Drugs – can machine learning cure the world faster and also save Billions?
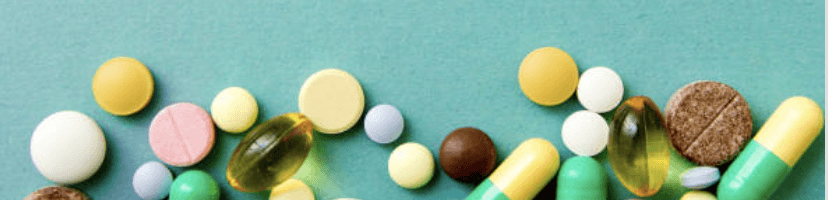
How machine learning is revolutionizing the drug discovery process and saving $$$
$2.5 Billion and 6 years – that’s the price tag of developing a new therapy treatment! [1] This is because finding a new effective drug is like solving a massive rubik’s cube with millions of smaller different color cubes. It takes testing millions of molecules over long-periods of time; after which 67% of them fail before they even reach phase-1 trials [2], effectively “pouring money down the drain”. Leading biopharma companies are now grappling with the question – can machine learning make the drug discovery process more efficient?
Diseases like cancer, neurodegeneration and others are “complex” because they result from multiple factors and have multiple gene interactions for one disease [3]. They can’t be treated by using a single drug or solution. They need combination therapy; which is combining two or more solutions to target a problem. The current R&D process for combination therapies suffer from high cost, long lead times and low efficiency because of the exponentially scaling costs and complexities associated with identifying potential combinations i.e. going from studying a single drug to test the disease to then using multiple combination pairs of drugs to triplets and so on [4].
As a result, any early studies on even small sets of combinations can cost millions of dollars and 4.5+ years in target identification time as illustrated below (Figure 1) and there are potentially millions of combinations that can be drawn. This traditional approach also faces high failure rates across the drug development cycle [5]
Figure 1:
Machine learning is a critical megatrend for process improvement in the drug discovery phase as it ensures a high throughput low-cost screening approach to target solutions that can enable companies to derive value from a few winners instead of spreading themselves thin. The platform can also address multiple use cases such as drug repurposing & novel drug discovery or precision medicine.
Engine Biosciences
Engine Biosciences [6] (Singapore/SF based co.) is one such company tackling this problem. It is an integrated platform company that is developing breakthrough therapies to target complex diseases in a significantly more efficient and scalable manner than existing methods used in early stage drug discovery. Their product development approach depends on using massively parallel processes running combinations (multiplexing CRISPR [7] using their proprietary barcoding mechanism) to build unique datasets with millions of data points. These are then fed to the machine learning algorithm which analyzes and keeps reiterating on the resulting datasets to identify the best solution or “combination” of drugs that can tackle a particular disease. The biggest advantage of this ML based approach to drug discovery is that it also takes into account molecules that might have been rejected in previous experiments.
Instead of developing completely proprietary list of molecules, ML also enables the use of publicly available datasets to build a computational and biological experimental technology which can identify solutions 10x faster and drive orders-of-magnitude gains in speed and scale. While a typical screen cost $1000 for a pharmaceutical company, it costs Engine $1. Think how long it would take for a simple excel to sort through million potential molecules combinations to find the perfect solution!
Leading biopharmaceutical companies are working with partners to solve this noble problem, but the efficacy of these projects still remains to be proven. For ex: Pfizer’s use of IBM Watson to search for immune-oncology drugs [8], Sanofi’s deal with Exscientia’s AI platform [9] as well as Genentech’s use of AI system from GNS Healthcare [10] are all to yet show strong results. One of the major concerns is providing enough correct data points to build a robust algorithm which can run at a greater efficacy rate. While publicly available databases like Chembl [11] can prove to be invaluable sources containing millions of bioactive molecules and assay results, they often suffer from inaccurate information and prior biases in categorizing molecules for eg – measurement systems can be wrong by orders of magnitude.
Engine biosciences can counter these issues in a two-fold manner. Firstly, building a propriety database combined with the existing public data can help to increase the precision of the algorithm and improve the quality of the data. Secondly, they can partner with personal care companies like J&J and P&G for screening identity markers using the companies’ historical data. This helps to build a credible database which can be free of previous biases.
A key question that remains open is if and how fast can engine’s platform be scaled in breadth to be applied to other complex therapies. One of the other concerns that companies need to address is around data governance and ensuring the integrity of the data so that it produces the right output. It would be interesting to see how fast can ML cure the world of cancer and save billions of dollars in healthcare costs!
[Word count 794]
Footnotes:
[1] Mullin, Rick “Cost to develop new pharmaceutical drug now exceeds $2.5B”, Scientificamerican.com, November 24, 2014. https://www.scientificamerican.com/article/cost-to-develop-new-pharmaceutical-drug-now-exceeds-2-5b/, accessed November 2018
[2] David W. Thomas, Justin Burns, John Audette, Adam Carroll, Corey Dow-Hygelund, Michael Hay, Biomedtracker, Sagient Research Systems, Informa, San Diego, California, USA. 2 Biotechnology Innovation Organization (BIO), Washington, District of Columbia, June 2016
[3]Mokhtari Bayat, “Combination therapy in combating cancer”, ncbi.nlm.nih.gov, June 6, 2017. https://www.ncbi.nlm.nih.gov/pubmed/28410237 , accessed November 2018
[4] Ucaine K.Scarlett, Dennis C. Chang. Thomas J. murtagh and Keith T. Flaherty, “High-throughout testing of Novel-Novem combination therapies for cancer: An idea whose time has come”, cancer discovery, september 2016; http://cancerdiscovery.aacrjournals.org/content/6/9/956, Accessed november 2018
[5] Lo, Chris, “Counting the cost of failure in drug development” Pharmaceutical-technology.com, June 19, 2017 https://www.pharmaceutical-technology.com/features/featurecounting-the-cost-of-failure-in-drug-development-5813046/. Accessed November 2018
[6] Engine biosciences, “Company overview”, https://www.enginebio.com/, accessed November 2018
[7]Ledford, Heidi, “CRISPR: gene editing is just the beginning”, March 07, 2016. https://www.nature.com/news/crispr-gene-editing-is-just-the-beginning-1.19510. Accessed November 2018
[8] Pfizer, “News – IBM and Pfizer to accelerate Immuno-oncology research with Watson for drug discover”,December 1,2016 https://www.pfizer.com/news/press-release/press-release-detail/ibm_and_pfizer_to_accelerate_immuno_oncology_research_with_watson_for_drug_discovery, accessed November 2018
[9] Fleming, nick, “How artificial intelligence is changing drug discovery”, nature.com, May 30,2018. https://www.nature.com/articles/d41586-018-05267-x Accessed November 2018
[10] GNS healthcare, “News – GNS Healthcare Announces Collaboration to Power Cancer Drug Development with REFS™ Causal Machine Learning and Simulation AI Platform” June 19, 2017 – https://www.gnshealthcare.com/gns-healthcare-announces-collaboration-to-power-cancer-drug-development/, Accessed November 2018
[11] Gaulton Anna, etc – Nucleic Acids Research, Volume 40, Issue D1, 1 January 2012, Pages D1100–D1107, September 23, 2011, https://doi.org/10.1093/nar/gkr777, Accessed November 2018
This is such an interesting aspect of machine learning that could definitely save the end consumer thousands and thousands of dollars — not to mention potentially save their lives!
However, I wonder whether or not algorithms are able to replace the scientific experimentation work or the discovery aspect that goes into developing new drugs. For example, some of the groundbreaking medicines like penicillin were discovered by accident in a lab setting. Or, scientists are able to test responses of different molecules in extreme conditions, and these often lead to new findings and innovation. Additionally, machine learning is best used to generate prediction and not causation — I question how this would be able to be effectively designed into an algorithm.