Partnering with AI: Optum Labs’ Efforts to Improve U.S. Health Care
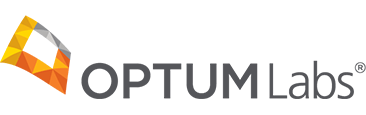
Through industry partnerships, a 200 million life database, and the use of artificial intelligence (AI), Optum Labs is confronting the daunting cost and quality challenges facing the U.S. health care system
The cost and quality challenges facing the U.S. health care system have been well documented over the years. The U.S. currently spends over $10,000 per capita, totaling over $3.3 trillion dollars in annual health care spend[1]. Although U.S. spend exceeds all other developed nations, the U.S. fares worse than the Organization for Economic Cooperation and Development (OECD) average on most measures of health quality, including life expectancy[2]. It is within this context that pressure has been mounting for organizations to identify methods to improve health care quality while lowering cost.
Optum Labs was established in 2013 with the purpose of using data to tackle health care’s biggest problems. Through partnerships with over 25 organizations spanning the industry, including hospitals, payers, policy makers, academic institutions, and medical device manufacturers, Optum Labs has created a database of over 200 million de-identified lives containing linked data from insurance claims, electronic medical records (EMRs), and self-reported health information[3]. Using this immense data set and new machine learning technologies, Optum Labs’ goal is to “turn artificial intelligence into health care intelligence”[4].
The use of machine learning is critical to achieving Optum Labs’ goal due to the unstructured and disjointed nature of health care data. According to Optum Labs, roughly 80% of data in EMRs are physician’s unstructured notes[4]. Without the use of AI, utilizing this enormous amount of unstructured data in conjunction with other fragmented claims and administrative data sets would be nearly impossible. The concurrent use of these data sets, through AI, allows Optum Labs’ to not only better understand the health of individuals, but also their impact on industry costs.
Big data and the use of machine learning has allowed Optum Labs’ to compliment a clinician’s judgement with access to a massive amount of pertinent experiences from other clinicians and patients. This supplementary data provides additional support to clinicians when creating treatment plans for complex cases, hopefully leading to better patient outcomes. In addition to care delivery improvements, Optum Labs has focused its use of AI on solving practical business problems and improving disease prediction. Machine learning techniques have been used to develop predictive models that can diagnose conditions such as diabetes, heart failure, Alzheimer’s, and dementia years in advance of traditional methods[5].
Predictive models are also being used for cost saving efficiencies. In 2017, Optum Labs began using AI to automate pre-screening medical charts and forecasting out-of-network and emergency department utilization, two large causes of high consumer spend[5]. Utilizing AI, Optum Labs was also able to create a prototype network design tool that identifies smaller networks of providers that have naturally collaborated in patient care. In one test market, the tool has shown that these smaller, natural networks can achieve better outcomes at ~20% lower cost than some defined payer networks[6].
Optum Labs has already made great progress in identifying ways to address the U.S.’ quality and cost challenges; however, there is still many years’ worth of work to be done to solve these inefficiencies. Moving forward, Optum Labs has begun focusing the application of AI to the analysis of EMRs. The goal over the coming years is to use natural language processing to extract meaning from physician notes and feed this data to machine learning models[4]. Optum Labs must be careful in this venture as physicians may have different observations on the same patient, especially in complex cases. Varying physician perceptions makes this EMR data difficult to utilize appropriately without increasing the susceptibility to AI error.
Another important consideration for Optum Labs to consider, as it continues to obtain and analyze data, is the minute amount of time most patients interact with the health care system. Patients who currently do not suffer from chronic conditions only interact with the health care system a few times a year; yet, it is these patients that Optum Labs hopes to benefit with early diagnosis. To optimize this process, Optum Labs should focus on obtaining data on consumers outside of their interactions with providers and payers. Optum Labs could benefit greatly by partnering with organizations such as Fitbit, Apple, and Samsung that produce wearable technologies. Additional partners, such as Amazon and Google, that understand the gamut of consumer behavior could help complete patient personas and further link behavior to health outcomes.
It is clear that, when used appropriately, AI can improve clinical outcomes and enhance operational efficiencies. However, compared to other industries, health care has greater inherent risks. It is one thing to use AI for operational efficiency and waste reduction, but when used in clinical scenarios controls must be put in place to protect patients and clinicians. The question remains: What types of control systems can Optum Labs implement against inaccurate AI insights? How can Optum Labs protect clinician partners from the threat of malpractice resulting from AI research errors?
(796 Words)
[1] Centers for Medicare and Medicaid Services, “Historical National Health Expenditure Data,” https://www.cms.gov/Research-Statistics-Data-and-Systems/Statistics-Trends-and-Reports/NationalHealthExpendData/NationalHealthAccountsHistorical.html, accessed November 2018.
[2] Joseph Walker, “Why Americans Spend So Much on Health Care—In 12 Charts,“ The Wall Street Journal, July 31, 2018, https://www.wsj.com/articles/why-americans-spend-so-much-on-health-carein-12-charts-1533047243, accessed November 2018.
[3] Optum Labs, “Our story,” https://www.optumlabs.com/about/story.html, accessed November 2018.
[4] Optum Labs, “Harnessing artificial intelligence (AI),” https://www.optumlabs.com/work/artificial-intelligence.html, accessed November 2018.
[5] Optum Labs, “2017 Year in Review” (PDF File), downloaded from Optum Labs website, https://www.optumlabs.com/about/year-in-review.html, accessed November 3, 2018.
[6] Optum Labs, “Designing better provider networks,” https://www.optumlabs.com/work/designing-networks.html, accessed November 2018.
While I do think that Optum has a responsibility to act ethically (as I believe all companies do) I think it is possible to frame their product as a decision augmentation tool for doctors, which would simply provide them with more data, as a recommendation. It would be still up to professionals what the appropriate course of action (in the case of a diagnosed disease) or diagnosis (in the case of symptoms or pain in a patient).
Thanks for sharing, Alex. I agree on the potential to harness data for good in operating efficiently and reducing waste, and think that having human interpretation of the results would be a best practice for both operational and clinical use cases. The notion of malpractice is an interesting one when it comes to using AI for clinical decisions. If the decision rights remain with the clinician, I think it make sense for them to remain the responsible party.. if the decision is made between alternatives presented from an AI algorithm, however, a clinician may not have the opportunity to even make the right choice if the algorithm is buggy or doesn’t work as expected.
Thanks for a great article, Alex. I think you make a really great point when you write about how Optum Labs focuses on patients who do not suffer from chronic conditions, so are only interacting with the health care system a few times a year. For this reason, partnerships with Samsung, Apple and companies like Fitbit makes a lot of sense. In other words, how can Optum Labs work on early diagnosis by meeting patients where they are in their day to day lives, instead of in the doctors office. OL will need to put a huge focus on making sure that there IS a control systems to ensure that there are not inaccurate AI insights (or at least as few as possible). As we see with AI in other industries, like in autonomous vehicles, the public reacts VERY strongly to any issues in automation, even though human error if often much more common than machine error. This means that there will need to be intensive training for staff and experts on how to use these tools, and also the tools shouldn’t be administered until they are completely ready, because as you said, when used in clinical scenarios the controls must be even tighter to protect clinicians and also patients. Awesome work!
This is really great Alex, thanks for sharing. I especially value your point that the total sum of time spent in healthcare interactions for most patients is tiny compared to their experience outside of interactions with providers and payors. This is an unmet need that will be addressed, as you said, by using existing technologies, such as smartphones and wearables, to understand what types of data can be gather about patients continuously and how we can identify meaningful patterns in this massive stream of data that can then inform the care that we provide to patients. I’m hopeful that we will see this come to fruition in our lifetime.