American Express has Struck Gold with Machine Learning
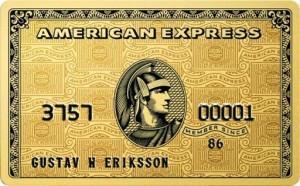
American Express, a company which has been around for over 150 years, moves into the next generation harnessing the power of machine learning.
American Express (“Amex”) is an issuer processor, network and merchant acquirer. Their revenues are derived from three primary sources: annual card fees; interest income on late payments; and merchant and network fees on each transaction.[1] Amex has become one of the most profitable and prestigious companies in the card market globally. Yet, they still have much untapped potential, which can be unleashing from harnessing the power of machine learning.
Over the past 150 years, they have accumulated data from transactions that have been made on their cards. Given that in 2017 alone, they processed $1.1 trillion worth of transactions and had 112.8 billion cards in force, the volume of data they possess is unparalleled.[1] Moreover, as the card market is one in which trust, customer service and risk systems are paramount, effective use of this data through machine learning presents tangible upside to the company’s performance. In this short essay, I will discuss a few key issues inherent in this market, which can drive the profitability of Amex if handled effectively: A) fraud detection; B) customer acquisition; and C) customer service.
The first use case we shall discuss is fraud detection. The incidence of credit card fraud is on the rise, with scammers getting increasingly smart and creative in their thieving ways.[2] An individual makes thousands of transactions a year, how can we instantaneously stop those which are fraudulent? Using machine learning, the management of Amex is aiming to detect and prevent fraudulent transactions as they are taking place, whilst allowing all normal transactions to go through.[3] Given the vast data set of historic transaction information on an individual and similar individuals, they can recognize spending patterns – Where do customers spend? What do customers spend on? Predictive technology can be used to indicate whether all future transactions are considered normal or abnormal. In the latter case, anomalies can be prevented and investigated further, avoiding potential substantial losses to scammers.
In the medium term, new customer acquisition will be crucial in sustaining a successful business. Amex has engaged in targeted marketing through machine learning models.[3] This allows them to identify potential customers and place them in dynamic groups based on interest variables derived from their online interactions. This information allows Amex to identify which features individuals value most and to accurately target certain groups with certain products (i.e Individual X travels a lot, we should direct them to our zero foreign transaction fee card).[4] This will not only increase demand for Amex cards, but also reduce acquisition costs which have historically been high due to direct mail campaigns.[3]
The final use case is to drive customer satisfaction through their recommendation model.[3] By using the transaction history and profile data of an individual, Amex can predict which restaurants an individual will like and make customized restaurant recommendations accordingly. This will improve a customer’s experience (hopefully reducing the churn rate), and also has potential to drive further transactions and create advertising revenue.
But why stop there? Amex can further harness the potency of machine learning in the short and medium term. Amex can take the lessons learned from improving the new customer acquisition process to develop new products. By combining existing transaction data with the dynamic grouping of targeted marketing, Amex can truly understand the needs of its current and future customers. This will allow them to predict what exact features each of the distinct customer segments desire and create products accordingly.
They can also further augment the customer service with other machine learning use cases. Amex can develop methods to accurately preempt customers’ questions and answer them before they have to call customer support for help. By tracking the way customers generally navigate through their website or mobile application before they reach out with their issues, Amex will be able to develop a help page which pushes the most likely issue a customer is facing. Other financial institutions have reached a 53% accuracy in determining the top 3 potential issues a customer may have.[5] Given the vast data set available, Amex is likely to generate similar if not better outcomes.
Overall, this short essay brushes the surface of the potential use cases of machine learning in this industry. Exploiting all potential avenues of this megatrend, Amex can benefit substantially through the generation of greater card and transaction fees, lower costs and lower loan loss provisions.
A couple of open questions related to machine learning in this context would be:
- How can you ensure that customers appreciate the customized recommendations without them assuming that Amex is exploiting their data, especially when it can relate to rather personal expenses?
- How can you accurately segment customers into groups in order to more effectively predict outcomes? (i.e a transaction in Tajikistan may be normal for one group but not for another)
(797 words)
Citations
[1] American Express 2017 Annual Report
[2] https://www.businessinsider.com/credit-card-fraud-scam-what-to-do-2018-8
[3] https://www.linkedin.com/pulse/case-study-machine-learning-american-express-mats-uddenfeldt/
[4] https://www.forbes.com/sites/mariyayao/2018/04/10/14-ways-machine-learning-can-boost-your-marketing/#15634be11b6d
[5] https://monzo.com/blog/2017/05/03/practical-machine-learning-for-startups/
Thanks PM for the post! My initial reaction was the ethical worries of machine learning using AMEX’s vast sum of data. I would imagine a user being concerned about AMEX looking through his/her purchases, which tend to be privately held. I would be interested to know what precautions AMEX is taking to avoid a Facebook-esque falling out with consumers as it tries to advance it’s big data usage. I think the issue of fraud detection is a very important one as more and more transactions move online making financial data even more important to protect and fraud harder to detect. Does AMEX work with other security companies to help with fraud detection or are the algorithms created from the inside? I’d also be interested to see if machine learning can help in predicting when fraud will happen, making fraud prevention more of proactive and less reactive.
Thanks for the post! Credit card companies have a huge source of data, and I believe they can learn a lot (even maybe too much) about an individual through data science.
I think the point you touched on credit card fraud is especially important. To augment on the issue – in 2018, it is expected that total losses due to credit card fraud would amount to $19B globally (https://www.nasdaq.com/article/credit-card-fraud-and-id-theft-statistics-cm520388). Considering the amount of money on the table, it is not surprising Amex is putting R&D efforts to tackle that issue head on.
I also believe this is an issue to be handled on the long term – as time passes by, more and more transactions move from the physical world to the digital. Thus, people are more susceptible to digital identity theft and fraud – and that must be stopped.
Thanks for the post! It is no surprise that credit card companies including American express are focussing on how to upsell to customer and make more ‘smart products’. On the credit card fraud issue, I am interested to understand how far along is American express in predictive analysis of fraud detection and how far behind are it’s competitors. In terms of upselling products through data there is huge potential of strategic tie ups with 3rd parties that give more targeted incentives to the customer than the normal points system. That way, customers can actually get a true customized version of their of cards rather than having to chose from a selected few options. However, how that can be achieved from an operations and regulatory perspective remains to be seen.
Very interesting article. I was thinking about your first question as I was going through, and I think It would be close to impossible for American Express to recommend products to customers without raising issues of privacy and authenticity. I think about how I uncomfortable feel when Facebook, Instagram or generally on Google I receive a recommendation on a product to buy that was related to something I had spoken to from a friend without actually searching for it. I think with a credit card, when I know the company would be intimately familiar with my spending, the last thing I would like to see is recommendations. I feel it’s at the same time deeply invading and highly uncomfortable.
However, to your second question, the company can and should certainly use their own data to better segment the customers. As it’s internal, and you can curate the services and marketing messages to a specific group of people, it would create the same visceral reaction as the former example I had mentioned. It do think it’s crucial for the company to strive to present its marketing message as a place of authenticity as opposed to a place where customers may feel like their privacy is invaded.
Really enjoyed reading this. I do think that the use of machine learning in fraud prevention could be effective, but it could also have pitfalls. My card has been suspended while I am travelling, causing enormous inconvenience and denying me access to credit while overseas. I could see this happening with a machine learning tool that observes transactions occurring in a different jurisdiction from the client’s home. Moreover, given the increasing sophistication of hackers, it is not inconceivable that hackers could somehow create fraudulent transaction data on which to train the tool, thereby allowing fraudulent charges to go through undetected. These challenges aside, I do think the implications of machine learning in fraud prevention will be immense, both in the credit card industry and beyond.
Thanks PM for the post – I’m very interested in the relationship of consumer behavior and data, so this was really exciting to read. I think there is an interesting line that American Express has to watch with customized recommendations. Eventually these recommendations will transition from being perceived as helpful to being perceived as “creepy”, and I’m curious to see when (or if) that happens. The public has become so accustomed to extremely targeted ads and perhaps this will just be another example of that. How can you ensure this doesn’t happen? Some randomness in the targeting might help, or simply training your audience about how you decide what to show them. It’s an interesting problem to explore.
Very interesting read! I think the data American Express collects can be used even further beyond the cases you described here. Credit card purchase data is incredibly valuable as it is itemized and has a location and a time stamp associated with it. Using this granular data and machine learning could yield powerful customer profiles that any B2C company would love to get their hands on. The key question is under what circumstances if at all American Express is allowed to share that data with others vs. keeping it for their internal uses only.
Super interesting article! As I was reading your article, the first open question you posed loomed in my head throughout. How can you provide customization and recommendation to a level that is commensurate with consumers’ individual privacy. One way to circumvent this potential breach is to segment the types of recommendation by level of sensitivity. Some types of recommendations should never be shown to any consumers. Accordingly, customers should also be segmented by their spending behaviour. If they are able to build general profiles of people and cross that with types of recommendations, they could mitigate privacy risk.
Representing the unpopular opinion, I believe that personalization is an incredible value add of the digital age. No one likes advertisements but if I have to watch them, I prefer for them to be about products and topics that I care about. In the context of AMEX, I believe that consumers will equally appreciate a personal ‘concierge’ like service, as long as they can explicitly opt into the service and they don’t become the product themselves. AMEX needs to resists to follow advertising giants like Google and Facebook and put the interests of the consumer first. If they can accomplish a clear partnership with consumers in the mind of the end-user personalized recommendations will unlock incredible transaction revenues.
Great job! I think your first question is a very important one: how can companies reconcile AI with customers’ privacy concerns? I know that Google originally got in lots of hot water upon the debut of advertising in GMail when people thought it was reading their emails, despite the fact that computers need to read the text to render it anyways. In Amex’s case, I think a subtle approach will be best. They have a very engaged cardholder rewards community, so I think that offering suggestions as a new “perk” offering (rather than as apparent advertising) will be a very efficient channel for driving customer belief. Customer suggestions are a product for which packaging and context is everything. With the right context, personalized suggestions will be a powerful product.