Square Inc.: Demystifying the Black Box of Small Business Lending
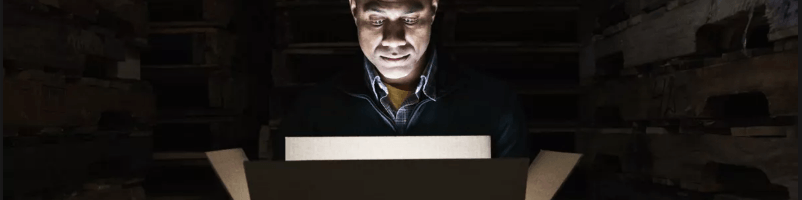
Square’s use of alternate data sources combined with machine learning is transforming access to credit for millions of small businesses.
The MSME Credit Gap
Small businesses, which make up the backbone of the American economy employing some 62 million people, face a notorious credit gap. More than 70% of small businesses seek loans less than $250,000. These small ticket sizes, coupled with the difficulty in determining credit risk due to information asymmetry i.e. lack of public information on comparable small businesses, make small business lending, frankly, an unattractive market from the viewpoint of traditional banks and financial institutions [1].

Square Capital: A Positive Feedback Loop
Square Inc., launched in 2009, was initially founded to solve another problem faced by small businesses: ability to accept point-of-sale payments. With a revolutionary hardware device, Square enabled merchants to take credit and debit card payments from their own smart phones. This initial payment product now provides the customer acquisition and essential data source for Square Capital, Square Inc’s small business lending arm. Square’s ability, unlike banks, to tap into transaction level data (through its payments product) has enabled $3.1 billion in lending to over 200,000 merchants with average loan sizes of $6,000 [2].
Square leverages machine learning to combine a variety of alternative data inputs including customer mix (new vs. returning), number and frequency of payments, processing volumes, inventory movements, and hiring practices to determine a merchant’s loan eligibility [3]. Access to a wide variety of offline and online data enables Square to predict both a merchant’s intent and ability to pay with limited manual intervention, thereby making the smaller ticket loans business margin positive, unlike traditional lenders. Given the regular working capital gaps faced by small businesses, credit availability helps to increases cash flows and grow baseline revenues creating a positive feedback loop to Square’s core payments business [4].
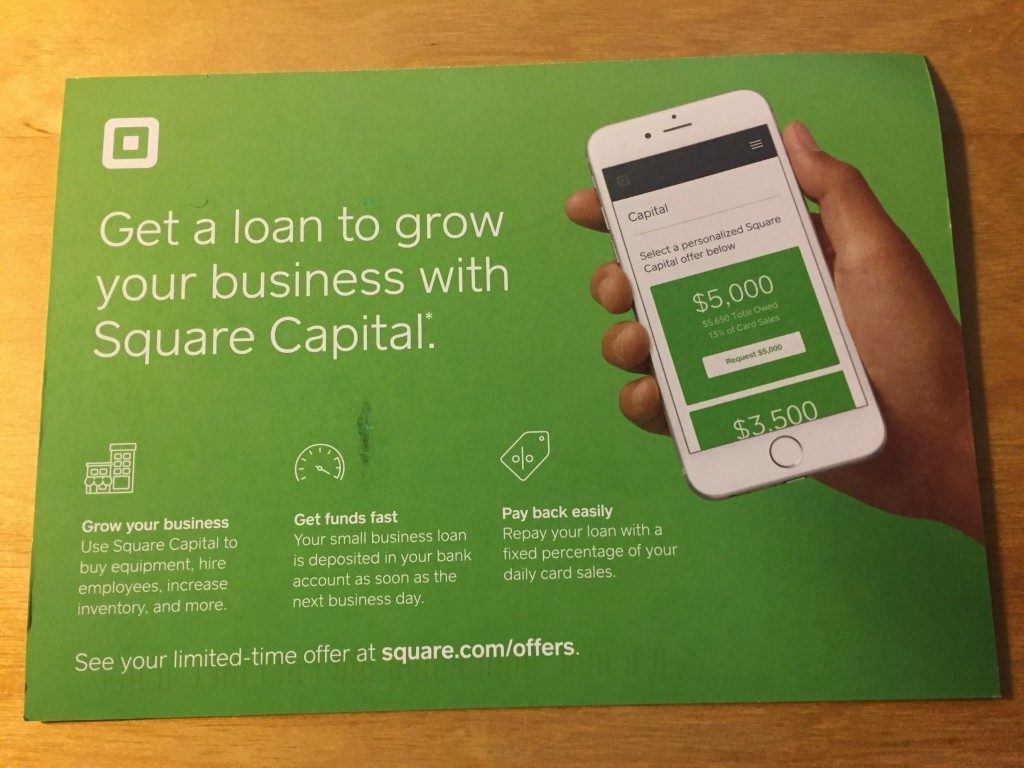
Alternate data breeds safer lending
Square has strategically transitioned from a batch, offline machine learning pipeline to a real-time architecture which allows for risk models to be integrated with real-time flow of payments data. This provides Square with the ability to design dynamic risk models and trigger early warnings for merchants at risk of default [5]. Former CFO, Sarah Friar recently asserted that Square’s machine learning capabilities can now be categorized as deep learning. For example, Square’s capital models in a specific geographic region will narrow i.e. tighten loan terms offered, for two to three weeks post a hurricane as the model interprets the drop in transaction frequency as a risk in the saliency of the businesses in the region [6]. In this way, machine learning offers greater security versus typical credit models which either ignore macro-weather risk or would see the event as irrelevant once passed.
Future plans
In Square’s most recent earnings call (Q3 2018), the new roadmap laid out involves three pillars: omnichannel, banking services and international expansion [5]. As Square continues to expand its machine learning capabilities to meet these pillars it will follow a four-step development workflow: defining the customer problem, collecting and understanding data, building a model, and iterating. In the medium term this includes scalable fraud detection, prediction of product fit for existing merchants to omnichannel offerings from Payroll to Customer Engagement, and predictive customer support i.e. chatbots [3].
While it is clear that Square’s strategy involves implementing their data science capabilities across company functions, Square should also be cautious to stay true to their core offering to small businesses: improving cash flows. A wide gap remains between the $6000 average ticket size offered today and the $250,000 request which banks are still unwilling to service [7]. Square should look at this as a greenfield opportunity, and therefore, rather than compete, find a way to share anonymized data with other players with access to SME alternate data sources including PayPal, Amazon and Stripe to build robust models around what forms of capital investment (ERP, CRM, real estate, etc.) in particular retail verticals / geographies / etc. are most aligned to increasing turnover. As a shorter-term in-process goal, Square should also track and incorporate data points that reflect core business growth decisions that its merchants have made since onboarding with Square’s payments product whether in pricing, selection, location, investment and how these reflect business stability and sustainability.
The idea of sharing data across the network of financial services players, while incredibly useful, also brings to light a large concern given the lack of regulation in the space today [8]. Square Capital does not hold the loans on their own balance sheets, instead selling them to third-parties. This creates an underlying risk that merchant privacy may be compromised if the data is used without the borrower’s consent, particularly given the high level of granularity of the underlying data. As a leading financial technology company in the small business lending space, does Square have a responsibility to define data empowerment policy as well?
(793)
References
[1] Karen Gordon Mills and Brayden McCarthy, “The State of Small Business Lending: Innovation and Technology and the Implications for Regulation,” Harvard Business School, Working Paper No. 17-042, (2016).
[2] Square Inc. (2018), “Square Capital”, https://squareup.com/capital, accessed November 2018.
[3] Sara Vera, “A Peak into Machine Learning at Square,” Medium (blog), August 4, 2017, [https://medium.com/square-corner-blog/a-peek-into-machine-learning-at-square-bdd75b272e23], accessed November 2018.
[4] Bloomberg Technology (2016), “How Square Capital Manages Risk”, https://www.forbes.com/sites/andyswan/2018/08/31/square-growth/#3c4005f0291d , accessed November 2018.
[5] Square Incorporation, September 2018 Quarterly Earnings Call. (San Francisco: Square Incorporation. 2018.)
[6] Recode Decode, “Square CFO Sarah Friar and Instacart CEO Apoorva Mehta,” September 28, 2018, podcast, http://podplayer.net/?id=57952450, accessed November 2018.
[7] John Heggestuen, “The Small Business Lending Opportunity: Paypal, Square, and competitors are moving into lending to help businesses grow,” Business Insider Intelligence (February 2015), Business Insider, Inc., http://www.intellengence.businessinsider.com, accessed November 2018
[8] Julapa Jagtiani, “The Roles of Alternative Data and Machine Learning in Fintech Lending: Evidence From the LendingClub Platform”, Philadelphia Federal Reserve, 2018, Pg. 3, https://www.philadelphiafed.org/-/media/research-and-data/publications/working-papers/2018/wp18-15.pdf], accessed November 2018
Interesting topic and very well written. It’s great to see Square (and Paypal, eBay, BlueVine) extend lending to small business owners in need of alternative cash sources. The machine learning aspect of the evaluation process here makes a lot of sense. The granularity of consumer behavior captured by Square seems to lead to much more informed credit judgments.
Worth pointing out that these loans are only available to merchants who use the Square POS system, so it’s a clever loop they’ve created. I think that the data security concern is a real one, and I’d be curious to learn more about how is addressing these concerns. One thing that’s missing from this piece is the structure of the loan itself and how it compares to other types of financing. How do the financing rates and repayment terms compare to other small business offerings? My research shows that repayment occurs as a fixed percentage of daily sales, so I would love to learn more about how merchants respond to this fluctuating obligation. I also see that Stripe is preparing to enter the space, so we shall see how this small business lending space changes over time due to competition.
I had no idea Square was doing this! I still think of them primarily as the payment transaction tool…I should have been paying more attention. (It’s because every hipster cafe in San Francisco uses the square app! ) Their expansion into small business lending appears to be perfectly strategic for their business. I particularly like how responsive their algorithms are when it comes to real-time information about risk. I believe this could lead to meaningful market expansion for them as they move into foreign countries that need this kind of lending for small businesses, but have a high incidence of natural disasters.
I do see the flow of data and lack of regulation as a challenge, especially as data privacy becomes an increasing concern – I’m thinking of GDPR, and similar policies that may be enacted elsewhere in the world. As a big player in this space I think Square has a responsibility to help shape future policies, and should take a proactive stance, even when dealing with other companies.
Loved this article as well as the Square’s move to provide loans to the small and mid-size businesses. To your question on data privacy, I wonder if, when Square sells the loan, they are not actually passing along the downstream data, but rather just the loan itself? This feels to me far more likely than the concern you pose of Square’s potential to transmit data without the lender’s consent (which I agree would be a significant ethical consideration!).