Lemonade reinvents the insurance industry with machine learning
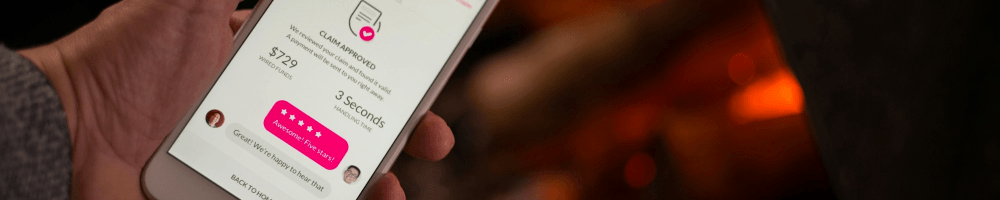
Lemonade, a company offering home insurance policies, is a pioneer in the InsurTech world where its use of machine learning (ML) goes beyond satisfying customers and driving efficiencies to underwriting risks and managing claims
Despite its exponential growth, Lemonade’s scalability is questioned considering increasing customer demand and limitations around availability and precision of data.
Introduction
Lemonade, a company offering home insurance policies, is a pioneer in the InsurTech world where its use of machine learning (ML) goes beyond satisfying customers and driving efficiencies to underwriting risks and managing claims. Viewing Lemonade as a revolution to the insurance industry, investors like SoftBank and Google Ventures backed the company with a $120 million funding [1]. The investors’ trust was strengthened as the company managed to attract more than 14,000 subscribers in eight months only [2].
Figure 1: Lemonade’s growth trajectory [3]
Despite its exponential growth, Lemonade’s scalability is questioned considering increasing customer demand and limitations around availability and precision of data.
Lemonade’s unique business model
Lemonade’s business model is based on a transparent fee model, fast claim settlement, and social good.
The InsurTech company communicates its fee structure with its customers to achieve trustworthiness and transparency; two key attributes missing in the insurance industry.
Collected customer premium is utilized by Lemonade in the following manner:
- 20% flat fee for Lemonade
- 40% for reinsurance to cover major claims
- 40% for claims; any surplus goes to charity
Figure 2: Lemonade’s business model [4]
Apart from its transparent fee model, Lemonade differentiates itself by replacing agents with bots that run insurance processes from end-to-end. However, more than half of Lemonade’s claims are still being handled by humans given their complexity which means that Lemonade still has a long way to go [5].
Use of machine learning to transform the DNA of insurance
Lemonade uses ML to underwrite customer risk and handle claims transforming insurance from “grudge purchase” to a “convenience purchase”. The usage of AI significantly improves processes in insurance as it increases underwriting precision improving premium pricing accuracy, enhances customer experience through fast paper-free processes, and lowers claims costs by reducing fraud.
When issuing insurance policies, Lemonade uses big data to predict risks and quantify losses by placing the customer in a risk group and thereby quoting a relevant premium. These groups of “uniform insurers” share similar risk behaviors and are compiled by AI algorithms that gather extensive customer data and monitor loss ratios (“ratio of claims paid by an insurer to premiums earned” [6]). The more data accumulates, the more recursive risk patterns emerge enabling more precise assessments.
Figure 3: recursion patters forming with data accumulation [7]
Figure 4: improvement of underwriting precision with more data points [8]
In claims settlement, Lemonade’s “AI Jim” assesses the claim by cross-referencing home information, compares it to the customer’s policy, runs fraud algorithms, and finally approves or rejects a claim. The whole process takes up to three minutes and is approved within seconds [8].
Figure 5: claim handled by Lemonade’s AI Jim [9]
Lemonade’s scalability questioned
Lemonade acknowledges that its AI models and infrastructure are not yet equipped to achieve its innovation targets [10]. Improving current models is important, but the company should also have a vision for its expansion plan to new products and geographies.
The following challenges should be addressed for Lemonade to achieve scale:
- Increase in customer demand: increase in claims especially after natural disasters should still be handled in a cost-efficient manner. Will lemonade’s bots and “no-employee” model be able to process all the data as these transactions become more complex? [11]
- Data shortage: Lemonade’s databases are still limited. How will it compete with traditional insurance companies that own extensive data and are already digitizing processes? Will Lemonade’s AI-based underwriting still be relevant? [12]
- Low quality of data: gathered data is based on customer information which could potentially cover factors important in decision making. How can Lemonade ensure its data is accurate, representative, and unbiased? [13]
While the company mentions a medium-term plan to scale, it still does not have a concrete strategy to address the aforementioned issues. Lemonade should consider practical solutions such as acquiring representative external data to train its models, partnering with large insurance companies to handle complex claims, and outsourcing customer service when capacity is exceeded.
Future of machine learning in insurance going forward
As the company thinks about improving its current AI models and prepares to scale, it could explore a wider use of AI across the insurance value chain. Will it be possible to use AI in product development i.e. in customizing policies? Can it use ML and IoT to prevent risks from happening? (800)
[1] Ralph, Oliver. “Financial Times Softbank Takes Stake in US Insurtech Start-up Lemonade”, Financial Times, December 19, 2017, www.ft.com/content/62d7d133-0b69-346d-8c29-6e87848fecbd, accessed November 2018
[2] Alois, JD. “Lemonade Updates on Platform Growth. Adds 14,300 Customers in 8 Months” Crowdfund Insider.com, June 1, 2017, www.crowdfundinsider.com/2017/06/101283-lemonade-updates-platform-growth-adds-14300-customers-8-months/, accessed November 2018
[3] Wininger, Shai. “Lemonade Exposed. Eight Months In. Lemonade Renters & Home Insurance | Protect The Stuff You Love” May 6, 2018, www.lemonade.com/blog/lemonade-exposed-eight-months/, accessed November 2018
[4] Ariely, Dan. “Lemonade’s Social Impact in 100 Days. Lemonade Renters & Home Insurance | Protect The Stuff You Love, Lemonade”, May 3, 2018, www.lemonade.com/blog/lemonade-social-impact-100-days/ , accessed November 2018
[5] Flack, Tom. “Analysis: Lemonade by Numbers.” Insurance Times, August 1, 2017, www.insurancetimes.co.uk/analysis-lemonade-by-numbers/1422155.article
[6] Investopedia. “Loss Ratio.”, June 29, 2018, www.investopedia.com/terms/l/loss-ratio.asp , accessed November 2018
[7] Schreiber, Daniel. “Precision Underwriting. Lemonade Renters & Home Insurance | Protect The Stuff You Love, Lemonade”, August 7, 2018, www.lemonade.com/blog/precision-underwriting , accessed November 2018
[8] Schreiber, Daniel. “Lemonade Sets a New World Record. Lemonade Renters & Home Insurance | Protect The Stuff You Love”, May 2, 2018, www.lemonade.com/blog/lemonade-sets-new-world-record/ , accessed November 2018
[9] Wininger, Shai Wininger.“The Secret Behind Lemonade’s Instant Insurance – Lemonade Stories. Lemonade Stories, Lemonade Stories”, November 23, 2016, https://stories.lemonade.com/the-secret-behind-lemonades-instant-insurance-3129537d661 , accessed November 2018
[10] Gogel, David. “Interview with Daniel Schreiber, CEO and Co-Founder of Lemonade. Medium.com, Medium”, December 20, 2016, https://medium.com/wharton-fintech/interview-with-daniel-schreiber-ceo-and-co-founder-of-lemonade-1a6aef384e2f , accessed November 2018
[11] Accenture. “Machine Learning in Insurance.”, https://www.accenture.com/t20180822T093440Z__w__/us-en/_acnmedia/PDF-84/Accenture-Machine-Leaning-Insurance.pdf , accessed November 2018
[12] Deloitte Digital. “From mystery to mastery: Unlocking the business value of Artificial Intelligence in the insurance industry.”, November 2017, https://www2.deloitte.com/content/dam/Deloitte/de/Documents/Innovation/Artificial-Intelligence-in-Insurance-Whitepaper-deloitte-digital.pd f, accessed November 2018
[13] Balasubramanian, Ramnath. “Insurance 2030–The Impact of AI on the Future of Insurance.” McKinsey & Company, April 2018, www.mckinsey.com/industries/financial-services/our-insights/insurance-2030-the-impact-of-ai-on-the-future-of-insurance , accessed November 2018
Really interesting topic. I’ve used Lemonade and found it easy to use and vastly more transparent then traditional insurance companies. I would be curious of the marginal impact of investing in more data as they should be able to acquire data from the existing customer base as they grow. I would also be curious to see how they stack up against traditional competitors in this space. Glad that you chose to write about Lemonade as I was interested in learning more!
Super interesting topic. My major takeaway question walking away from this is Lemonade achieving cheaper premiums for people and are they taking on the majority of the risk? It seems they are an actual insurance policy so I see an enormous amount of risk with limited data. Insurance works by pooling risk and if it doesn’t have enough data to accurately predict the risk then I’m curious what a natural disaster or exogenous shock would do to its algorithm. My worry would be that it isn’t priced correctly.
I think there is opportunity to build the platform and partner with large insurance firm just for the capital, if nothing else. I imagine the difficulty of forming insurance company is the massive capital investment in order to be able to pay claims.
Fascinating article! You mention two things that are big red flags for me, as I think about the long-term viability of the Lemonade product: data short and data quality. Based on my understanding of machine learning, the models are only as good as the data that you feed them; additionally, the models need to continue to be fed in order to change as consumers, communities, and economies change. If Lemonade is facing data issues, the underlying AI could fail to make good predictions, and ultimately customers could be turned away by overly-high prices or the company could end-up paying too many claims. Resource-strapped start-ups often need to be singularly focused on one strategic issue at a time, and Lemonade needs to focus on both improving its model and acquiring more customers to keep the model working appropriately. I like Lemonade’s mission, so I hope that they’re able to do both well. Wishing Lemonade luck!
Great insights, Petra! I wrote my blog about Allstate’s use of ML for lines of personal insurance, and raised many of the same questions you have. I think while Lemonade certainly appears disruptive in the near term, I question its long term competitive advantage as large incumbents such as Progressive and Allstate embrace ML technologies with much larger data sets and overall scale. I also didn’t know that more than half of Lemonade’s claims are still being handled by humans, which makes me question how advanced Lemonade’s business model truly is. While the speed and social good elements of Lemonade are differentiated, I will be interested to see how Lemonade competes in the future. Perhaps Lemonade would function best a broker that helps connect large, regulated insurance companies with millennials that like the tech-enabled approach of Lemonade, an idea raised by analysts.[1]
[1] William Wilt et al., “Peer-to-Peer Insurance: Proprietary Review of Global Landscape post launch of Lemonade”, Gordon Haskett Research Advisors, December 7, 2016, https://amr.thomsonone.com/, accessed November 2018.
Great article. I was amused at the company’s choice of “Lemonade” as their company name, likely a reference to George Akerlof’s famous market for lemons model, which won him the Nobel Prize for his work in information economics and information asymmetry.
As I read this article, my mind drew parallels with Vitality, an insurance company that uses AI and wearable devices to promote overall health and well-being among its insurers. To my understanding, Lemonade insures homeowners, so I’d be curious if we could solve the data quality issues by providing incentives to Lemonade users to install IoT devices in their homes to enhance accuracy and measurability of their claims.
https://www.vitalitygroup.com/
I agree with MrMayhem’s insight that Lemonade’s best potential value may be in connecting tech-savvy millennials with existing legacy insurance companies, rather than successfully creating an entirely new model of the insurance business. There seems to be a fundamental problem with ensuring that users are honestly submitting accurate claims and not attempting to fleece the system, which could create a vicious cycle in driving up the cost of paying claims and therefore the price of premiums charged. Other tech driven, machine learning companies have some sort of feedback loop for enforcement that is scalable, such as ratings submissions by both parties on Airbnb and Uber. This identifies bad actors in a scaled way. Lemonade relies on a “pledge of honesty” and may be able to audit a sampling of claims, but the business model depends on machine learning accurately validating the veracity of claims.
Further, the legacy insurance business somewhat relies on long processing times for claims to improve their cash conversion cycle as well as reduce the amount of claims ultimately paid out. By delaying processing and putting up high roadblocks and standards to pay claims, it somewhat attempts to weed out frivolous or less-than-truthful claims. Lemonade does the opposite of this, with instant approval and quick processing. While this may appeal to customers and win new users, in the long run it may lead to a spiral of increasing costs and premiums due to adverse selection, meaning bad actors will be especially attracted to the platform to try to make dodgy insurance claims. It’s unclear if the ML technology is sophisticated enough yet to prevent this.
It’s interesting that Lemonade’s product front is a facade for humans running the system in the background — this is a technique common to startups wanting to quickly scale growth but it’s not certain that Lemonade has proven that it can actually transition to ML algorithms eventually instead of humans.
Great article, Petra! My main thought when reading this is how Lemonade can create differentiators and barriers within its machine learning that other companies will not be able to copy-cat effectively. Is there certain data that they are able to gather more accurately? Does their current market give them a unique view? Or is their first-to-market advantage enough to maintain their position?
Awesome research on Lemonade; it’s definitely got some potential to revolutionize the way insurance companies do business. I will say, however, that I am wary about its expansion, especially outside of homeowner’s insurance. Insurance carriers underwrite some pretty complicated policies; homeowner’s insurance is a relatively simple line of business relative to other lines like medical malpractice/worker’s compensation/etc. and has a significant amount of data points to use, given the fact the policy is renewed every year. Does the machine learning have the ability to write more complicated insurance with significantly less data? Furthermore, insurance companies invest heavily in actuarial sciences and predictive data, so I wonder whether they will opt to use this type of technology in-house rather than go with Lemonade.
I like that even the claims processing side can be automated using ML. Traditionally, insurance companies have had to employ hundreds of claims adjusters in order to evaluate claims and reduce the incidence of fraud. However, because Lemonade is removing the cost of hiring claims adjusters, it can probably afford to have a slightly higher incidence of fraud. As Lemonade’s claims algorithm improves and the incidence of fraud is reduced, Lemonade should be able to materially outperform traditional insurance companies.
I wonder, however, about a few things:
1. To what extent is Lemonade’s algorithm unable to underwrite and process more complex and costly claims? Such tail-risks or black swan events (e.g. large hurricanes) could potentially bankrupt the company, especially because doesn’t seem to have investment gains on its float to provide additional income. Therefore, Lemonade’s solvency becomes even more dependent on the accuracy of its underwriting model (i.e. will 80% of premiums always be sufficient to cover claims and reinsurance)
2. As Lemonade gains scale, will it be able to reduce the 20% fee? I could imagine that traditional insurance companies would be able to charge a much lower fee once they move into automated/digital insurance because the would have better economies of scale/lower operating costs to cover.
Very interesting write-up on how Lemonade is using machine learning to fix a broken system. The entire insurance industry consistently frustrates its consumers. The biggest concern I have is the quality of data. Data is the new oil. Lemonade needs to ensure it has a well-structured and actionable strategy with regards to both internal and external data.