Man or Machine: Who holds the “stronger suit” in the courtroom?
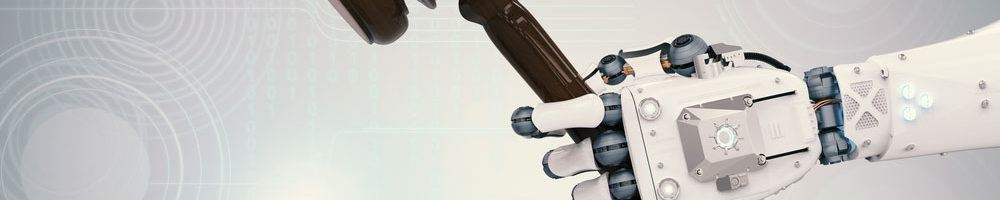
Firstly, let me acknowledge that I am no expert in the megatrend of machine learning and therefore writing about an atypical organization might be a bit of a bold move. I shall express my thoughts nonetheless – because of how much this topic area has intrigued me over the past few days.
My choice of organization for this analysis is the US Justice Department. Machine Learning as a megatrend has been extremely critical to the “process improvement” for global justice systems at large, and in this case the extent of adoption and its implications can be studied by diving deeper into the evolution of trials in some US courts. What makes machine-learning adoption (or the lack of it) as critical in this context is the gravity of its implications – we’re juggling between inherent human biases and potentially faulty algorithms to decide on the future of defendants in the court of law.
Several studies in the past, including Austin & Williams’ 1977 note on sentencing disparity [1], have highlighted the consistency problem with the judicial system – bringing to light the fact that court judges even in the same state end up with very disparate judgements for the same cases. In more than a few instances, it has also been highlighted that the same judges arrive at different judgements when presented with the exact same case, just at a different point in time [2]. This makes it apparent that there are several biases at play when forming judgement, as with every other scenario that requires human interpretation.
With the courtroom stakes as high as they are, it would make sense that the US Justice Department would seek to employ methods that could help eliminate some of these human biases. Therefore, to no surprise, the Justice Department’s National Institute of Corrections encourages the use of “(algorithm-based) assessments at every stage of the criminal justice process” [3]. After all, using algorithms in the process allows for greater consistency – with the expectation that the outcome will be more standardized and therefore equitable for every case where the parameters match. However, my research in this area has highlighted the shortcomings of this very claim – because it entirely depends on comparing cases on a pre-defined set of parameters, ignoring any other context the case may provide.
While there are several dimensions to this challenge, we shall explore the risks of overdependence on algorithms using one simple real-world example. Privately developed tools such as COMPAS [4] – which have been designed to assess risk and predict re-offense rates of defendants – have been commonly used across several state courts to make decisions on plea bargains. The deployment of this tool in Wisconsin’s court was subject to negative publicity when in one case (Paul Zilly – convicted of stealing a lawnmower), the judges changed their decision from agreeing to a plea deal to instead doubling Zilly’s sentence to two years in state prison, based on the risk assessment presented by the algorithm [3]. While the algorithm is “proprietary” and there is no way of knowing the true accuracy of the prediction, what is apparent is that there is a bias towards believing that the “machine” is right – therefore putting additional onus on the computer program being fair and holistic.
Are the systems that are being used fair and holistic however? Are our machine-learning capabilities sophisticated enough that we value system-generated trial judgements over the “subjective” conclusions of judges who come with decades of experience and the ability to empathize with individual contexts? While there is no single answer to these questions, my analysis leads me to believe that there are sufficient grounds to be skeptical.
There is a school of thought with the belief that the increased dependence on algorithms will allow us to eliminate human bias during trials, which as I understand is not necessarily true. At the very core of this challenge is that even in very sophisticated programs, the ‘machine’ is set up to ‘learn’, at least partially, from historic incidences and behavior of crime. One of the several implications of this phenomena is that the algorithms will continue to make race and gender biased predictions, even when race and gender might not be coded as parameters to match against, leading to increased false positives [5].
It is important therefore, especially in a sensitive and high-stake environment such as the judicial system, to “get it right”. What should be the stance of the US Justice Department in this transitionary phase of machine learning sophistication? How do we strike a balance between taking advantage of the technology currently available and the traditional procedure for trials? Should all computer programs in this field go through a centralized audit and review process before being allowed to go-to-market? Looking forward to your comments!
(Word Count: 783)
Endnotes:
Overall concept credit:
Hannah Fry. Hello World: Being Human in the Age of Algorithms. W. W. Norton & Company Inc. (2018)
(where the author provides a narrative on the evolution, current applications and potential future of algorithms across seven industries)
[1] William Austin and Thomas A. III Williams, “A Survey of Judges’ Responses to Simulated Legal Cases: Research Note on Sentencing Disparity”, The Journal of Criminal Law and Criminology, Vol. 68 Issue 2, https://scholarlycommons.law.northwestern.edu/jclc/vol68/iss2/12/ accessed November 2018
[2] Ben Bryant. “Judges are more lenient after taking a break, study finds”, The Guardian, April 11, 2011. https://www.theguardian.com/law/2011/apr/11/judges-lenient-break accessed November 2018
[3] Julia Angwin, Jeff Larson, Surya Mattu and Lauren Kirchner, “Machine Bias”, Pro Publica, May 23, 2016. https://www.propublica.org/article/machine-bias-risk-assessments-in-criminal-sentencing accessed November 2018
[4] Adam Liptak, “Sent to Prison by a Software Program’s Secret Algorithms”, The New York Times, May 1, 2017. https://www.nytimes.com/2017/05/01/us/politics/sent-to-prison-by-a-software-programs-secret-algorithms.html accessed November 2018
[5] Hannah Fry. Hello World: Being Human in the Age of Algorithms. W. W. Norton & Company Inc. (2018): p. 62
Image Credit: Christopher Markou, “Are We Ready for Robot Judges?” (blog), Discover Magazine, http://blogs.discovermagazine.com/crux/2017/05/16/are-we-ready-for-robot-judges/#.W-pFU5NKg2w, accessed November 2018
This is a very interesting topic. The author is right in pointing out that there is room for process improvement at the US judicial system. While I agree with his point on the potential for judges’ biases, I would add that human judges should be better at flexibly adjusting to the latest social and cultural trends than machines. I think it is an important point because the “right” verdicts constantly change as the society keeps changing. So to answer his question on what the US Justice Department should work on, I believe an important aspect to address is to teach machines how to learn the latest social and cultural trends and to accordingly change the way they reach verdicts that they think are “right.”
Training data fed to machine learning algorithms that make up these models becomes incredibly important in this situation for many of the reasons pointed out by the author above. If the model is trained to assess accuracy on the basis of demographic information and the historical decisions of judges which are inherently exposed to human biases – the model will reflect all of those biases in its assessment. Moreover, it’s not clear to me how this type model can actually react to information that has come to light over the course of the case (where there is often different sets or facts or at minimum different interpretation of facts presented), rather the judgement formed by these types of models seem to be based on historical data and demographics, which to me feels less relevant here when we are assessing facts presented by parties for accuracy and measuring those against ever changing legal standards. For these reasons, I’m skeptical that machine learning would be anything but detrimental in this current application.