WAKE COUNTY USING MACHINE LEARNING FOR PROPERTY APPRAISAL
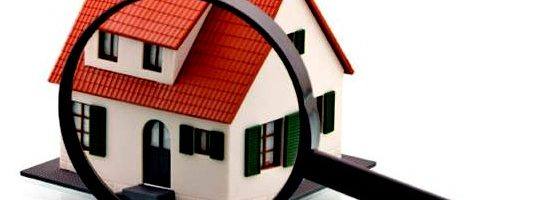
Wake County in North Carolina has implemented machine learning to improve the property value assessment process, something extremely important for tax purposes. The new assessments will be effective in 2020 and will be the result of a new process that intends to get more objective, timely, accurate, thoughtful, and equitable property valuations that provide more confidence to both the County and the taxpayers.
With its population having more than tripled since the 1980s, Wake County in North Carolina, home of the state capital, has seen a significant growth in its real estate activity. The transition from a largely rural area into an increasingly populated district, brought many challenges, many of them related to the properties’ appraisal, something essential for tax purposes. [1] Up until last period, the assessments were performed by only 16 county assessors, who evaluated more than 20 variables for each of the 400,000 properties. [2] This highly manual job was presenting many challenges that needed to be tackled. On the one hand, the staff was not enough to attend the increasing activity and the new policies on appraisal frequency, being extremely challenging to hire and train new appraisers in a reasonable timeframe. On the other, the process was subject to biases or mistakes because it mostly relied on the judgement of the appraisers, who according to Marcus Kinrade, Wake County’s Revenue Director, may “tend to rely more on their experience rather than what the data may be saying”. [1]
Pursuing higher accuracy and fairness, Wake County introduced machine learning (ML) to improve the property value assessment process. The platform relies on ML models that take into account more than 100 variables, such as location, market activity, size, or property exterior, and learns/becomes more “intelligent” with every new transaction. This system addresses many of the abovementioned issues, including completing the assessments in half of the historical time (4 years versus 8), or getting more objective and uniform valuations. [3] Now the employees can enhance their judgement by comparing the properties’ assessment with the system report, which includes the property evaluation and comparable values in similar neighborhoods, enabling them to spend more time in value-added activities, such as understanding and analyzing changes. The overall output is a more objective, timely, accurate, thoughtful, and equitable property valuation, providing more confidence to both the County and the taxpayers.
The new appraisals will become effective in 2020. [3] Nevertheless, to fully untap the system potential, the County will require several years and additional employees. Kinrade expressed that the County’s goal is to continue increasing its “productivity by automating repetitive tasks and increase our accuracy through quality data analysis, sound decision making and the elimination of errors. We try to benefit our Wake County taxpayers by offering quality, accurate services for the lowest cost of operation possible”. [1] In this line, he explained that this project does not address the “tax rate side of the equation” [1], suggesting that longer term plans of the County may include ML to tackle issues such as tax rate determination, and improvement in the allocation of the budget towards the services that the Wake County’s residents value the most.
There are still recurring activities that this system does not address, which would be worth it to evaluate to continue tackling the challenges related to time/resources constraints and confidence of the assessments. In the long term, processing and evaluating the homeowners’ appeals on the assessed values [2] is a task that the system could eventually perform, if the County manages to further standardize the appealing process. Developing a module that can learn from the historic appeals and its resolutions, to then evaluate future complaints, can lead to appraisers spending less time on cases which the system has a high degree of confidence are not applicable, and more time in analyzing those that it predicts can lead to corrective actions from the County. In the short term, an intermediate task ML can improve is the processing of the appeals’ supporting documents and its classification by relevance, learning patterns from every new case. Many legal buffets are already using this kind of technologies when reviewing cases and performing due diligence. [4] Similarly, a professor involved in a study of the National Bureau of Economic Research on algorithms to support the justice system, claimed that ML can be very applicable even in the legal context “where there’s considerable human expertise being brought to bear”. [5]
Making municipalities more efficient and smarter by ML applications is not only good in terms of better resource allocation but also desirable in terms of equality among taxpayers. However, there are some open questions on which Wake County and other organizations going through similar processes, should still work on. The main one relates to how to make sure that the employees are sufficiently trained and understand the basis of the algorithms behind the predictions. The output of this new process will impact not only the local government budget, but the pockets of hundreds of thousands of residents. If the applications of ML are extended to work on resident’s preferences to better allocate the County’s budget, other open questions may include how to make sure that this sensitive information is used only for the intended purposes.
(Word count: 796)
[1] Rick Smith, “Wake County tax team enlists high-tech SAS help to tackle property assessments”, WRAL TechWire, August 20, 2018 – https://www.wraltechwire.com/2018/08/20/wake-county-tax-team-enlists-high-tech-sas-help-to-tackle-property-assessments/
[2] Colin Wood, “Machine learning is helping this North Carolina county keep up on property assessments”, Statescoop, September 4, 2018 – https://statescoop.com/machine-learning-is-helping-this-north-carolina-county-keep-up-on-property-assessments/
[3] Artificial intelligence improves assessment accuracy and productivity in Wake County, SAS Corporate Website, Customer Stories – https://www.sas.com/en_us/customers/wake-county.html
[4] Bernard Marr, “How AI And Machine Learning Are Transforming Law Firms And The Legal Sector”, Forbes, May 23, 2018 – https://www.forbes.com/sites/bernardmarr/2018/05/23/how-ai-and-machine-learning-are-transforming-law-firms-and-the-legal-sector/#57f18b5832c3
[5] Tom Simonite, “How to Upgrade Judges with Machine Learning”, MIT Technology Review, March 6, 2017 – https://www.technologyreview.com/s/603763/how-to-upgrade-judges-with-machine-learning/
I find the idea of using machine learning to evaluate the fair value of real state fascinating! The impact for the local governments and the tax payers can be huge and very positive, as stated in the article. I understand they still have a some refinement work to do on the model, but I wonder if we could extrapolate the use of this technology to help preventing events such as the 2008 housing market crisis for example. Back them, if there was an trustworthy algorithm indicating that there was a bubble and that the houses were actually overpriced, it could have been easier for the investors to understand how the mortgages were overvalued and that a market crash was imminent.
Thanks for a fantastic look into a creative application of machine learning in the public sphere. I grew up in Wake County and am very interested to see how this unfolds in the Research Triangle Park area. The program makes a lot of sense—having the system continuously update with verified home sale prices and refining the model accordingly. A few concerns I would have with its implementation are:
1) Will auditors put in enough data to build a precise model by 2020? Considering that this initiative may displace them in the long run, it seems the incentives are not aligned to build the machine learning capabilities in an efficient way
2) How will the market adjust to the new pricing tool? Considering this new valuation mechanism may reduce or eliminate inefficiencies in the market, that transition may be challenging for property owners
3) Data security will be a large concern as the data points collected to price homes may be intrusive or require sensitive information which could easily lead to abuses or privacy violations
I would also be interested in seeing if Wake County may be able to scale this product to other districts or license the program to real estate agencies as a new source of revenue for the local government. This is definitely an exciting development, but one that must be handled extremely carefully to ensure it does not harm Wake County’s residents.
This is an excellent use of machine learning that brings accuracy and fairness to public sector assessments. Zillow and Redfin have long used machine learning to come up with expected values for millions of properties across the United States – it is well past time that local governments do the same.
I hope to see this model extended to other cities and states. In fact, it’d be best if the underlying algorithms and datasets are aggregated across municipalities to improve their predictive accuracy and reduce total operating costs to any one city government. The results of this data should be freely and easily accessible to the public, so that inequities in property taxes can be made more evident. My hometown of Los Angeles, by law, only charges property taxes on the last assessment conducted when a home was either sold or refinanced. If people truly understand the delta between actual taxes paid and current property values, we’d likely have fairer taxation systems.