Paypal vs. Fraud – Have no fear, machine learning is here !
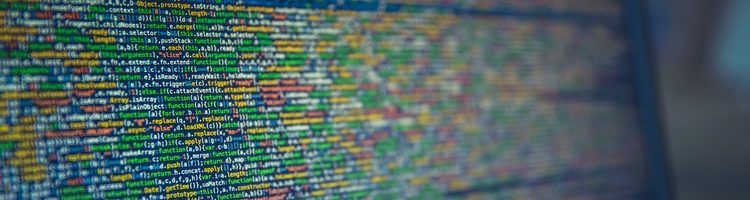
As globalization and online shopping increase the risk of fraud, consumers are looking to protect their data. Some companies, like PayPal, promise end-users to keep their information safe. But how can they deliver on this promise? Machine learning is certainly playing an increasing role.
Fraud in Financial Services
Globalization and online transactions have increased the risk of fraud – which has always been a key issue for financial institutions. McAfee, a global Security company, estimates in its 2018 report that “cybercrime currently costs the global economy some $600 billion, or 0.8% of global gross domestic product. One of the most prevalent forms and preventable types of cybercrime is credit card fraud.” [1]. Thanks to the large volumes of credit card transactions data, machine learning can be used to identify credit card behavior patterns that are irregular. It will constantly fit credit card transactions into dynamic clusters that it creates for accurate comparison, and then pinpoints potentially fraudulent transactions.
Fraud, Paypal and Machine Learning
PayPal is a digital platform that facilitates payments between two parties through online funds transfers. It connects a user’s credit card or bank account to an ‘online wallet’ that then enables the user to send or receive payments from other PayPal users. Although the transaction losses due to fraud “increased by $168 million, or 26%, in 2017 compared to 2016” [2], this increase can be attributed entirely to the increase in transaction volumes. Indeed, PayPal’s use of machine learning has kept its fraud rate “remarkably low, at 0.32 percent of revenue; a figure far better than the 1.32 percent average that other merchants see.” [3] So, how is Paypal leveraging machine learning in its quest to eliminate Fraud?
Wang, the senior director of risk sciences at PayPal, affirms that PayPal is a pioneer in risk management, as it uses three types of machine learning algorithms for risk management: linear, neural network, and deep learning [4]. The reason why risk management is so critical for PayPal is because their brand is built on the trust their customers put in them. Indeed, the reason why end-users are attracted to PayPal’s services is the fact that they do not need to provide different online shopping sites with all their credit card information and billing address and can therefore protect their personal information and prevent potential fraud. As such, quickly – i.e., in microseconds – deciding which customers and/or transactions are trustworthy and which ones are not, is the key to PayPal’s sustainable growth. According to Wang, running relational queries and linear algorithms, among other techniques, is sufficient to filter out trustworthy customers. However, more problematic transactions require more sophisticated algorithms, complex neural structures and in-depth screening – which require large quantities of transaction data. [4] Wang explains how Paypal applies different machine learning algorithms together:
“Let’s take a linear algorithm. You might think it’s outdated, but it still potentially catches something the nonlinear algorithm might not be able to. So in order to get the best out of all [three], we “ensemble” them together. We have a “voting committee.” One is linear and one is nonlinear and we just ask them: What is your opinion on this file? Then we take their vote and eventually ensemble them together for our final assessment … It’s like taking a lot of doctors and listening to all of them. With that kind of community-based voting, hopefully something better or more accurate will come out.” [4]
In light of its strong focus on machine learning to detect and eliminate fraud, in June 2018 PayPal acquired a startup, Simility, in a transaction valued at $120 million. [5] Founded in 2014, Simility leverages machine learning to detect potential fraudulent transactions. For instance, “Simility looks at various session, device, and behavioral biometrics and builds a profile for what constitutes ‘normal’ user login behavior; if an anomaly is spotted, it acts to prevent the action.” [5] This transaction shows PayPal’s commitment to growing its machine learning capabilities and deliver on its promise as a company.
What’s Next?
However, one might wonder – do these advances in machine learning mean that there will be no place for people in the decision-making process of detecting fraud? Wang had a clear view on this widely publicized topic: “I never worry that these machines will replace humans. […] There is so much data, so much variety, but the flip side is: What is useful? We still rely on human oversight to decide what ingredients to pump into the machine.” [4] Her argument is that the success of machine learning depends not only on the availability of a large number of data points, but also on the quality of these data points which only a human brain can judge. Indeed, it seems like fraud analysts and machine learning complement each other, rather than replace each other.
Comparison between fraud analyst and fraud machine learning [6]
Another question that comes to mind as PayPal thinks about the future – as they train to catch fraudulent transactions through machine learning, will criminals learn how to dupe the machine?
(Word Count: 773)
[1] Vian Chinner, “Artificial Intelligence And The Future Of Financial Fraud Detection”, Forbes, June 4, 2018, [https://www.forbes.com/sites/theyec/2018/06/04/artificial-intelligence-and-the-future-of-financial-fraud-detection/#196de87127a6], accessed November 2018.
[2] Paypal, 2017 Annual Report, P.43, [https://investor.paypal-corp.com/secfiling.cfm?filingID=1633917-18-29&CIK=1633917], accessed November 2018.
[3] Michael Morisy, “How PayPal Boosts Security with Artificial Intelligence”, MIT Technology Review, January 25, 2016, [https://www.technologyreview.com/s/545631/how-paypal-boosts-security-with-artificial-intelligence/?fbclid=IwAR2OgJHyujzaUT9gJO9XFJtNluLL_oVfx53CgWaF00Gja0EeJxFCuGjClGw], accessed November 2018.
[4] Eric Knorr. (April 13, 2015 Monday). How PayPal beats the bad guys with machine learning. InfoWorld Daily News. Via LexisNexis Academic, accessed [11/2018]
[5] Paul Sawers. (June 21, 2018 Thursday). PayPal to acquire machine learning-powered fraud detection startup Simility for $120 million. VentureBeat. Via LexisNexis Academic, accessed [11/2018]
[6] McKenna, F. (2017, February 23). 5 Reasons Why Machine Learning Models Will Never Eliminate The Fraud Analyst. Retrieved November 11, 2018, from https://frankonfraud.com/fraud-operations/5-reasons-why-machine-learning-models-will-never-eliminate-the-fraud-analyst/
This was a very interesting article and raises some important questions. I agree with you that a key question here is once criminals learn to dupe the machine, how will Paypal ensure the safety and security of its customers? Paypal will have to think carefully about how to compensate customers if and when this does occur. Additionally, Paypal will need to always stay abreast of the best in class fraud detection and prevention technologies. For this reason, I do not think that machine learning could ever replace humans at Paypal, because human fraud analysts will need to be the ones that are in the know on all the latest technologies.
Great article, thanks for sharing! I was particularly impressed with Paypal’s tactic of combining linear and non-linear algorithms to create an “ensemble” that can better predict the level of fraud on any given transaction. Since Paypal is an online payment system connecting banks and consumers, It would be interesting to know how Paypal is working in collaboration with banks on checking out fraudulent activity. Since we know banks and credit card companies constantly check transactions, it would be interesting to know if the work is being done twice (once at the bank, and once at Paypal), and if so, whether they are both employing the use of machine learning or not. The sheer number of transactions that a machine can proof may appear to threaten the jobs of fraud analysts, but there is a big difference in quality versus quantity. Fraud analysts (or any human mind for that matter) provide a level of quality in judgement that machines cannot outsmart…at least yet!