Narrative Science, the Automated Journalism Startup

Narrative Science’s technology can help journalists reach new heights – our replace them altogether.
Narrative Science is a Chicago-based startup that has developed technology that enables it to automatically transform raw data into written narratives. The company’s natural language generation platform, called Quill, leverages machine learning to create customized stories, such as sports game recaps and news articles.
Why Machine Learning is Important to Narrative Science

The estimated global volume of data is expected to grow 10x between 2016 and 2025 from 16 Zettabytes to 160 Zettabytes (1 Zettabye = 1 Trillion Gigabytes)[1]. However, while data is forecasted to grow exponentially, the number of professionals capable of analyzing that data is growing linearly, at best. One of the many reasons Narrative Science was founded is to address this issue.
To tackle this problem, the team at Narrative Science is employing the use of natural language generation (NLG), a software process that automatically transforms data into narratives written in natural language. Narrative Science uses NLG to improve organizational productivity by automating time-intensive data analysis and routine reporting activities, two well-known pain points in the technology and media industries. The company chose to focus on these applications due to its unique roots, as it was founded through a collaboration between two professors from the McCormick School of Engineering and students from the Medill School of Journalism, Media, and Integrated Marketing Communications at Northwestern University[2].
The company’s initial prototype, called StatsMonkey, was made to automatically generate short news recaps of baseball games from relevant game data such as players, game score, and hitting performance[3]. After seeing the tremendous potential for the technology, the company successfully commercialized its IP with the launch of its advanced NLG platform called Quill, which analyzes structured data to automatically generates narratives written in human-like prose. The company’s Quill platform, offered as a software-as-a-service (SaaS) product, is used by online media outlets and sports networks to vastly increase the efficiency of the wire reporting process, a traditionally time-intensive effort due to the large number of games and events these organization cover.
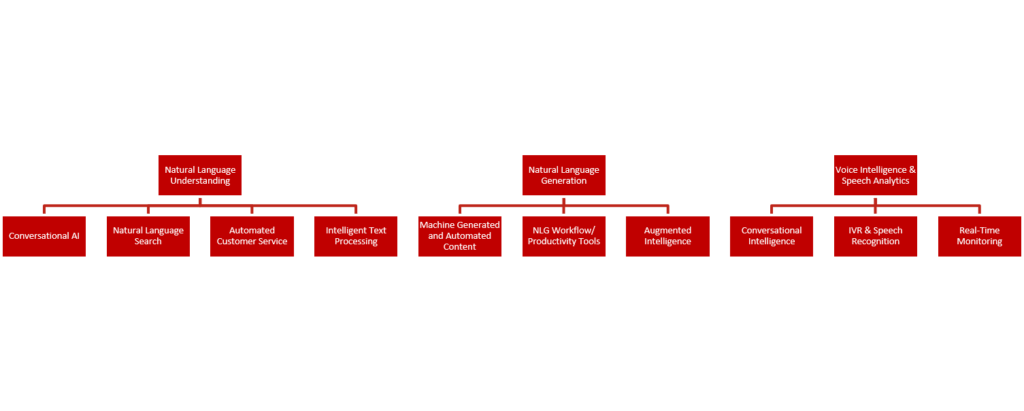
How it Works
Narrative Science focuses on the production of machine generated and automated content, a sub-sector within NLG. Companies in this sub-sector use machine learning and pattern recognition to scan large data sets, discover key insights, and automatically generate written narratives. At a technical level, Narrative Science’s production engine works by amassing high-quality data, teaching algorithms to fit that data into some “broader understanding of the subject matter” (such as the rules of a baseball game), and then using a team of “meta-writers” (trained journalists) to turn that analysis into natural language using templates that vary in structure according to the subject at hand (such as sports or finance).[4] Over time, the algorithms automatically learn to communicate data in the tone, style, and language of each client, as the platform is capable of understanding user intent and identifying what is most important to the target audience[5].
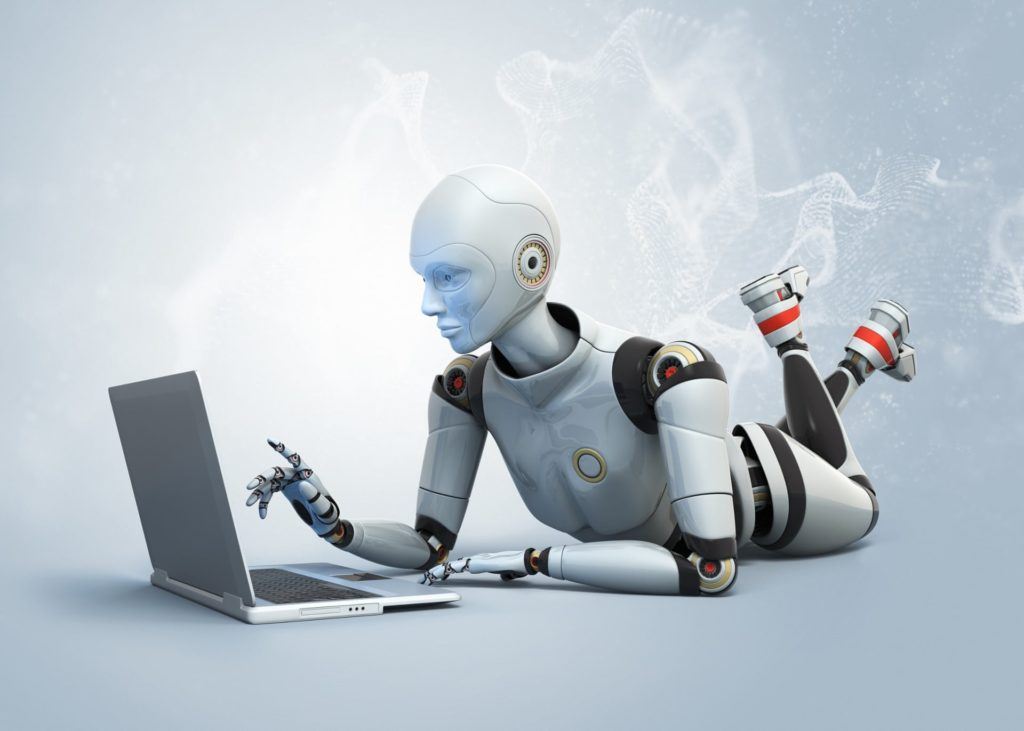
Product Expansion: Quill for the Enterprise
After focusing initially on sports, Narrative Science saw an opportunity to further leverage the company’s machine learning capabilities by adding additional features that allow it to deliver customized insights for all types of enterprises. In the short-term, the shift towards becoming an “insights” company will allow Narrative Science to expand its footprint across the enterprise, creating value for both external stakeholders of an organization (such as a consumer who read the paper) as well as internal stakeholders (such as a CEO that reads an internal report). Instead of producing simple game recaps, helping organization realize true potential of their data should enable Narrative Science to differentiate itself in an increasingly crowded market.
New Product Development: Dynamic Narratives
Additionally, Narrative Science is also in the process of launching Dynamic Narratives, a suite of natural language extensions that can be embedded business intelligence platforms to explain not readily obvious in charts, tables, or graphs. For this effort, the company was honored at the Chicago Innovation Awards in 2017[6].
The numbers don’t tell you anything. It’s the understanding of those numbers and translating them into something that is readable that’s a story that matters -Narrative Science CTO and Co-Founder Kristian Hammond. (Levy, 2018)
Next Steps for Narrative Science
One area I hope the company’s management considers focusing on is in expanding local news coverage. Due to the immense pressures on the media industry and business model, an increasing number of small and independent news organizations have been forced to close or consolidate with larger firms in recent years. To its credit, the company is saying the right things, as management recently stated its hope to eventually be used to uncover the “small-scale” stories that journalists overlook [7] But these applications need to be more carefully considered going forward.
Open Questions Still to be Addressed
Despite the company’s claims, there is genuine fear in the media industry that Narrative Science, and startups like it, will eventually one day replace journalists altogether. Is this fear warranted? Will the computers at Narrative Science replace paid writers? Will there still be a need for reporters in 10 years? If so, what will their role be?
(791 Words)
[1] Aguilar, A. (2018). The data landscape: A report for Facebook. [online] Deloitte UK. Available at: https://www2.deloitte.com/uk/en/pages/technology-media-and-telecommunications/articles/the-data-landscape.html [Accessed 14 Nov. 2018].
[2] Adams, T. (2018). And the Pulitzer goes to… a computer. [online] the Guardian. Available at: https://www.theguardian.com/technology/2015/jun/28/computer-writing-journalism-artificial-intelligence [Accessed 14 Nov. 2018].
[3] Narrativescience.com. (2018). About Us | Narrative Science. [online] Available at: https://narrativescience.com/Resources/About-Narrative-Science/About-Us [Accessed 14 Nov. 2018].
[4] Levy, S. (2018). Can an Algorithm Write a Better News Story Than a Human Reporter?. [online] WIRED. Available at: https://www.wired.com/2012/04/can-an-algorithm-write-a-better-news-story-than-a-human-reporter/ [Accessed 13 Nov. 2018].
[5] Hammond, J. (2018). Narratives Science Products- Quill. [online] Narrativescience.com. Available at: https://narrativescience.com/Products/Our-Products/Quill [Accessed 13 Nov. 2018].
[6] Northwestern Engineering. (2018). Narrative Science Receives Chicago Innovation Award. [online] Available at: https://www.mccormick.northwestern.edu/news/articles/2017/11/narrative-science-receives-chicago-innovation-award.html [Accessed 14 Nov. 2018].
[7] Levy, S. (2018). Can an Algorithm Write a Better News Story Than a Human Reporter?. [online] WIRED. Available at: https://www.wired.com/2012/04/can-an-algorithm-write-a-better-news-story-than-a-human-reporter/ [Accessed 13 Nov. 2018].
Very interesting topic, and equally interesting questions. I think some of the fears you allude to are warranted, but are also natural. The introduction of new technology into pre-existing industries always causes growing pains in the labor market. Certain skills, and sometimes even entire professions, become obsolete. At the same time, however, new professions and demanded skillsets emerge to replace them, as human ingenuity is needed to integrate said new technology into old industry. Will paid writers or journalists be replaced at some point in the future? Perhaps, but I think the more difficult question to answer is whether or not this kind of new technology will be a net positive on the labor market as a whole as people are forced to adapt themselves to the new environment.
Thanks for the post! This essay reminds me of an article I recently read about China’s first AI news anchor (https://www.npr.org/2018/11/09/666239216/ai-news-anchor-makes-debut-in-china) – the AI anchor innovation invites similar analyses, as mentioned in the comment above (i.e., what will the impact be of such changes on the labor market). Beyond workforce participation, however, I wonder if there are an additional set of questions around the implications of potential machine-learning-driven mistakes and vulnerabilities? For example, mistakes made in Jeopardy and in baseball game recaps may be less consequential than mistakes in an article about fiscal or monetary policy. Moreover, would these (news-gathering and news-presenting) algorithms become targets for interference from non-friendly actors?
Very interesting article! While it seems like machine learning can help report facts on a small scale, my concern is that it is more difficult to interpret qualitative information that typically makes up journalism. If journalism truly takes this direction on a large scale, I can imagine fewer interviews with individuals and more of a focus on analyzing data sets and taking the human experience out of the equation.
This is a fascinating research Brian! I see parallel in the rest of media (movie and TV development, and even music) – but in those fields the experts (producers) stand firmly with the stance that machine learning can aid with screening of human-created content, but could not produce content, because what’s most valuable in media is creativity and human interpretation [1]. I think same logic can be applied here, as the audience of a sports article, for example, read it mainly for the human interpretation of the data. If people are simply interested in the data and their summary, there are faster ways to view those (e.g. charts and other data summaries) than NLG.
1. https://www.intotheminds.com/blog/en/artificial-intelligence-wont-replace-you-if-you-are-creative-heres-why/
“There is genuine fear in the media industry that Narrative Science, and startups like it, will eventually one day replace journalists altogether. Is this fear warranted?”
It’s worth noting that journalists do more than put words on paper. They also go out and collect data, which Narrative Science can’t do. But I could imagine a future where the ubiquity of sensors means all the data is already being collected. Then what? I don’t think the analysis and detective work that journalists do is trivial, and so I don’t see Narrative Science as even a long term threat. Maybe one day, in the far future, NLG will be sophisticated enough to replace journalists, but then we’ll all be out of jobs.
This is a really interesting company, I can see a lot of applications for this across sectors. For exampe, consolidating market research reports or helping policy makers and academics read through large quantities of academic reports. Regarding your question about journalism, one of the things I really value in journalism is the point of view and analysis that a journalist provides. In addition, a big part of journalism is interviewing people to draw out insights and develop narratives and stories. While I can see this being useful for summarizing facts, I think a human can provide unique insights and storytelling aspects to make stories truly come to life and provide analysis and a point of view. To me, this seems like a great example of how machine learning can augment a person’s job and give them more room for creativity.
Very interesting article and thought provoking question. Particularly: “There is genuine fear in the media industry that Narrative Science, and startups like it, will eventually one day replace journalists altogether. Is this fear warranted?” I think in addressing this question it is important to think about the different types of activities that journalists do and which of those lend themselves to being completed by machines. While there are certain type of news output (financial data / performance, sports data) that is highly structured and likely able to be replaced by a machine, there is a large part of what journalists do that do not lend themselves to machine learning platforms, for example: building sources, gathering and interpretting information that is not in the public domain. Hopefully, by automating some of the less nuanced work we can free up journalists to do more impactful work.
Brian this is so interesting, thanks for sharing this story. I read something similar in the book ‘Rise of the Robots’ if you are interested in learning more and totally agree that it is an inevitable trend.
Two thoughts:
1. Our society is increasingly sensitive to terminology journalists use in news coverage, whether the topic is Middle Eastern politics, Women’s issues, or election coverage. I wonder if algorithms are capable of understanding, and continually adapting to, these subtleties. If not, shaping tone could become an even larger part of a journalist’s role, which unfortunately might further undermine our society’s fleeting trust of journalists if they come to be seen as there to add spin.
2. I also wonder if journalists have a role in curation of news stories, given that a journalist might be able to think about curation on more sophisticated levels beyond what will generate the most clicks. Unfortunately, as long as media outlets are funded by advertising, driving clicks will continue to be the norm, which seems to only strengthen the case for using more ML in lieu of journalists.
Brian, great story. I would echo the sentiment in some of the previous posters in that, newspaper readers, whether it is on sports or politics, want something more than facts. They look for opinions, reactions, player remarks, the illusion of suspense to feel like they were at the game. Unless AI can provide this, I see a place where they are desired. In my work in investor relations, I have seen the advantages of AI in curating earning reports of competitors and summarizing. Where it was useful for me or the CEO for example, is a quick summary and a guide for what details I needed to dig into. At the end of the day, unless you can form an opinion on what that competitors announcement means to you, the technology will only be a funnel for end users.