Commoditizing Crowdsourcing; How a Distributed Network Helped Build a Business
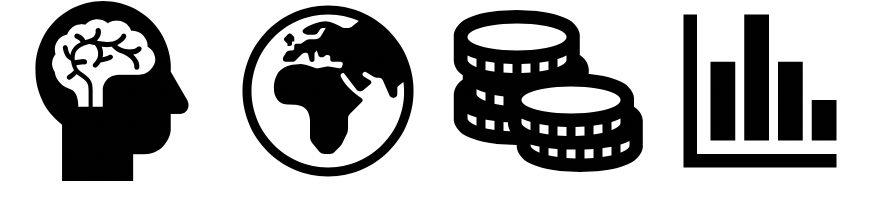
Premise uses distributed data collection to gather valuable information for customers ranging from governments to businesses.
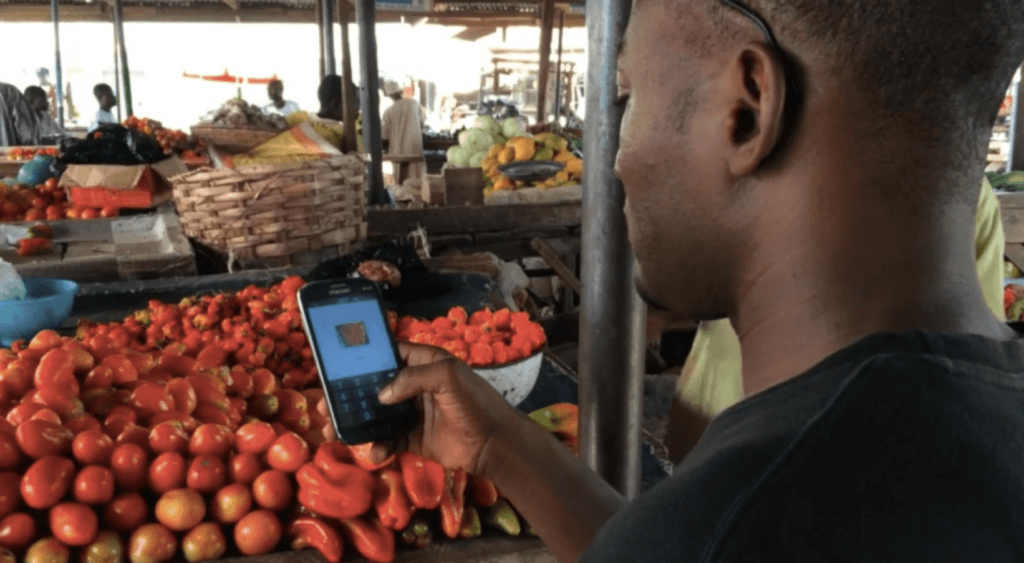
In order to fuel advances in machine learning, AI, and predictive analytics, companies are after the same scarce resource; high quality data, in aggressively large quantities [1]. Without accurate data, algorithms driving process and strategy improvements are rendered useless. Premise serves to bridge this gap by crowdsourcing data collection, creating a company built through the power of distributed innovation.
Premise employs a smartphone application to allow anyone, anywhere, to collect data on a variety of different phenomenon [2]. Through its network of more than 80,000 contributors in over 30 countries across the world, Premise collects information on things like the price of goods in local markets, the conditions of public parks and pathways, or even the quality of health clinics [3, 4, 5, 6]. Contributors receive instructions through the application, and upload photos and text to document local conditions in exchange for small payments [3].
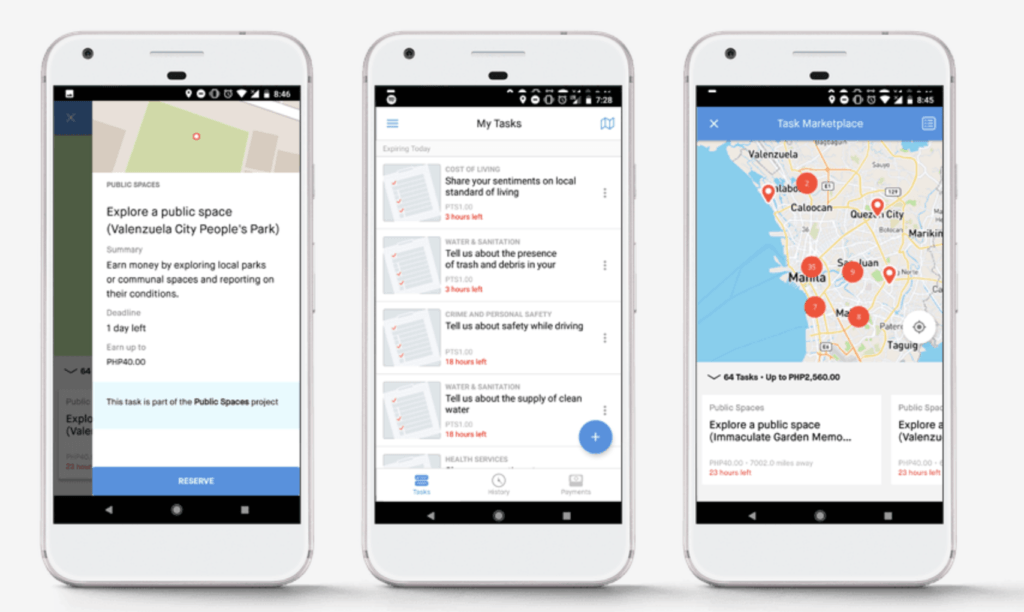
Clients, ranging from businesses and banks to non-profits and governments, then use this information to create everything from real time price indexes gauging inflation, to adherence to government policy [4].
The data is particularly valuable because it is often inaccessible and costly to gather. Instead of relying on traditional methods like surveys, or site visits, organizations can have a constant finger on the pulse of rapidly changing conditions around the globe. In essence, Premise has commoditized crowdsourcing, effectively leveraging open innovation as a business model.
Diving into Crowdsourcing
Crowdsourcing as a gateway to untapped resources is not a new concept. The model has been used to generate creative content and solutions for companies across many industries, from mining to advertising [7]. Is this model of crowdsourcing data collection then particularly defensible, and does it meet the growing needs of organizations looking for quality data, free of bias? To address these issues, Premise has implemented several strategic controls rooted in machine learning that help eliminate common concerns around open innovation models [8].
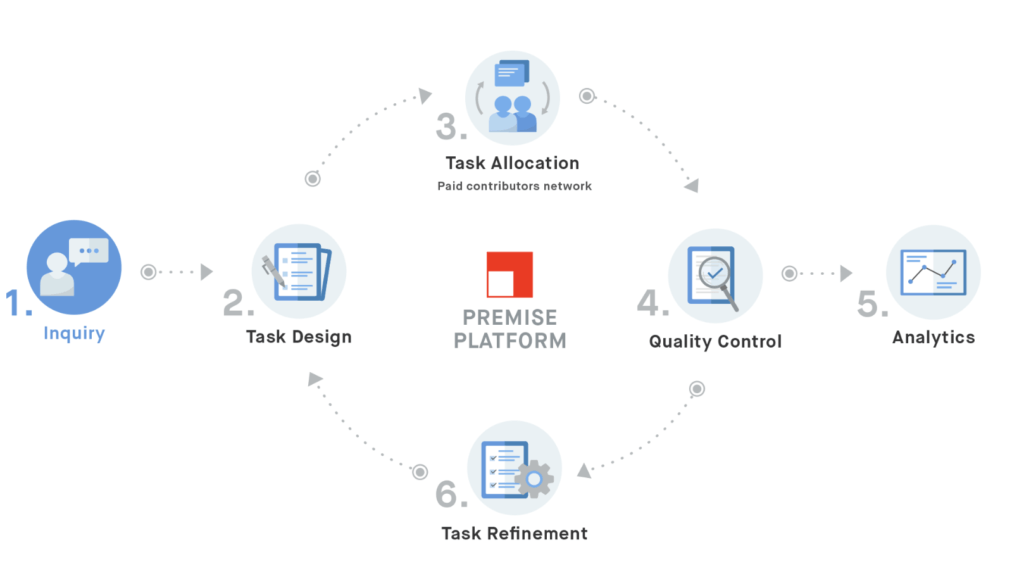
Defensible:
The difference in implementation lies in the locus of control. While social media and other open source datasets are valuable and have been proven to help with critical situational awareness in disasters or catastrophic events, they are ultimately a passive vehicle of data collection [9]. By constantly directing their contributors, Premise is able to control the flow of collection, and hone in on specific areas of focus for their customers [10]. Thus the data is not only less noisy, but also much more flexible. The company can adjust their metrics immediately, depending on the needs of the customer or identified weaknesses in the current dataset. Instead of being beholden to collecting and analyzing only the data that surfaces, Premise can seek out and provide access to new datasets that would otherwise remain hidden. This control separates them from more traditional applications of distributed data collection.
Bias:
Part of the issue with distributed data collection at scale, is that it becomes difficult to verify, particularly with the added complication of financial incentives. The quality of the data is of paramount importance, and as a result Premise needs to focus on both scaling the collection of data, and also the review and verification of their resultant dataset.
In order to do this, Premise uses a variety of technological tools including timestamps, GPS tracking, and algorithms that can help limit fraud [8]. The review process ensures that every data point is actually coming from consumers in the target markets, and that it matches other inflows in the system [8].
Another potential point of bias is the composition of the network of contributors. If the contributors act substantially different from the local market, then the data could be flawed. Again, the fact that Premise controls the collection of data helps to alleviate this bias, because the individual preferences of the contributors matter very little in terms of the type of data that is collected. Moreover, studies have demonstrated that even in fields like healthcare where individual characteristics play a more important role, crowdsourcing can be just as reliable and accurate as medical surveys and research [10].
What’s Next?
Moving forward it would also be interesting to see the crowdsourcing model expanded beyond a limited range of inputs. Information such as ethnographic interviews and usability testing are valuable data points that remain difficult to aggregate at scale. If Premise could incorporate this type of outreach, their position would be even more defensible and the appetite for these difficult datasets could drive further customer expansion.
Questions around payment for crowdsourcing contributors remain, both in the context of data collection at Premise, and the ever-evolving model of open innovation. Is it fair to take advantage of the lower costs of distributed labor and what role do financial incentives play in production? Finally, what protections are in place to ensure that workers in a gig economy are adequately compensated?
(798 words)
References:
[1] Bean, Randy. “How Big Data Is Empowering AI and Machine Learning at Scale.” MIT Sloan Management Review, May 8, 2017. https://sloanreview.mit.edu/article/how-big-data-is-empowering-ai-and-machine-learning-at-scale/, accessed November 11, 2018.
[2] Premise Data Corporation. “Product Overview.” https://www.premise.com/products/, accessed November 12, 2018.
[3] Shueh, Jason. “Under new CEO, Premise brings crowdsourced research to government.” Statescoop, February 7, 2018. https://statescoop.com/under-new-ceo-premise-brings-crowdsourced-research-to-government/, accessed November 12, 2018.
[4] Captain, Sean. “Premise Pays App Users To Gather Real-World Economic Data.” Fast Company, September 24, 2015. https://www.fastcompany.com/3051471/premise-pays-app-users-to-gather-real-world-economic-data, accessed November 12, 2018.
[5] Premise Data Corporation. “Premise Data Selected by the City of Waco, Texas to Gather Real-Time Resident Sentiment for Data-Driven City Management.” press release, September 15, 2018. PR Newswire, https://www.prnewswire.com/news-releases/premise-data-selected-by-the-city-of-waco-texas-to-gather-real-time-resident-sentiment-for-data-driven-city-management-300717889.html, accessed November 2018.
[6] Premise Data Corporation. “Premise Data Tapped to Collect Ground Truth on Health Facilities Worldwide.” press release, September 5, 2018. PR Newswire, https://www.prnewswire.com/news-releases/premise-data-tapped-to-collect-ground-truth-on-health-facilities-worldwide-300706704.html, accessed November 2018.
[7] Brabham, Darren C. “Crowdsourcing as a Model for Problem Solving: An Introduction and Cases.” Convergence, Vol. 14, No. 1 (February 2008): 75-90. SAGE Journals, accessed November 2018.
[8] Baker, David. “Photos are creating a real-time food-price index.” Wired, April 5, 2016. https://www.wired.co.uk/article/premise-app-food-tracking-brazil-philippines, accessed November 12, 2018.
[9] Yin, Jie, Andrew Lampert, Mark Cameron, Bella Robinson, and Robert Power. “Using Social Media to Enhance Emergency Situation Awareness.” IEEE Intelligent Systems, Vol. 27, No. 6 (November-December 2012): 52-59. IEEE Xplore Digital Library via ProQuest, accessed November 2018.
[10] Loizos, Connie. “Premise Raises $50 Million To Outsource The Collection Of Economic Data.” Techcrunch, September 24, 2015. https://techcrunch.com/2015/09/24/premise-raises-50-million-to-outsource-the-collection-of-economic-data/, accessed November 12, 2018.
[11] Mortensen, K., and T.L. Hughes. “Comparing Amazon’s Mechanical Turk Platform to Conventional Data Collection Methods in the Health and Medical Research Literature.” Journal of General Internal Medicine, Vol. 33, No. 4 (April 2018): 533-538. ABI/INFORM via ProQuest, accessed November 2018.
This was a very thought-provoking piece, there must be opportunities for this type of data collection in almost every industry. In investment management, I could imagine many firms being willing to pay for a unique, nonpublic set of data on companies or industries of interest in order to gain an information advantage on the market. Although some bias may still exist, I imagine this data would be much more representative than current sources like social media, where individual sentiment or emotion can impact the willingness to provide data and/or distort the reality.
A very cool idea, that should be able to play a really interesting role in health care and delivery and how it compares to classic epidemiological collection techniques. I can see really interesting future roles for examining the spread of disease (e.g. flu or other infectious agents), as long as quality control can remain high. The big question will ultimately be user adoption, but in instances where the payment is fair, I can see this taking off – especially in underdeveloped communities.
Fascinating concept for collecting the data that feeds machine learning – thanks for researching! I would be very interested to know what demographic information they collect from users and how that factors into their creation of target markets and efforts to eliminate bias. Do they use demographic characteristics (socio-economic status, religious identification, race, etc) to construct these markets and/or treat them as possible sources/determinants of biases (conscious or unconscious)? It seems like this kind of data would need to be included and considered in their processes in either case, but this in turn leads to additional security, privacy, and regulatory considerations.