“Minority Report” a Reality? The NYPD’s Big Data Approach to Predicting Crime
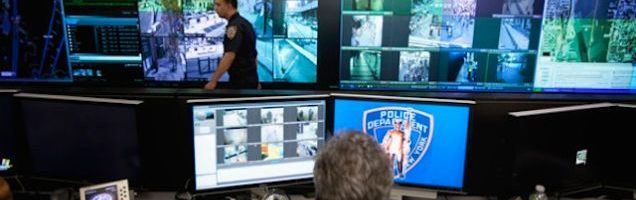
The New York Police (NYPD) has developed a data-driven approach to fight, and even predict, crime. A huge success – it helped reduce crime in NYC by 75% and made it America’s safest big city.
“Big Data”-driven predictive policing works by analyzing regularly recorded crime data (location, time, and crime), using sophisticated computer models and algorithms, to predict places of expected criminal activity. The predictions are narrowly tailored, limited to 500-by-500-square foot areas, and usually updated on a daily basis. Police officers are then deployed to those identified areas to deter crime from happening. Crunching numbers from crime statistics has therefore become a routine part of police work. [7]
NYPD Commissioner Bill Bratton has been perhaps the most aggressive advocate of data-based policing in the world. He introduced the data-driven, real-time “CompStat” performance management system to the NYPD in 1994 [1]. The system is based on tracking and stopping smaller crimes to stop bigger crimes, and to pinpoint hotspots where crimes are clustered. 22 years later, Compstat is still in use in NYC, and crime has fallen 75% — a fact Bratton calls a “New York miracle.” The city had 1,946 murders in 1993, or more than a five a day. It had 352 in 2015. [2]
CompStat
CompStat is a combination of management, philosophy, and organizational management tools for police departments.
It offers a dynamic approach to crime reduction, quality of life improvement, and personnel and resource management, whereby police department executives identify spikes in crimes using comparative statistics and address those spikes through the use of targeted enforcement. It includes 4 components:
- Timely and accurate information or intelligence
- Rapid deployment of resources
- Effective tactics
- Relentless follow-up. [3]
Operations
On a weekly basis, personnel from each of the NYPD’s 77 precincts, 9 police service areas and 12 transit districts compile a statistical summary of the week’s crime complaints, arrests and summons activity, as well as a written report of significant cases, crime patterns and police activities. This data, with specific crime and enforcement locations and times, is forwarded to the chief of the department’s CompStat Unit, where information is collated and loaded into a city-wide database. The unit runs computer analysis on the data and generates a weekly CompStat report which presents important performance indicators, making it easy to discern emerging and established crime trends, as well as deviations and anomalies.
The CompStat program involves weekly crime control strategy meetings between the agency’s executives and the commanders of operational units – enhancing accountability by providing local commanders with considerable discretion and resources. The program also ensures that precinct commanders remain aware of crime and quality of life conditions within their areas of responsibility. [3]
Critisism
Critics have pointed out that factors other than CompStat have led to a decrease in crime in NYC, such as an increased number and better training of police officers, end of the crack epidemic, housing vouchers, etc. [3]. There have also been reports of manipulation of data – retired police officials complained that pressure from department brass prompted widespread statistical manipulation of CompStat data, specifically by downgrading reports of serious crimes to less serious offenses [4]. Some people are also worried about discriminatory practices such as profiling [5], however, companies point out that crime prediction software only analyzes type, place and time of crimes rather than involving any information on individuals. [6]
Outlook & Recommendation
CompStat has been successfully exported to other U.S. cities and abroad. [2, 3] Furthermore, new products have been developed: thousands of police officers in 60 cities across the U.S. are using predictive maps from an extension of CompStat called “PredPol” to help them decide which areas they should patrol during their shifts. Crime in LA’s Foothill precinct went down 20% from 2013 to 2014 using the program, Atlanta saw a near 10% drop in crime for two precincts, and Santa Cruz’s police department witnessed a combined 38% drop in robberies and burglaries. [5]
The results of big data policing are promising. My recommendation is to implement the system in more cities, while also paying more attention to preventing data manipulation. Additionally, I would recommend that a data-driven approach be applied to other areas of policing, such as deciding which police officer is deployed. Last year a policeman in Texas, who had responded to two suicide calls that day, was then dispatched to a children’s pool party where he ended up pulling out his gun. Ideally, the station would have sent a less stressed officer. [9]
The applications of machine learning to public policy are vast. According to Stephen Goldsmith, a professor at Harvard and a former mayor of Indianapolis, it could also transform almost every sector of public policy. [9]
(749 Words)
[1] Fortune. 2016. How Big Data Is Helping the NYPD Solve Crimes Faster. [ONLINE] Available at: http://fortune.com/2016/07/17/big-data-nypd-situational-awareness/. [Accessed 17 November 2016].
[2] NBC News. 2016. An Inside Look at the System That Cut Crime in New York By 75 Percent – NBC News. [ONLINE] Available at: http://www.nbcnews.com/news/us-news/inside-look-system-cut-crime-new-york-75-percent-n557031. [Accessed 17 November 2016].
[3] Wikipedia. 2016. CompStat – Wikipedia. [ONLINE] Available at: https://en.wikipedia.org/wiki/CompStat. [Accessed 17 November 2016].
[4] The Huffington Post. 2016. What The CompStat Audit Reveals About The NYPD | The Huffington Post. [ONLINE] Available at: http://www.huffingtonpost.com/2013/07/12/nypd-compstat_n_3587637.html. [Accessed 17 November 2016].
[5] Lauren C. Williams. 2016. Police Departments Use Big Data To Predict Where Crime Will Hit Next. [ONLINE] Available at: https://thinkprogress.org/police-departments-use-big-data-to-predict-where-crime-will-hit-next-56dc95457a07#.ptiyml7tr. [Accessed 17 November 2016].
[6] PredPol. 2016. 5 Common Myths About Predictive Policing | PredPol. [ONLINE] Available at: http://www.predpol.com/5-common-myths-predictive-policing-predpol/. [Accessed 17 November 2016].
On the whole, the concept of using big data to make our cities safer is perfectly logical and forward-thinking. If we have the information readily available, it makes sense to utilize it in a way that will better people’s lives. While you’ve pointed out that analytics software only predicts the type, place, and time that a crime could potentially occur, police work is still ultimately done by people. I think that there is still a very real risk of profiling that would need to be strongly addressed in police training.
I’m curious to know about the causation of crime reduction. Provided that the data was not manipulated, crime has clearly gone down in the areas where analytics software was utilized. Was this simply because police presence in the area was enough to deter the crime? Were more arrests made? If analytics becomes standard policing, I can also see a risk to police departments in terms of how they choose to use this information in order to ensure resources are deployed appropriately (e.g. a disproportionate amount of resources in a more affluent neighbourhood less prone to crime but with some predictor of potential crime).
Thanks for writing on this topic – it was a very thought-provoking post!
Clemens, this was such an interesting read!
I’m quite excited by the prospect that this innovation will help achieve the efficiencies of scale in data mining that will allow police departments to search for, find and use critical information which is most likely to improve decision making and officer safety, and of course, faster case resolution.
My only concerns are with respect to two things that are quite idiosyncratic to the world of police departments- for example it appears that across geographies, the day to day needs and demands of law enforcement are very different from one another- the needs of a Police Chief in the midwest would be very different from say, major cities on the East or West coast.
I also worry about the traditional nature of police officials- who prefer ‘old school’ file keeping and crime-solving methodologies, and if there will be a “fear of technology” as this initiative is set to scale across the larger US. I wonder if these factors would contribute to increase complications in the adoption of such analytics by US law agencies.
Either way, this was super-cool- so thanks a lot!
Great read. It seems that the system is offering great results and seems like a good potential for cities around the world, based on the efficiency that it demonstrated. However, before recommending to expand the program further, I would love to understand what safeguards the NYPD has put in place to make sure that the data is handled in an ethical ways and what protection practices they have set up to prevent leaks from the system. Generally, as you underline, Big Data can offer strong promises in terms of holistically tackling social issues, however, it comes with a big tradeoff in the risk that the data manipulation represent.
Thank you Clemens, for enlightening us with this. I agree with you that data manipulation is a big risk, as flawed data may affect the machine learning into having false arrests. I am for the use of technology in fighting crimes, as you pointed above there are a lot of advantages of doing so. Furthermore, machine learning would ensure that there is no more human bias in crime prevention but also that there the right decisions are made. I am wondering how this technology could be employed on the ground, and how a machine can learn from policemen and thus avoid that they make the wrong decisions e.g. shoot an innocent person. As humans, we are more likely to make flawed decisions under pressure and enforcement officers such as policemen, although they are better trained and less likely to make errors, they are still human and still prone to these errors.
Why stop here! Big data firm, Palantir, apparently had partnered with the American government to help locate IEDs in Iraq, based on obscure data like moonlight visibility (logic being explosives would most likely be hidden in darker places, not easily spottable!)
Awesome! This is a case that really shows the power of data! To your point, it is now up to the leaders to decide how to best use this data to improve the operations/performance of their units. I am sure that this can have implications in both emergency officer dispatching and preventative police resource planning and allocation. I wonder whether the data could ultimately result in a bias in the way the NYPD operates. Since the nature of crime in general is often a function of irrational behavior, the NYPD may be wrongly adopting predictability into a space where it cannot exist. If police offers are prepared for a given situation based on the predictions of their data (trends based on location, time, etc.), they may walk into an emergency with a bias that could under prepare or over prepare them for the situation at hand. Could this ultimately compound the issue of excessive police use of force if data is wrongly predicting the severity of the call that they are dispatched to?
I enjoyed reading your post, but I’m well aware of the risks. For example, in Brazil, companies have used big data to legitimize the fact that they refuse to serve poor neighborhoods where they would lose money… just find (or even worse, develop) a (safety) statistic and you’re set.
Very interesting post, Clemens. Thank you for sharing. I love Minority Report, so this was a fascinating read for me. It is amazing what has been accomplished with this technology in NYC. What do you think are the broader implications are for this technology in combating global terrorism? I imagine that the CIA also uses sophisticated technology when making tactical military decisions. For example, Palantir was said to have contributed to the finding of Osama Bin Laden
Nice post Clemens. I wonder how body cameras for police officers will integrate into this system. My understanding is that the implementation of body cameras has been quite difficult – given the technology challenge that comes with storing vast amounts of video data. There is likely a future where an individual sitting in the command room can pull up the camera of any officer via CompSTAT, and layer it on to the existing data. The cameras will help hold cops to a higher level of accountability, and could potentially record clues and important crime scene data that a human might miss.
Clemens, I agree that the results, although not 100% clear a result of the big data output, are compelling and indeed a big success for New York but the issue I have with this system are some of the ethical dilemmas that could arise. It is great you mention the short story/movie minority report because the whole point of the movie was to evaluate the dilemma of arresting someone who was expected to commit a crime but never did. Although this system is not leading to then arrest of people before they commit a crime there could be some intrusion on the liberties of individuals who live in certain areas. Is it really fair to have an intense police presence in an area that is predicted to have crime? Especially during the era of stop and frisk these areas would have been subjected to what you could argue was unwarranted scrutiny. I also would be concerned that some of the data may point to correlation but not causation and could inadvertently target certain groups of people unfairly, whether it be by race or socioeconomic class. Again, the results are amazing so the dilemma is whether the benefits outweigh the costs and how you can implement a system that does not discriminate or impede on individual rights.
Interesting and important topic! The closer you look, the more fault (crime) you find. These data then feed back into the system for prediction. My point is, the system could be cyclical. It reminds me of the drug war that predominantly affected African Americans. Once you start with American American community for marijuana-related incidents, even without knowing the race of the people involved, the system as you described will increasingly flag African American communities. As we know, US cities are still quite racially segregated. And it is known that white people are much less likely to be searched, arrested, and punished for marijuana possession or use – and would this system lead to less search in white communities? I am just worried that biases could be introduced despite claim of neutrality and the effects can be cyclical.