Overpromising and Underdelivering at Sloan Kettering: is AI Still ‘Human’ After All?
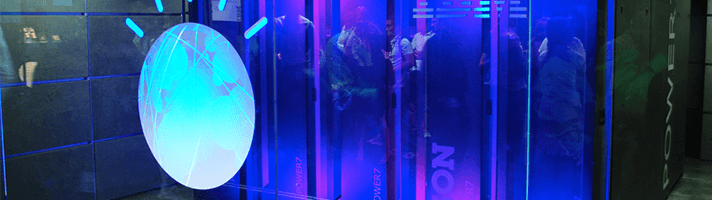
The introduction of artificial intelligence to healthcare has sent shockwaves through the system with the promise of dramatic increases in quality of care and organizational efficiencies coupled with much needed cost reductions. The promise of AI in healthcare predicts a futuristic world where machines are primary and human doctors are secondary. The reality is tempered by the many challenges facing the widespread adoption of AI in US hospitals. A review of the adoption of IBM Watson Health at Memorial Sloan-Kettering Cancer Center provides a case study.
AI holds a particular appeal in the realm of healthcare due to the major inefficiencies with the status quo and the excitement around what could be possible as quality data becomes available at exponentially increasing rates. Among many use cases, AI can assist physicians in staying up-to-date on the most recent and relevant scientific studies, reduce human errors when diagnosing patients, and leverage large population health datasets when predicting outcomes [1]. The inputs available today are categorized as either structured or unstructured data. Machine Learning (ML) works best with structured data such as diagnostic imaging, genetic testing and electrodiagnosis, while Natural Language Processing (NLP) works to extract information from unstructured data sources such as physical exam notes and clinical laboratory results [1].
In 2012, IBM formed a partnership with Sloan Kettering to use IBM’s NLP technology named Watson Health to develop a clinician decision support tool for treating oncology patients [2]. Executives at Sloan Kettering were eager to leverage Watson’s ability to read 200 million pages of data and produce a well-analyzed result in 3 seconds to help clinicians stay abreast of the cutting edge research and scientific studies when diagnosing and treating oncology patients [3]. The ability to rapidly review and interpret the latest scientific studies is of paramount importance. Each year, tens of thousands of oncology research papers are published every year, amounting to over 100 new papers everyday [4]. Clinicians are physically unable to keep up with the rapidly accumulating information, creating the opportunity for Watson to assist oncology clinicians in summarizing relevant research. In the short term, Sloan Kettering has sought to address this problem of rapidly accumulating research with a limited ability to translate the information into improved patient care by collaborating with Watson on a diagnostic support tool.
Challenges over recent years have cast doubt on Watson’s ability to meet its high expectations, leaving an unclear path forward for Sloan Kettering. The root cause of the disappointment is the underestimated complexity of training the software. In interviews conducted with STAT in 2017, Sloan Kettering’s lead Watson trainer, Dr. Mark Kris, complained of the painstaking time required of the physicians who are helping to train the data to input their own recommendations into Watson [5]. Not only is this resource intensive for hospitals to take on with the hope of increased efficiencies in the future, but there runs the risk that the outputs are only as good as the inputs – meaning if the physician operators input incorrect diagnoses into Watson, then the future outputs will also be faulty. Further complicating the success of the partnership are the frequent changes in the broader healthcare landscape that may require overhauls in the system. For example, Dr. Kris referenced recent changes to treatment protocols for metastatic lung cancer patients that changed protocols worldwide within a week [5]. While Watson may be able to ‘read’ staggering amounts of data in seconds, getting to that point of speed and accuracy requires significant amount of manual inputs of new literature and patient cases – a process that must be repeated for each type of cancer the product intends to serve. Over the short-term Sloan Kettering has collaborated on a product that is still in its stages in infancy. Moving forward into the medium term, the cancer center remains dedicated to its partnership despite the slow progress.
The inherent risk of launching a massive commercialization effort for an AI technology before it is fully developed and its efficacy is rigorously proven, is that distrust between AI software developers and health care providers could limit future adoption of effective AI solutions. In addition to pursuing this clinical decision support tool, I would encourage healthcare providers like Sloan Kettering to explore the adoption of AI in other realms of patient care. In contrast to the NLP deployed by Watson Health for Oncology, GE has been developing a suite of ML technologies that couple well with their diagnostic imaging machines. In the summer of 2017, GE entered into a 10-year partnership with Partners Healthcare in Boston to build a bridge between GE developers and clinicians to create products that assist doctors with a variety of functions starting with interpreting medical images [6]. Diagnostic imaging by humans has a high error rate [7] and the structured data typically makes for relatively easier product development. The success of the Partners and GE partnership is yet to be determined, but moving towards more agile, high impact products seems like a step in the right direction.
As a member of the executive team at a hospital, at what point do you decide that a collaboration has cost too much and yielded too little? As a patient, how would you feel about your physician using a diagnostic support tool for oncology compared to your radiologist using a diagnostic imaging support tool? (799)
[1] Jiang F, Jiang Y, Zhi H, et al. Artificial Intelligence in Healthcare: Past, Present and Future Stroke and Vascular Neurology. 2017; 2:doi: 10.1136/svn-2017-000101
[2] Bowman, D. (2013). New watson-based tool sends docs to the cloud for cancer treatment. FierceHealthIT, Retrieved from http://search.proquest.com.ezp-prod1.hul.harvard.edu/docview/1466241812?accountid=11311.
[3] Hirsch, Marla Durben. (2012). Memorial Sloan-Kettering, IBM Partner on Latest Watson Technology Project. FierceHealthIT.com
[4] Gachon University Gil Medical Center Adopts IBM Watson for Oncology Trained by Memorial Sloan Kettering, Marking Watson’s First Deployment in Korea. (2016, Sep 07). PR Newswire Retrieved from http://search.proquest.com.ezp-prod1.hul.harvard.edu/docview/1817414493?accountid=11311
[5] Ross, C. and Swetlitz, I. (2017). IBM pitched Watson as a revolution in cancer care. It’s nowhere close. [online] STAT. Available at: https://www.statnews.com/2017/09/05/watson-ibm-cancer/ [Accessed 11 Nov. 2018].
[6] GE and partners HealthCare launch AI initiative. (2017, Jun 19). M2 Presswire Retrieved from http://search.proquest.com.ezp-prod1.hul.harvard.edu/docview/1918988908?accountid=11311
[7] Understanding and Confronting Our Mistakes: The Epidemiology of Error in Radiology and Strategies for Error Reduction. Michael A. Bruno, Eric A. Walker, and Hani H. Abujudeh. RadioGraphics 2015 35:6, 1668-1676
[Image] Monegain, Bernie. “IBM Watson, Quest Diagnostics, Memorial Sloan Kettering Cancer Center, MIT, Harvard Combine Forces for Massive Oncology, Precision Medicine Initiative.” Healthcare IT News. 2016. Accessed 2018. https://www.healthcareitnews.com/news/ibm-watson-quest-diagnostics-memorial-sloan-kettering-cancer-center-mit-harvard-combine-forces.
It seems like the real issue is how to efficiently input accurate date into the algorithm so it can learn to produce correct outcomes. I optimistically hope that this will become easier as more and more of the healthcare system becomes digitized. I’d imagine raw data would need an intense quality control even when information is digital, but this could alleviate some of the pain. I also wonder if our expectations are too high. Can AI like Watson Health already add value by augmenting the work of doctors? Was IBM too ambitious with its goals and could it have had more of an impact attempting to complement doctors rather than attempt to take away much of their work?
Considering the factors that will influence patient/consumer perceptions of diagnostic support tools is crucial in evaluating their rate of adoption (1).
On the one hand, we know that there is a gap between the knowledge accumulated in most domains of medicine (and codified in guidelines) and the actual care delivered by physicians (2). Clinical decision-support tools can play a critical role in addressing this gap, especially if they are embraced and effectively used by physicians as part of their workflow.
However, as mentioned in this article, the push to commercialization of these technologies with unproven efficacy risks damaging patient/consumer and physician confidence. Given the critical impact that a diagnosis can have, for a patient that is aware of a tool’s use, the lack of confidence would span across specialties (radiology and oncology). Perhaps then, the question is not strictly of specialty but of efficacy. As a patient, how would I feel about my physician using a diagnostic support tool? Great – but only if it works!
1. Herzlinger RE. Why innovation in health care is so hard. Harv Bus Rev. 2006;84(5):58.
2. Bates DW, Kuperman GJ, Wang S, Gandhi T, Kittler A, Volk L, et al. Ten Commandments for Effective Clinical Decision Support: Making the Practice of Evidence-based Medicine a Reality. J Am Med Inform Assoc. 2003 Nov 1;10(6):523–30.
I firmly believe in a ‘bite and chew’ approach. Watson is taking on too much all at once. IBM was definitely too ambitious on this one. And it seems like the algorithm’s structure is itself a major reason why it is so difficult to train (in addition to other aforementioned reasons).
To the question, “As a member of the executive team at a hospital, at what point do you decide that a collaboration has cost too much and yielded too little?” A possible answer is when you determine that a significant amount of additional cost needs to be invested to move the technology a step further in the face of a high-impact albeit smaller alternative that can begin to yield results today. (Think next best alternative)