Can Machine Learning predict, prevent and diagnose Diabetes effectively?
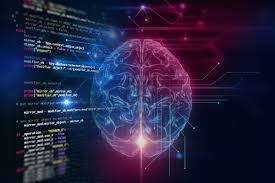
Diabetes is one of the fastest growing disorders affecting population across the globe, where diagnosis remains as big a challenge as treatment. Will Machine Learning enable healthcare companies to develop an effective disease management solution?
Why do you think the megatrend you selected is important to your organization’s management of process improvement and / or product development?
“Clearly rice consumption in Kerala has led to maximum prevalence of Diabetes[1] ” remarked the marketing manager, Abbott Diabetes Care business in India, as he looked at the reports prepared by an in-house analytics team [2] using Machine Learning (ML).
The results meant huge potential for Abbott, that had a continuum of care products in Diabetes accounting for over $1.5bn of sales globally and a growth of 33%. In India, Diabetes products accounted for nearly $300mm of annual sales.[3]
These insights reinforced the power of ML in healthcare to identify critical factors in disease management. Diabetes has about 422mm patients globally in 2014 and seventh leading cause of death in 2016, as per WHO. In India, 72mm cases were recorded in 2017, the figure expected to nearly double by 2025.[4]
Extensive research in all aspects of diabetes has led to the generation of huge amounts of data, making the disease a great fit for applying ML to find better solutions and improve outcomes.[5] A dual approach of developing inhouse capability and forging external partnership has enabled Abbott to take advantage of the new trend.
What is organizations management doing to address this issue in the short term (next two years) and the medium term (two to ten years out)?
In the short term, Abbott is leading the development of “smart” products that can interact with patients in real time and generate high quality patient data. Some of the products launched are as mentioned below: [6]
- FreeStyleLibre system: It consists of a small, disposable sensor placed under the skin and worn on the back of the arm for 14 days
- FreeStyle Libre reader: A handheld device, that scans the sensor to instantly obtain current glucose levels and historical patterns and trends
- LibreLink: A mobile app that enables a compatible Android phone to scan the FreeStyle Libre sensor
- LibreLinkUp: An app that allows caregivers to receive notifications when a user scans the sensors
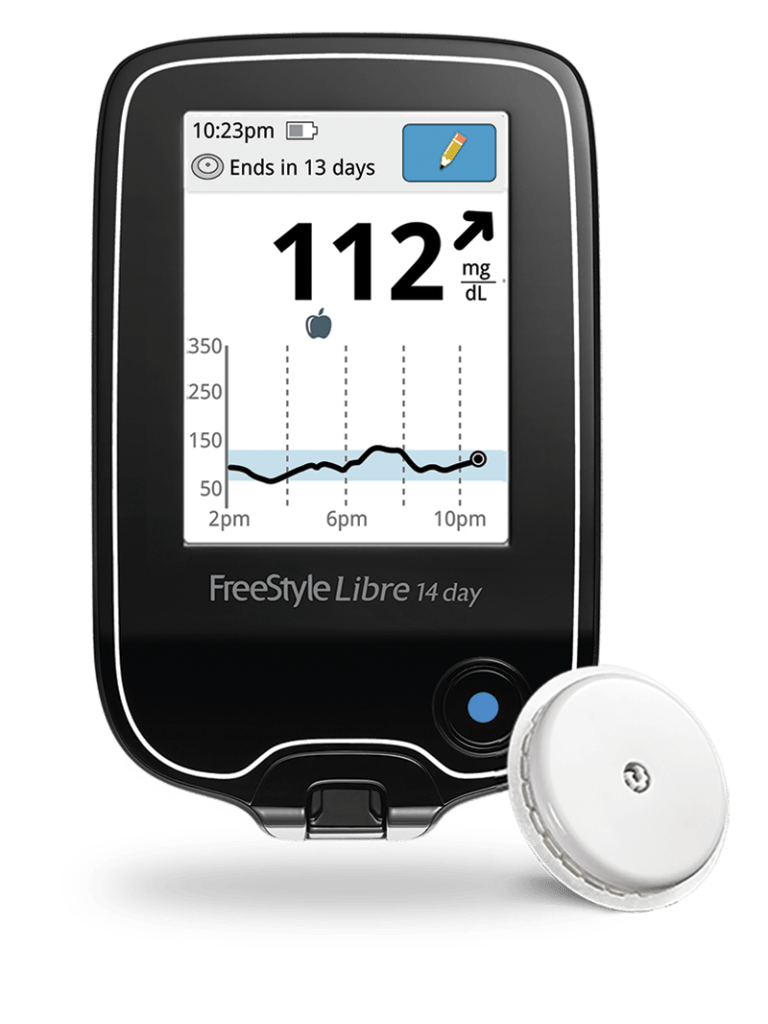
To capture the data produced, Abbott is seeking required patient and regulatory approval. In Europe, Abbott sought permission from its patients scanning their sensors for accessing their data through a cloud-based service to help guide treatment decisions. To date, more than 50,000 people who used the system from 2014 and 2016 granted Abbott access, yielding more than 409.4mm glucose measurements, 86.4mm monitoring hours and 63.8mm scans.
In the long term, Abbott plans to use ML for process improvement of the continuum of care by intervention at all stages of Diabetes management.
- Disease prediction: Abbott plans to support world-wide studies using ML to improve accuracy of heart risk prediction within a large population.[7]
- Disease prevention: Abbott is exploring partnerships with companies that leverage ML from smartphones to help patients – under a physician’s guidance – make better decisions on diet, medications and other health-related issues[8].
- Disease management: Abbott is evaluating partnerships with tech-giants to co-develop apps that would use voice-enabled software ( e.g. Amazon’s Alexa) to help diabetic patients manage disease at home – including lifestyle modification, dietary checks or pill reminders[9].
What other steps do you recommend the organization’s management take to address this issue in the short and medium term?
In India, diagnosis of Diabetes is 55% and the adherence to medicine is 40%. In the short term, Abbott can use ML to drive improvement in diagnosis and medicine adherence for Diabetes.
- Targeted diagnosis campaigns: Using ML, identify regions with higher distribution of diabetes population and conduct targeted campaigns or educational programs
- Identify associated factors: Identify related markers indicating onset of Diabetes such as chronic kidney disease, cardio vascular complications and eye-complications to proactively manage Diabetes.
- Pill plus models for active diabetes patients: Make the LibreLink App smarter integrating with mobile phone and setting reminders for pill consumption / routine checkups.
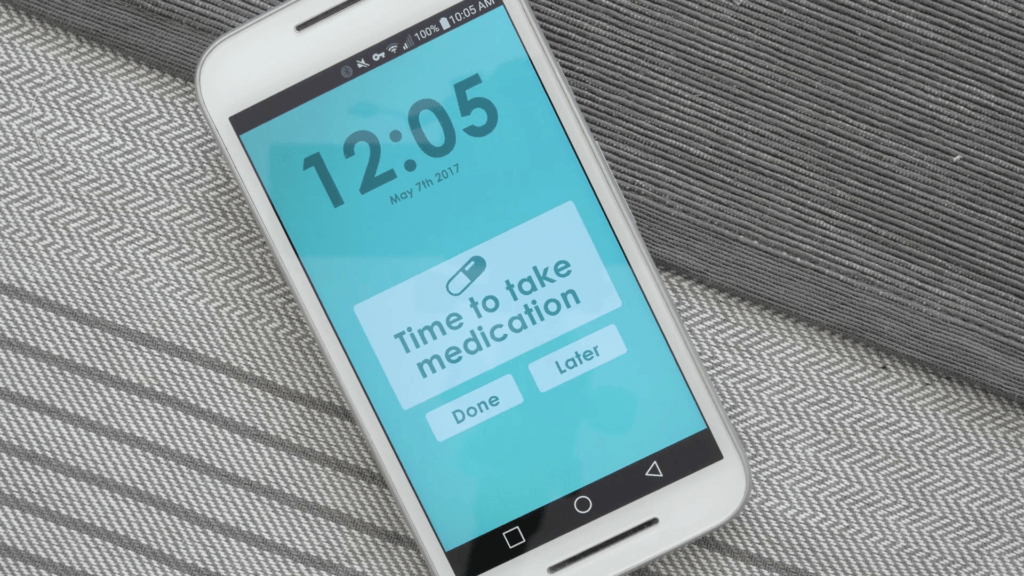
Medium terms
- Integration with mobile health apps such as Fitbit to provide recommendations on lifestyle management
- ML coupled with big data to be used in improving outcomes of drug-discovery or product enhancement for rare diseases such as oncology.
- With large sales force, ML can be used to improve the internal reporting and control of salesforce. ML can also be utilized to better manage supply demand planning for critical drugs that require expensive cold storage.
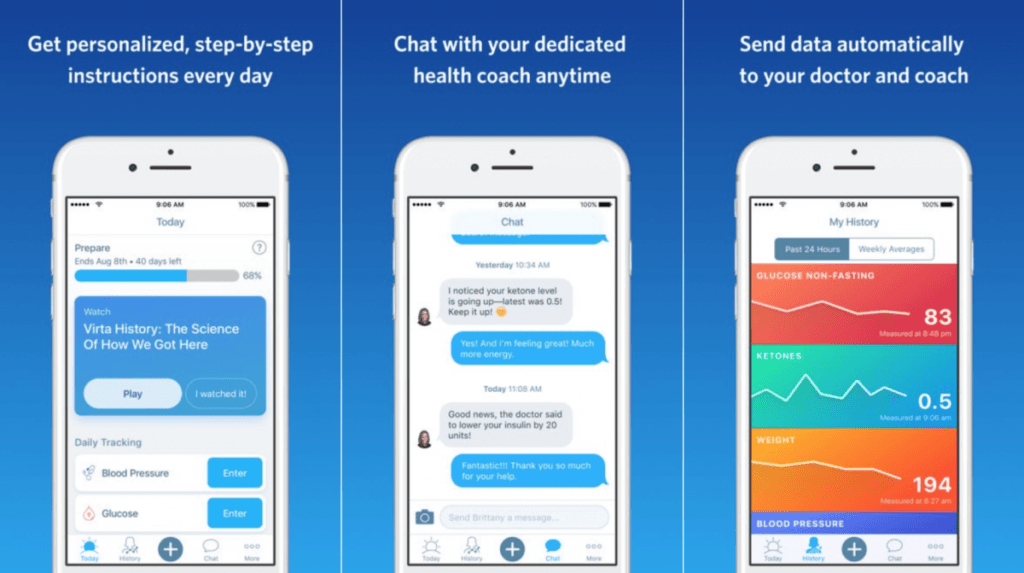
In the context of this organization, what are the one or two important open questions related to this issue that that you are unsure about that merits comments from your classmates?
- How to reduce dependence on human intervention and remove initial biases? ML is still dependent on human input as a human “trains” a system with data and categorizes a good pattern versus an inaccurate pattern.
- How to improve knowledge of limited variable? Scientists don’t know all of the components / unknown variables that affects disease manifestation / progresses.
- How to address concerns around privacy and regulations? Data access is an important input for success of ML, however regulations around patient data safety could be a potential hindrance.
- References
- Diabetes – Symptoms And Causes”. 2018. Mayo Clinic. https://www.mayoclinic.org/diseases-conditions/diabetes/symptoms-causes/syc-20371444.
- “Kerala Has High Rate Of Diabetes Incidence”. 2018. The Hindu. https://www.thehindu.com/news/cities/Thiruvananthapuram/kerala-has-high-rate-of-diabetes-incidence/article8457105.ece.
- “Abbott Reports Fourth-Quarter 2017 Results”. 2018. Prnewswire.Com. https://www.prnewswire.com/news-releases/abbott-reports-fourth-quarter-2017-results-300587414.html.
- “Diabetes”. 2018. World Health Organization. http://www.who.int/news-room/fact-sheets/detail/diabetes.
- “Big Pharma Outlook Webinar | Pharma Intelligence”. 2018. Pharmaintelligence.Informa.Com. https://pharmaintelligence.informa.com/resources/product-content/artificial-intelligence-and-future-health-care-innovation
- “Continuous Glucose Monitoring System”. 2018. Freestylelibre.Us. https://www.freestylelibre.us/.
- “Can machine-learning improve cardiovascular risk prediction using routine clinical data?” https://journals.plos.org/plosone/article?id=10.1371/journal.pone.0174944
- “Big Pharma Outlook Webinar | Pharma Intelligence”. 2018. Pharmaintelligence.Informa.Com. https://pharmaintelligence.informa.com/resources/product-content/artificial-intelligence-and-future-health-care-innovation
- “Big Pharma Outlook Webinar | Pharma Intelligence”. 2018. Pharmaintelligence.Informa.Com. https://pharmaintelligence.informa.com/resources/product-content/artificial-intelligence-and-future-health-care-innovation
Per your second question, I think this is precisely the limitation of machine learning. While I agree that ML has a place in medicine – including in regulating diabetes – I don’t think it will ever fully replace provider interaction. The provider (e.g., nurse, physician, etc.) is able to look beyond the test results and really get to the root of the problem, whether that is physical, psychological, genetic, or something else. The provider can process unknowns, which is something machine learning is not able to do as of now. Furthermore, medicine changes quickly, and I’m skeptical whether machine learning will be able to keep up with the rapid changes.
While machine learning is frequently discussed in the context of patient diagnosis and treatment across countless indications, given the scale I think diabetes will be a particularly important proving ground for the future of ML in healthcare. As you point out, the sheer prevalence is massive, and the problem is only getting worse. Abbott’s FreeStyle Libre and the data it collects is a great example of how data can be leveraged to improve disease management with limited physician interaction, and given there is less volatility in glucose levels for Type 2 patients there is less risk associated with using an AI-driven device. I believe Dexcom also recently received approval for a continuous glucose monitoring device that does not require calibration, and the data collected by these companies in the next several years would be massive.
The point Narmeen makes above regarding provider intervention is definitely valid, but I wonder if in diabetes there is a chance to massively minimize the role of the physician or replace it entirely when it comes to management of glucose levels (although of course imagine a provider would still be involved in diagnosis and general patient monitoring. There is a company called Intarcia (link to the website below) that is on file with the FDA for an implantable osmotic pump that can regulate glucose levels and only needs to be implanted/checked once per year – I wonder if down the road ML data collected from Abbot and Dexcom’s CGM devices can be leveraged for a fully autonomous treatment using the osmotic pump technology. Thanks for providing so much food for thought!
https://www.intarcia.com/
Great content! I found the topic you chose to be very interesting not only because Diabetes is a dangerous diseases, but also because it severely impacts the patient’s lifestyle. However, It is not clear to me how the ML models use data to improve. It seems to me like they are simply using data to gain knowledge about diabetic people’s habits as opposed to using it to predict actual patterns. For example, I would be more intrigued by their model if it predicted what the next sugar level of the patient would be and advise him on the Insulin intakes accordingly.
Also, i think your third question is spot on as this is a concern I had when reading your article. A lot of the data that these companies need is historical medical data which is usually confidential. How can they get access to it and when do we know if we are invading patients’ privacy? Will patients still feel safe using Abbott’s products knowing that their data is being used. What if this data was shared with insurance companies?
Thanks for writing on the issue, disease detection, management, and treatment are very important. I feel similarly to Petra that I’m not entirely clear on how machine learning versus advanced data analytics plays into the future of diabetes. This is not to say that there is not a place for ML, but there are almost so many ways that this could go. I think many of the examples listed have opportunities to be turned into questions that would benefit from ML, however, I feel as though I may be missing the link between these two states.
Thank you very much for sharing this important topic. You mentioned that 409.4mm glucose measurements, 86.4mm monitoring hours and 63.8mm scans by 50,000 people have been collected to date. This volume of data poses challenges for providers and patients and I’m particularly interested in the training and education that Abbott hopes to provide so that patients from all backgrounds can access and utilize this technology to the fullest extent.
Thank you for an interesting essay! Per your first question, you dive in to the issue of ML still being a “high touch” way of diagnosing diabetes due to the need to train the algorithm. While this is definitely true, this issue should become less relevant going forward as a function of the algorithms getting better – as this should reduce the number of “inconclusive” diagnosis, where doctors need to step in and make a call. Hopefully this should be enough to make it almost self-sufficient in terms of labor, but there might be other issues I am missing.
Thanks for the interesting read! I think that this countering human bias is one of the biggest challenges with regards to machine learning. I would argue that the reason that these biases occur is because machine learning algorithms do not discriminate between correlated relationships and causal relationships. We should strive to collect enough data on our processes to reveal true causal relationships which provide more accurate predictions than using correlated data. If we can improve data collection in this way, we can counter those biases.
I can see how machine learning can sift through data and learn from it to predict better solutions. It seems to me that Abbott at the moment is using a data analytics tool which then is read by scientists to determine the next steps. Building the cause and effect into the system will help build a library for it to predict solutions on its own in the future. One of the more critical solutions Machine learning in this instance provides, is early detection or even providing early warning signals that you are on the path to diabetes.
The third question you raise is very important as well and I am sure will be subject to scrutiny by the regulatory authorities. Health data is extremely private, with several individuals reluctant to even discuss this with their doctors. Submitting it to one pharmaceutical company could be viewed as dangerous by the authorities. Insurance companies may misuse this data as well, and therefore finding the right balance here is key.
Thanks for highlighting the effect machine learning can have in this field. I see the benefit of identifying new consequences or potential causes of diabetes; however, machine learning does not identify causal relationships, just correlated ones, so one could not simply state that, for example, rice consumption has led to a high incidence of diabetes. Instead, one could say that the two are correlated with one another, and this could then guide further research into the matter. So I see machine learning as a research tool to analyse complicated sets of data, not a replacement for identifying causation.
While I appreciate that the advent of automated glucose measurement devices enables the collection of useful data related to the management of diabetes, it was not exactly clear to me how machine learning will help diagnose new incidents of diabetes. Typically, I would assume that only people with diabetes or a familial history of diabetes would measure their glucose levels regularly. Therefore, I don’t see how a typical person might be identified as a high-risk candidate of having diabetes, unless he/she measures glucose levels regularly.
Thank you for sharing. This is a space that is ripe for innovation. One area in which I struggle, as some of the other reviewers have pointed out, is whether machine learning is truly a breakthrough, or if it is just one more step in a linear development model. How do we know when there is breakthrough technology that truly changes the game? I would love to know what other markets in which this will be applicable, and whether work is being done to make it affordable for low-income patients.