Harnessing Informatics to Fight Disease
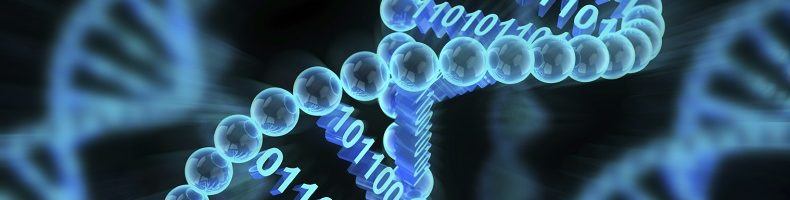
How the drug discovery process is keeping up with technology that outpaces Moore’s Law
It cost $2.7 billion and almost fifteen years to sequence the first whole human genome; that cost has now dropped to approximately $1000 and takes just a few days as a result of enhanced digitization of the genome [1]. The rapid pace of development in sequencing technology suggests that sequencing cost and time will no longer be prohibitive to using genomic data on a large scale. This is good news for pharmaceutical companies who rely upon patient genetic data to guide the selection of potential novel drug targets. However, as Figure 1 suggests, the reduction in sequencing cost and time has merely moved the discovery bottleneck downstream; regardless of how fast a genome is sequenced, it must be processed before finally being interpreted by a scientist [2]. Pharmaceutical companies are integrating collaborations with external technology companies into their operating models in order to shorten analytical timelines.
Figure 1
AstraZeneca initiated a collaboration with Bina Technologies in 2015 in order to develop faster and more scalable NGS (Next Generation Sequencing) processing capabilities. Bina’s RAVE software platform enabled AstraZeneca to cut their deep sequencing process timelines for exome (protein coding DNA sequences) sequencing from 16 hours down to 45 minutes, and whole genome (protein coding and noncoding DNA sequences) sequencing from 72 hours down to 6 hours [3]. The data processing steps also rely upon a set of 331 NGS tools, the majority of which are developed in academia and are updated approximately every month; each tool also has its own set of adaptable command lines. Bina’s ability to update, validate, and quality check each of these tools as a part of their Genomic Management Solution provides immense value [3].
However, processing large amounts of data quickly means nothing if the results do not allow for the extraction of key pieces of biological insight. Therefore, in addition to improving the speed at which genomic data is processed, AstraZeneca has also focused its efforts on improving the algorithms used in the processing steps. Commonly used variant callers miss complex genetic mutations, including large genomic insertions and deletions. Additionally, they do not perform well with ultra-deep sequencing which is necessary for detecting genetic variants in circulating tumor DNA. AstraZeneca has remedied these issues through the development of VarDict, a novel variant caller [4].
Given the rapid pace of development in genomics technology and the increasing data storage and analytics requirements, AstraZeneca has turned to Amazon Web Services (AWS). AWS has enabled AstraZeneca to build a private cloud. The Bina platforms have been integrated into the cloud, as well as high-performance computing clusters [3].
Finally, all of this data would be useless without scientists who can interpret it. However, mining the raw data files that are generated after processing requires a particular skillset; a whole-genome sequencing data file for a single patient is on the order of 102 GB in size. Analysis of these files requires an understanding of both disease biology and programming/big data analytics, a marriage of skills that is not abundant enough in the research community. In order to facilitate the exploration of large datasets by biologists, AstraZeneca has spearheaded a “Bioinformatics for the Bench” development initiative. In order to deliver this, AstraZeneca collaborated with Bina Technologies on using their Annotation and Analytics Intelligence Module Software (AAiM) to provide biologists with software and guided user interfaces that make large genomic datasets more accessible and interpretable [3].
The dramatic increase in availability of genomic data has provided opportunities for AstraZeneca to shift its business strategy towards personalized healthcare. For example, after the failure of olaparib in 2011 and 2012 clinical trials in triple-negative breast and ovarian cancers, AstraZeneca halted development of the drug [5]. However, further exploration of the biology aided by genomic datasets of patients in these trials led to the conclusion that ovarian cancer patients carrying BRCA mutations responded well. AstraZeneca subsequently achieved olaparib approval in this patient population, and was the first company to release a drug with a companion diagnostic to identify appropriate patients [6]. Moving forwards, and with a general push in the healthcare space towards outcomes, AstraZeneca will be able to harness the increasing amounts of genetic data to understand which patients will benefit from a given therapy.
In addition to the initiatives previously discussed, I think there is opportunity for AstraZeneca to further explore collaborations with technology companies focused on healthcare. Specifically, integrating genomic data with clinical metadata that might be easily collected through the Apple ResearchKit may provide avenues to explore a systems biology approach to understanding disease and identifying drug targets. Flatiron Health also provides a unique opportunity to integrate clinical and genomic data [7]. Additionally, working with experts in machine learning and data analytics at tech companies such as Google to understand novel methods of analyzing big data may provide enhanced insights.
Word Count: 797
References
[1] Tirrell, Meg. Unlocking my genome: Was it worth it? December 2015. <http://www.cnbc.com/2015/12/10/unlocking-my-genome-was-it-worth-it.html>.
[2] BM Good, BJ Ainscough, JF McMichael, AI Su, and OL Griffith. “Organizing knowledge to enable personalization of medicine in cancer.” Genome Biology 15 (2014): 438.
[3] Bina Technologies. Solving NGS Bottlenecks with a Globally Distributed Genomic Data Management Solution. June 2015. <http://blog.bina.com/read/solving-ngs-bottlenecks-with-a-globally-distributed-genomic-data-management-solution>.
[4] ZL Lai, A Markovets, M Ahdesmaki, B Chapman, O Hofmann, R McEwen, J Johnson, B Dougherty, JC Barrett, and JR Dry. “VarDict: a novel and versatile variant caller for next-generation sequencing in cancer research.” Nucleic Acids Research (2016).
[5] Garber, Ken. “PARP inhibitors bounce back.” Nature Reviews Drug Discovery (2013): 725-727.
[6] FDA. FDA approves Lynparza to treat advanced ovarian cancer. December 2014. <http://www.fda.gov/NewsEvents/Newsroom/PressAnnouncements/ucm427554.htm>.
[7] Flatiron Health. n.d. <https://flatiron.com/>.
Cover Image Source
http://www.thinkcx.co.uk/wp-content/uploads/2014/07/DigtalDNA.jpg
AstraZeneca’s customer promise of developing innovative genetic sequencing approaches that are fast and usable hold enormous potential applications in healthcare. Issues with data storage as you outlined are indeed serious ones that the company needs to address. AstraZeneca should also pay attention to privacy and cyber-security. As genetic sequencing becomes readily available and prevalent, there is a risk of predicting health status and outcomes, which could influence how insurance companies evaluate risk and manage healthcare, making patient privacy and cyber-security very important. Companies pushing the frontiers of genetic engineering therefore need to innovate, but not without considering the social implications of innovation.
I think the most compelling piece of your article is the fact that AstraZeneca can now provide a cure and a diagnostic method for doctors and patients alike. Cancer treatment is very personalized and each patient receives a different cocktail of chemotherapy drugs but still so much of that customization process remains a series of trials and errors. This genetic sequencing technology could allow for a much larger degree of precision and help millions of cancer patients identify and eradicate their particular diseases.
Very interesting post. With some big data advancements (Hadoop, Spark) happening only within the past few years, there are likely huge gains to be made in computational biology in the next few years, especially if the ability to analyze such large data sets more quickly is feasible.
I also wonder how big pharma will respond. You suggest that they collaborate with these healthcare technology companies. What not buy them? Big pharma buys small biotech companies to expand or extend their drug pipelines, particularly if it allows them to build a capability in a new therapeutically. Similarly, pharmacos should look to buy genomics companies if they strengthen their drug discovery capabilities.