Machine Learning – In Theatres Now
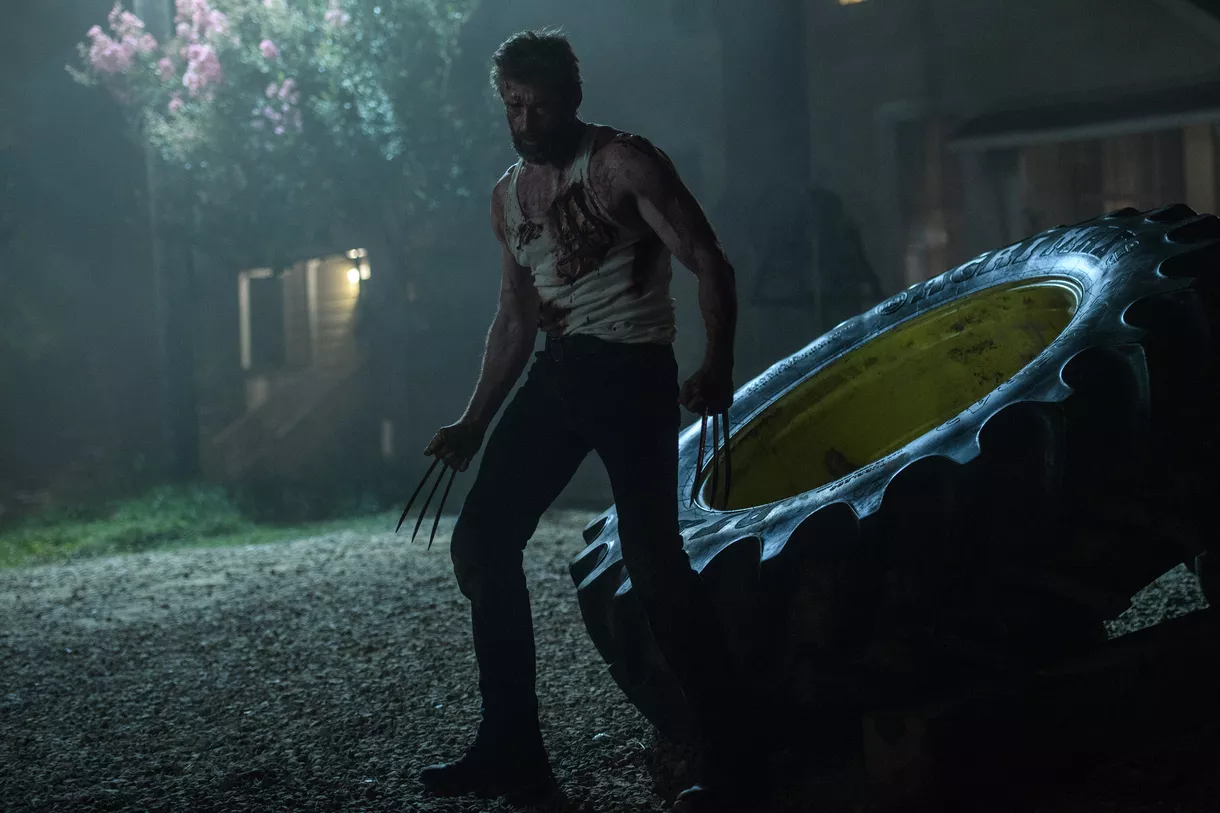
Movie studios are using machine learning to try to predict audience demand and develop new titles
Importance of the megatrend: Machine learning as a predictive tool for movie viewership
Given the lengthy time periods and high costs associated with producing feature films, studios are strongly incentivized to invest in projects with maximum potential viewership. Estimating future demand for any given title is difficult, so studios have used past experience to inform investment decisions on new scripts. This approach can risky, especially for original stories.[1] Major movie studios have started to use data science to better understand the composition of their audiences and more accurately forecast demand for new releases.[2] Using machine learning to analyze movie trailers and overlay historical viewing data, 20th Century Fox hopes to learn more about movie goers to better predict what kind of movies they may want to see in the future. Studios have historically relied on traditional methods such as interviews and questionnaires for insights on audience composition[3], but the limitations of these methods made granular audience segmentation challenging.[4] Without such ‘micro-segmentation’ of an audience, demand forecasting for an individual title is almost impossible. Researchers at 20th Century Fox have built “an experimental movie attendance prediction and recommendation system,”[5] called Merlin, which they hope to use to inform greenlighting decisions, as well as marketing and distribution strategies for new releases.
Organization’s response: 20th Century Fox applies ML to movie trailers
Fox partnered with Google Cloud, using its ML deep learning framework TensorFlow[6] to create Merlin, a computer vision tool that learns patterns in movie trailers to predict a trailer’s future audience up to a year in advance of film release. This is an example of a pure cold-start prediction that is challenging for brand new movies[7]. Merlin first scans the trailer, returning the most frequently occurring elements, taking into account the sequence in which they appear, since timing of each object’s appearance is correlated with film genre.[8] Merlin then compares this information with analyses from past trailers and combines it with historical viewership data to create predictions of audience behavior. A comparison of Merlin’s predictions for viewers of Logan (right hand column) with actual results (left hand column) demonstrates that Merlin correctly predicted all 5 of the top actual movies (in green in the left hand column), but fails to rank them correctly.
However, there are some noteworthy mismatches, such as the prediction that Tarzan and The Revenant will be popular with fans of superhero movie Logan. Despite these kinks, in a little over a year, Fox has created a predictive tool that is now used routinely in making business decisions. Looking further ahead, management is refining how to use Merlin to further inform marketing strategy and its performance tracking of new releases. The team is introducing scorecards that compare actual versus predicted box office performance for new releases which can be shared with the rest of the organisation.
Recommendation and Key question: Can machines really be movie buffs?
In the short term, researchers at Fox need to improve Merlin’s accuracy to make its predictions more useful. In the medium term, however, it remains to be seen whether or not the tool can accurately determine future demand or decide what kind of movies to make. This is in part due to the nature of the attendance data on which the Merlin model is trained. A variety of factors influence consumers’ decision to see a particular movie, including who they are with, title availability, and even the impact of impulsive behavior. Research has demonstrated that people are more likely to depart from their preferences when making consumption decisions in a group (a frequent movie goer configuration)[9]. The fact that an individual watched Logan in the cinema in the past is not necessarily an indicator that he or she will go see another superhero movie under different circumstances. I would therefore be cautious in inferring too much from historical viewership data, and use the tool primarily to gain granular insights on the composition of past audiences. When it comes to the tool’s ability to guide future content creation, it is important to recall that people are notoriously poor at predicting what they will like in the future, and past preferences are not necessarily an indication of future tastes. Accordingly, a model that uses past viewing patterns to determine content of future films could have serious limitations, suffering the same pitfalls that plagued Amazon’s machine learning hiring algorithm[10]. As Netflix series Stranger Things has demonstrated, often the most popular original concepts are not engineered based on what has worked in the past, but rather created by visionary screenwriters who have the ability to create something truly novel. In the age of cookies, in which the consumer is bombarded with content based on past purchasing behavior, do audiences want more than ever to be surprised and delighted by cinema, or is content based on past viewing patterns more likely to be a hit?
Word count: 808
[1] Miguel Campo and Sona Oakley, “How 20th Century Fox uses ML to predict a movie audience,” Google Cloud Blog, 29 October 2018, https://cloud.google.com/blog/products/ai-machine-learning/how-20th-century-fox-uses-ml-to-predict-a-movie-audience
[2] Cheng-Kang Hsieh, Miguel Campo et al, “Convolutional Collaborative Filter Network for Video Based Recommendation Systems,” (October 2018)
[3] James Vincent, “20th Century Fox is using AI to analyze movie trailers and find out what films audiences will like”, The Verge, November 2, 2018, https://www.theverge.com/2018/11/2/18055514/fox-google-ai-analyze-movie-trailer-predict-success-logan
[4] Campo Oakley, “How 20th Century Fox uses ML to predict a movie audience”
[5] Hsieh, Campo et al, “Convolutional Collaborative Filter Network for Video Based Recommendation Systems,” (October 2018)
[6] https://www.tensorflow.org/
[7] Ibid.
[8] Vincent, “20th Century Fox is using AI to analyze movie trailers and find out what films audiences will like”, The Verge, November 2, 2018
[9] Ratner, R. K., & Kahn, B. E. (2002). The impact of private versus public consumption on variety-seeking behavior. Journal of Consumer Research,29(2), 246-257. https://www.jstor.org/stable/pdf/10.1086/341574.pdf
[10] Alex Woodie, “Do Amazon’s Biased Algorithms Spell the End of AI in Hiring?,” 18 October 2018 https://www.datanami.com/2018/10/16/do-amazons-biased-algorithms-spell-the-end-of-ai-in-hiring/
As a movie buff, I’m kind of wary when it comes to machine learning’s role in predicting future hits in the industry. I believe that movie hits are a result of creative screenwriting and the ability to tell a story (no matter what it is) in an engaging way, so in my opinion past data can be used as another tool to assess historical viewer preferences but will not be very useful in making predictions about future blockbusters. For this reason, I think that its applicability would be more valuable on the marketing and distribution strategy side, rather than on the content creation side.
Thanks for the interesting article. Because cinema can be considered an art form, I sense an inherent conflict in Fox’s efforts to combine machine with an expression of human imagination. This leads me to infer there are limits to the technology’s benefits. For example, will Fox begin to rely on the output of Merlin more than the intuition of a seasoned film executive who can sense the potential for an instant cult classic? Another thought related to the above – given the human element of creative skill in film, is open innovation a better megatrend for Fox to consider when gauging demand? Said another way, could it harness the visions and opinions of its customers to help generate content in a controlled manner? Additionally, as streaming content stands to disrupt traditional media channels, is Fox focuses its resources on solving the right problem?
As a movie fan, this article is fascinating to me. It’s smart to use machine learning to predict potential movie hits. However, I believe it will only be useful to a certain extent because the factors that drive each movie to be hits does not only depend on titles or contents but also depend on abilities to tell stories (production), which machine learning might not be able to help that much. In order for Fox studio to succeed in the long run, I would recommend them to continue to leverage machine learning but still focus on bringing out creativities from a human being as well as develop skills for the production team to create high-quality movies to attract audiences.
Interesting article about how ML can predict demand for types of movies! You brought up an important missing piece for studios, which is the ability to bucket types of consumers and monitor externalities such as social influence on the decision making process. This article made me think a lot about MoviePass and its ability to provide granular, consumer level data on movie watching trends. While many wonder how they will sustain their current subscription business model, I actually think their end goal is to build up a diverse set of data across many theaters, geographies, and demographics, and then sell it to movie studios or theaters to feed into programs such as Merlin. With the flexibility of an app, it would be easy to tweak the kinds of data being collected. So for example, adding a social aspect to see patterns in groups of friends and perhaps information on the tipping point for users watching an “unexpected” genre of movie. Studios can then cater their marketing or production to these trends.