).
Figure 3: Cross Sections of Deep Thunder Model, public.dhe.ibm.com
Figure 4: Personal Weather System Heat Map & Illustrative Example, www.weatherunderground.com
Where Should Deep Thunder Go Next? In terms of short-term goals, Deep Thunder should continue to focus on expanding its network of personal weather stations by i) incentivizing individuals in remote areas to install units, potentially by subsidizing costs in exchange for ongoing Weather Underground access, and ii) exploring new ways of increasing touchpoints. For example, barometric pressure sensors have been built into smartphones since 2011 for location services; as of 2016 Deep Thunder was tapping into ~75k reports per hour, but estimated this could increase to “over a billion per day.”[9] GE has also begun implementing “smart streetlights” in MSAs that measure humidity, light and air quality, In terms of the medium-to-long term, IBM should more formally partner with NOAA to facilitate better transfer of data/existing models and AI methodologies. As NOAA tackles the impact of severe weather systems from the top-down and IBM tackles daily weather from the bottom-up, the ideal outcome would be a system that can predict severe systems at a hyper-local level.
As Deep Thunder is incorporating new data, it’s imperative to always consider new observable characteristics that might impact weather patterns. Some upgrades on the horizon include i) updated snow coverage, ii) internal/external solar radiation, and iii) real-time updated vegetation database.
Figure 5: Initial Deep Thunder Hurricane Mapping, ibm.com/waston
Further Impact?
- Monetization: In what ways can IBM monetize its forecasting ability beyond its current platform (ad revenue, pay-for-service to consumer, sell to city planners/utility providers, etc.)?
- Other Natural Disasters? While the system is currently equipped to predict the impact of weather systems, and will ultimately target severe storm systems, is there a way for this methodology to link with other AI systems that exist to better predict natural disasters such as forest fires, earthquakes, etc.?[10]
Word Count (798)
Endnotes:
1Samenow, Jason and Fritz, Angela. “Five myths about weather forecasting.” The Washington Post, January 2, 2015. https://www.washingtonpost.com/opinions/five-myths-about-weather-forecasting/2015/01/02/e49e8950-8b86-11e4-a085-34e9b9f09a58_story.html?noredirect=on&utm_term=.436a9eb4ccda accessed November 2018
2Walker, John. “AI for Weather Forecasting – In Retail, Agriculture, Disaster Prediction, and More.” Tech Emergence, October 7, 2017. https://www.techemergence.com/ai-for-weather-forecasting/ accessed November 2018
3National Oceanic and Atmospheric Administration, “Numerical Weather Prediction” and “Global Forecast System” https://www.ncdc.noaa.gov/data-access/model-data/model-datasets/numerical-weather-prediction accessed November 2018
4McGovern, Amy. “Using Artificial Intelligence to Improve Real-Time Decision-Making for High-Impact Weather.” American Meteorological Society (2017). ABI/INFORM via ProQuest, accessed November 2018
5Pierre-Louis, Kendra. “Hurricane Florene: Your Forecasting and Climate Questions Answered.” The New York Times, September 12, 2018. https://www.nytimes.com/2018/09/12/climate/hurricane-florence-forecast-science.html accessed November 2018
6Gallagher, Sean. “How IBM’s Deep Thunder delivers “hyper-local” forecasts 3-1/2 days out.” Ars Technica, March 14, 2012. https://arstechnica.com/information-technology/2012/03/how-ibms-deep-thunder-delivers-hyper-local-forecasts-3-12-days-out/ accessed November 2018
7Joshi, Naveen. “Transforming weather forecast with AI.” Allerin, August 25, 2018. https://www.allerin.com/blog/transforming-weather-forecast-with-ai accessed November 2018
8Weather Underground, “About Our Data.” https://www.wunderground.com/about/data accessed November 2018
9Waddell, Kaveh. “How Phones Can Help Predict Thunderstorms.” The Atlantic, August 11, 2016, https://www.theatlantic.com/technology/archive/2016/08/how-phones-can-help-predict-thunderstorms/495389/ accessed November 2018
10Fuller, Thomas and Metz, Cade. A.I. Is Helping Scientists Predict When and Where the Next Big Earthquake Will Be.” The New York Times, October 26, 2018. https://www.nytimes.com/2018/10/26/technology/earthquake-predictions-artificial-intelligence.html accessed November 2018
Other Sources:
“Why weather forecasts are so often wrong.” The Economist, June 19, 2016. https://www.economist.com/the-economist-explains/2016/06/19/why-weather-forecasts-are-so-often-wrong accessed November 20
Wilson, S. Sachdev, and A. Alter. “How companies are using machine learning to get faster and more efficient.” Harvard Business Review Digital Articles (May 3, 2016).
Brynjolfsson and A. McAfee. “What’s driving the machine learning explosion?” Harvard Business Review Digital Articles (July 18, 2017).
First, Gossip Girl, I have to thank you for your excellent use of an image of Karen Smith in this post.
I have an idea for monetization: I think that IBM should market Deep Thunder to airlines and airports, which lose a lot of money (many millions of dollars, I assume) each year by canceling flights that could have departed; some of these cancelations are made because of inaccurate or insufficiently localized weather data. On the other hand, if the weather is likely to be too inclement for flights to depart, the earlier the airline can cancel the flight, the easier it is for customers to make alternative arrangements. So in both directions, the more accurate the available information, the better. I would also think about ways to market the product to other businesses that have to make operating decisions based on weather at the last minute (e.g., amusement parks, indoor/outdoor concert or wedding venues).
Great article! My main concern for the Deep Thunder solution, in addition to revenue-generation opportunities, is the feasibility of scaling. The fact that individuals in remote areas will have to be incentivized to install units means that the financial cost of scaling the network across the globe could quickly become prohibitive. With that in mind, the pricing considerations for future monetization efforts might not be economically feasible in terms of willing and able demand for the product, given its incremental benefits over current predictive solutions.
Great article !! Also, love the title and name, very creative.
My concern is similar to Dan’s: what incentive will you provide to individuals to help scale the network. For me, one key challenge is the go-to market and how they can maintain this network once built.
Thank you for this.