Machine learning in the Chemicals industry: Lyondellbasell
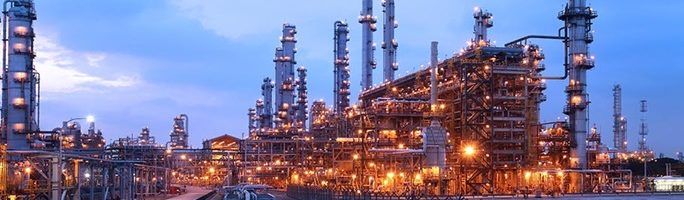
Despite limited investments to date, the industrial giant is primed to extract benefits from machine learning across its value chain
Lyondellbasell (LYB) is an integrated Chemicals company, with significant market positions in all major organic materials (example products include plastics and color dyes) and across the value chain from R&D to manufacturing to sales. As the chemicals industry begins to adopt digital innovations, LYB is exceptionally situated to benefit from advances in machine learning; though they have yet to make significant investments in the space, this report contends that LYB’s current status as the most efficient asset operator and it’s access to wide and deep datasets uniquely positions it to improve both its business processes and its product development capabilities.
The value of machine learning in Chemicals
Machine learning has applications across the entire Chemicals value chain, shown below (simplified):
Exhibit 1: Simplified Chemicals value chain
R&D: Chemicals companies rely on their R&D organizations to develop a wide array of product innovations, from big (synthesizing new/novel plastic polymers) to small (developing new colors to meet customer needs). By deploying machine learning technology to ever expanding datasets, companies can discover new combinations of materials and paints more rapidly.
Feedstock selection: With their newer plants that can accept multiple feedstock sources (e.g., naptha vs. propane vs. ethane), Chemicals companies face constant optimization decisions. As each feedstock produces a different proportion of end products, managers rely on accurate assessment and projections of both input and output prices. Machine learning can support appropriate modelling of commodity supply and demand.
Manufacturing: Like other firms in the industrial sector, Chemicals companies are using machine learning to improve their production processes. Leveraging massive operational datasets and IOT technology across a number of plants, these companies can use machine learning for predictive maintenance (i.e., proactively predict when a machine might breakdown to schedule maintenance) and optimization (i.e., recommend optimal temperatures, pressures, etc. to improve output).
Sales / Customer service: At the end of the value chain, companies field a high volume of technical questions from their customers. As in other industries, machine learning can be used to create virtual assistants that can provide quick assistance to customers.
As the Chemicals industry is highly commoditized, the benefits that machine learning brings in terms of efficiency and costs reduction can create significant competitive advantages. A number of LYB’s competitors have started to adopt solutions across the value chain.
Exhibit 2: Competitor solutions
R&D | DOW/1Qbit[1]: Dow Chemical began a collaboration in 2Q2017 to use quantum computing and machine learning to discover new useful materials and optimize existing formulations |
Feedstock selection | Shell[2]: Shell’s Downstream division is using AI to predict supply/demand for oil (and it’s chemical derivatives) and recommending appropriate refining mixes |
Manufacturing | BASF[3]: The German company has partnered with Schneider electric for predictive asset analytics |
Sales/customer service | Shell[4]: Shell has deployed its virtual assistant technology across 151 countries to answer questions around technical properties of products |
LYB’s positioning in Machine Learning
LYB is notably behind on adoption of the technology (at least publically). There are no mention of artificial intelligence or machine learning in their annual report or in their previous 3 earnings calls. However, LYB’s operational decisions has potentially made it uniquely positioned to take advantage of this megatrend:
- Feedstock flexibility: Roughly 50% of LYB’s assets have flexibility to accept more than one feedstock. While industry benchmarks are not widely available through public sources, LYB’s asset base is known to be best-in-class in this metric, given the investments the company made in their naphtha assets during the shale revolution to accept gas-based feedstock. As such, optimization of feedstock selection would have an outsized impact for LYB[5]
- Best-in-class, diversified assets: With over 50 assets across 17 countries producing meaningful volumes for every organic chemical (#6 position or better in every market), LYB has an extensive and ever-expanding dataset to utilize. Moreover, LYB is widely recognized as the most efficient operator in the space (LYB’s EBIT/employee is over 2x the nearest competitor), and would thus be able to train AI on a truly best-in-class dataset[6]
- Acquisitions: LYB’s position as the future industry consolidator will give it access to an even more comprehensive and extensive dataset (LYB recently completed the integration of A. Schulman and is negotiating a purchase of Braskem, the Brazilian national firm)[7]. Furthermore, LYB could apply the benefits of optimization through machine learning to a wider base of assets.
Recommendations
The obvious recommendation for LYB is to identify partners to develop machine learning solutions, as its competitors have. However, they must also take a number of steps before effective solutions can be implemented:
- Improve data capture: LYB should invest in IoT technology across its asset base to effectively capture leading indicators of performance
- Integrate/normalize data: As it continues to grow through acquisition, LYB should take care to carefully integrate and normalize data across ERP systems
- Identify and prioritize highest value opportunities: Finally, LYB should take care to invest selectively to maximize ROI. Where is machine learning truly needed? (as opposed to simple automation)
Questions for discussion
What are the implications for proprietary data as companies partner with third parties for machine learning solutions?
(777 words)
[1] Dow Chemical Company, “Dow and 1QBit Announce Collaboration Agreement on Quantum Computing.” Dow Global, 21 June 2017, www.dow.com/en-us/news/press-releases/dow-and-1qbit-announce-collaboration-agreement-on-quantum-computing, accessed November 2018
[2] Maxine-Laurie Marshall, “Shell taps into AI to streamline operations and refine customer-centricity.” Fujitsu, August 2017, https://www.i-cio.com/strategy/digitalization/item/shell-taps-into-ai-to-streamline-operations-and-refine-customer-centricity, accessed November 2018
[3] Kim Custeau, “Improve reliability in Chemical manufacturing with predictive asset analytics.” Schneider Electric, 6 February 2018, https://blog.schneider-electric.com/industrial-software/2018/02/06/improve-reliability-chemical-manufacturing-predictive-asset-analytics/, accessed November 2018
[4] Shell, “Shell launches AI-powered service for lubricant customers.” Shell, 4 August 2015, https://www.shell.com/business-customers/lubricants-for-business/news-and-media-releases/2015/artificial-intelligence-powered-service-for-lubricant-customers.html, accessed November 2018
[5] Lyondellbasell, Investor Day presentation 2017, Lyondellbasell, 5 April 2017 https://www.lyondellbasell.com/globalassets/investors/events/2017/170403-2017-lyb-investor-day-full-deck.pdf?id=19745, accessed November 2018
[6] Lyondellbasell, Investor Day presentation 2017, Lyondellbasell, 5 April 2017 https://www.lyondellbasell.com/globalassets/investors/events/2017/170403-2017-lyb-investor-day-full-deck.pdf?id=19745, accessed November 2018
[7] Reuters, “Odebrecht, Lyondellbasell to seal Braskem deal by mid-October.” Reuters, 10 July 2018, https://www.reuters.com/article/us-braskem-lyondell/odebrecht-lyondellbasell-to-seal-braskem-deal-by-mid-october-paper-idUSKBN1K01E7, accessed November 2018
Those are great opportunities indeed. I would argue, however, that the highest potential of machine learning for LYB is in the maintenance space. If they can use the data from sensors such as mechanical seal temperature of pumps or spell back rate of compressors they can unlock huge value in predicting equipment failure. Moreover, they can use the help of ML to chose the turnaround windows based on the season of the slowest demands and perhaps have shorter spread shutdowns instead of one large major turnaround every 5 years or so. The energy market is positioned well to take advantage of ML.
Great article! I agree with Energy that there is a huge potential in developing predictive maintenance solutions as you also described under the “Manufacturing” section of the article. However, I found the application of ML in feedstock selection to be even more interesting. The opportunity for optimizing the differential between raw material and produced good pricing could be a massive savings at newer plants with multiple feedstock capabilities. It seems like right now managers may not necessarily have all the information they need to make the best decision when selecting which feedstocks to use.
Interesting stuff here. Your question – “what are the implications for proprietary data as companies partner with third parties for machine learning solutions?” – is an important one, too.
Recent technological advancements have commoditized machine learning. Now, basically any software developer with a basic understanding of statistics can build machine learning algorithms. Your article and the question paired with it imply that LYB should find expertise externally. Why not in-source the data science? This would protect their data, and potentially build even more of a moat between them and the competition.
Great post, really thought it was provocative. To extend your question, do you think data becomes a commodity that you could sell? Think of Kodak going bankrupt, they surely have a lot of data on camera usage -does Apple pay for that data once AI is able to harness it?
Tom, great article. You really hit the nail on the head with the industry’s use of computer programming to help make the decision in feedstock selection. I unfortunately do not believe this data or program is entirely valuable outside of internal use, because the programs are generally programmed for specific plants and not very scale-able. Overtime, using historic data, the programs can be iterated and improved in predicting the best feedstock, but ultimately human judgement makes the final decision.
To answer your discussion question, I don’t think the data LYB owns is valuable to third parties. I think third parties can be hired to help program more efficient machine automation systems in plants, but their data is best used for internal decision-making.