Beauty Brand with Droves of Data: How Glossier Employs Machine Learning
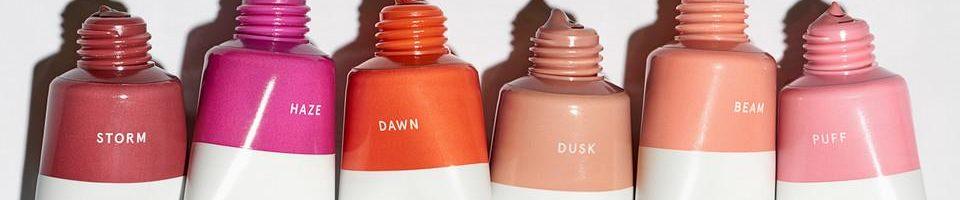
Glossier engages its customers on numerous platforms, each providing this beauty brand with a wealth of data. Glossier is feeding this data into machine learning algorithms to better direct the customer down a purchasing path and to automate the crowdsourcing of product development.
Glossier, launched in 2014, is a beauty brand born from a blog that now deems itself a technology company [1]. Its redefining of beauty standards and authoring of makeup trends have propelled Glossier to millennial-cult-brand status. However, Glossier’s inclination to maximize learnings from customer data might be what really gives this beauty titan its competitive edge.
Beauty Brand with Droves of Data
Glossier engages customers on numerous data-rich platforms. Customers visit the brand’s originating blog (Into the Gloss), browse Glossier’s website, interact with Glossier’s social media, and visit physical stores [2]. Customer-brand interactions provide data that informs predictions of when behaviors will turn profitable. Glossier’s blog and social media provide platforms for customers to voice opinions on Glossier products and desires for new ones. This offers opportunity for Glossier to predict what products or features will perform well in the future, a process currently done manually but which machine learning could automate [3].
Glossier’s massive amounts of data from varied sources result in data that is both “big” and “wide.” As Glossier grows, its data becomes bigger and wider. Glossier must distinguish “out-of-sample” from “out-of-context” data before mistakenly applying a model informed by a subset of customer interactions (e.g. online) to a different category of interactions (e.g. in-person). [4] Further, Glossier must use caution when automating the digestion of customer-brand engagement if these interactions are core to its identity.
Short Term
Glossier recognized the need to connect disparate data upon wrongly assuming blog readers would become online customers [3]. Though the brand’s launch spurred blog traffic (see Figure 1), existing readers did not equate to revenues on Glossier.com. To study customer behavior fluidly between online platforms, Glossier hired marketing tech firm Segment [5]. Using cross-domain machine learning, Glossier tracked identities across the channels to identify profitable patterns. Glossier discovered that blog readers read mobile articles, followed links to shop on Glossier.com, then completed transactions via desktop. Now, Glossier recognizes this pattern and proactively provides customers with desktop and mobile links between the blog and website. [3] Glossier also sources data from email, social media, and physical stores. Segment helps store this data and feeds it to machine learning algorithms [5].
Glossier relies on customer engagement to inform product development, once using a blog post to crowdsource its facial cleanser development [6]. Glossier manually digests “comments left on articles, social media, user-generated content posts and product pages,” but plans automate this process with machine learning [3].
Figure 1
Source: Data excerpted from We Analyzed 9 Of The Biggest Direct-to-Consumer Success Stories To Figure Out The Secrets to Their Growth — Here’s What We Learned (CBInsights, 2017).
Further Ahead
CEO Emily Weiss is planning Glossier’s “Phase Two,” during which the brand will create a “social-selling” website providing customers with a “social media and shopping mashup” [2]. A social commerce development will provide richer data from which to learn customer behavior patterns and desires. With these plans and 37% of its employees deemed “technologists”, Glossier dubs itself a technology company [1].
Recommendations
A social-selling app could close gaps between data sources, just as Glossier did across online channels. Glossier could incorporate member profiles into blog browsing, online shopping, and in-person shopping with member check-ins or location recognition. With cross-channel tracking of customer behavior, machine learning could identify profitable patterns and tailor customer experiences accordingly. For example, a common purchase path might involve a customer viewing a product online before purchasing in person when geographically feasible. Glossier could identify the start of this pattern online and prompt purchases by inviting customers to visit nearby stores.
Glossier’s data sources are growing at a faster rate than what can be developed to learn from them. Figure 2 plots traffic on Glossier.com since its 2014 launch. Machine learning only accurately answers isolated questions such that “the data you feed to your learning algorithm includes [all] there is to the problem” [7]. For example, Glossier identified customer behavior patterns online that generated purchases. New physical stores might significantly alter the purchase journey. Until data from in-person interactions is incorporated into Glossier’s learning algorithms as recommended above, the algorithm that learned solely from online behavior will capture a limited picture.
Utilizing Glossier’s wealth of data warrants caution. Data-rich organizations often derive findings beyond the scope of KPIs. Using machine learning, Glossier must cut through massive amounts of data to discern which performance indicators are key [8].
Figure 2
Source: Data excerpted from We Analyzed 9 Of The Biggest Direct-to-Consumer Success Stories To Figure Out The Secrets to Their Growth — Here’s What We Learned (CBInsights, 2017).
Open Questions
Looking ahead at Glossier’s employment of machine learning, questions remain:
Although a social-selling app provides promise, will it be feasible for Glossier to analyze customer behavior across all channels seamlessly, or will disparate data need to be learned from as such, limiting machine learnings to the extent of customer behavior in individual channels?
Glossier plans to use machine learning to analyze customer engagement to inform product development, a process currently done when the brand interacts with customers’ comments and crowdsourced ideas online. Will this automation hinder customers’ personal connections with Glossier and tarnish Glossier’s brand?
(798 words)
- Pamela Danziger, “5 Reasons That Glossier Is So Successful,” Forbes, November 7, 2018, https://www.forbes.com/sites/pamdanziger/2018/11/07/5-keys-to-beauty-brand-glossiers-success/#698cf85f417d, accessed November 2018.
- Kim Bhasin and Janine Wolf, “Inside Glossier’s Plans to Shake Up Your Makeup Routine,” Bloomberg, August 30, 2018, https://www.bloomberg.com/news/features/2018-08-30/millennial-makeup-brand-glossier-shakeup-makeup-routine, accessed November 2018.
- Hilary Milnes, “How Glossier Uses Data to Make Content and Commerce Work,” Digiday, June 13, 2017, https://digiday.com/marketing/glossier-uses-data-make-content-commerce-work/, accessed November 2018.
- What every manager should know about machine learning. Harvard Business Review Digital Articles (July 7, 2015).
- Lauren Johnson, “These Marketers Are Crunching Mounds of Data and Using AI to Understand the Customer Experience,” Adweek, November 9, 2017, https://www.adweek.com/digital/these-marketers-are-crunching-mounds-of-data-and-using-ai-to-understand-the-customer-experience/, accessed November 2018.
- Bridget March, “Glossier’s Emily Weiss on Democratising Beauty,” Harper’s Bazaar, October 9, 2017, https://www.harpersbazaar.com/uk/beauty/a44230/glossier-emily-weiss-interview/, accessed November 2018.
- How to tell if machine learning can solve your business problem. Harvard Business Review Digital Articles (November 15, 2016).
- Ahmed, T. Jilani, W. Haider, M. Abbasi, S. Nand, and S. Kamran. Establishing Standard Rlues for Choosing Best KPIs for an E-Commerce Business based on Google Analytics and Machine Learning Technique. International Journal of Advanced Computer Science and Applications(November 5, 2017).
I really enjoyed reading your Glossier post from a machine learning standpoint as I had studied it from a crowdsourcing viewpoint. While machine learning would provide increased efficiencies as the company scales, I think there is definite risk in overdoing it and losing their connection with the consumer (its strongest asset). The solution in my mine is to limit machine learning and roll it out slowly (i.e., channel specific and only within the more automatable aspects of data analytics) rather than aim to completely automate its business model.
Very cool perspective! Thinking about it from a different perspective, where you want to unleash the full power machine learning, I think having checks and balances in your system to ensure customer satisfaction and engagement levels are still high would work to ensure that your connection with customers is not lost. I think Glossier, whose leaders consider themselves a technology company, would be more amenable to this, given eventually they will want to converge towards machine learning for making sense of the massive amounts of customer data.
The bigger question for me relates to Jade’s initial question, is how to parse out the data and to understand which data is credible and should be acted upon? I.e. looking at customer surveys vs actual customer purchasing behavior. How do you assess credibility of these data sets and prioritize the data segments when making decisions? Do you still need human decision making skills for this or can machine learning solve this as well?
Agree with the response above–losing connections with customers could be a risk as these types of companies increase their use of machine learning and big data analytics. That being said, there are opportunities to leverage machine learning even further with unstructured data sources such as social media to further enhance customer understanding.
Great read! Although I see your point about potentially losing connection with customers, I wonder if Glossier could mitigate this risk by having more of an interactive online presence. By having “customizable” colors, for example, Glossier can both get users involved on a deeper level and also gather valuable data that could benefit the evolution of their products.
Love your post about this extremely relevant, millennial-driven brand. You bring up a valid concern about the risks of automation, but I think so long as the automation relates to the aggregation of data (comments left on articles, social media, user-generated content posts and product pages) and does not impact interaction with the customer, Glossier should be able to maintain their personal touch, while improving efficiency. Moving forward, they should make sure that machine learning is only used to inform product decisions, but does not serve as a replacement for their consumer-brand dialogue.
Super interesting piece linking both machine learning and the “inbound concept” we learned in marketing. I share your concerns about the massive amounts of data challenging Glossier’s ability to “mine” the data effectively and derive important insights. It seems plausible that they could inaccurately glean trends from the data without understanding the full picture given the significant size and scope. Interested to see what happens with the social-selling app!
I enjoyed reading this piece. It really highlights the question of how much information we as consumers are willing to share with brands, and what value we will get in return for sharing that information. Would a consumer be willing to let Glossier track their brand interactions across channels if they felt that it created a better overall shopping and/or lifestyle experience? I would think yes, but how many brands would they be willing to provide this access to before it starts to feel intrusive? I think Glossier is definitely helping its chances at being one of the few brands that will get this type of holistic access to its consumers if it continues to build out its brand ecosystem and focus on customer loyalty.
This was so interesting to read – I am a huge fan of glossier both as a consumer and as a co-founder of a digitally native skincare brand (aavrani – check out aavrani.com). As you mention, the driving force behind the brand’s success has been its sustained high level of customer engagement. This was achievable because Into the Gloss had established credibility with Glossier’s target consumer. I think the question you pose about whether or not the use of machine learning in product development will jeopardise this trust is an excellent one. I also wonder whether the brand’s meteoric rise from indie to mega brand will also alienate consumers.
Jade, thank you for this eloquent piece on Glossier — I learned quite a bit. I wonder if your proposal for Glossier’s social-selling app actually addresses your concern about Glossier maintaining personal connections with its customers. Glossier could be strategic about its customer interaction by identifying and engaging select high-value customers via the app, as measured by data relating to members who could be/are brand ambassadors, product predictors, frequent buyers, etc., while still having the benefits of machine learning to digest comments en masse.
Really enjoyed reading this! I think that if Glossier plans to aggressively pursue an omnichannel strategy, they don’t really have a choice other than to analyze trends across channels. As you pointed out, the customer purchase journey often crosses channels, including some non-purchase engagement channels, so KPIs will also need to cross channels. Certainly, they can track some single channel trends, as well, but a holistic approach may have the additional benefit of driving a more unified omnichannel experience. With regards to customer interactions, I think it’s possible to do both. If the algorithms are correctly making predictions, customers should still feel heard. However, marketing teams will need to give this process a “human” face, which may require some old-school interactions, as well.
Glossier is my favourite brand, thanks for writing this! It has been interesting reading about them from a machine learning perspective. As the most digitally native and social savvy brand, I think there is definitely potential in harnessing their troves of data for product development and customer service. I think you raise a valid concern in this transition toward machine learning perhaps disrupting individual connection with the brand. One way to mitigate this could be to have an “upvote” system similar to Reddit where users could vote on each others’ ideas which would further build a connected community. Another reference is online skincare brand Paula’s Choice which has built a connected affiliate website Beautypedia as a wiki for skincare with user-generated content and reviews, which reinforce the prestige and credibility of the Paula’s Choice brand.
Ms. Enns, I thoroughly enjoyed your article! And I am not-so-ashamed to admit that I occaisionally am known to enjoy a pleasantly refreshing Glossier face mask from time to time…but I digress. To your question regarding being able to utilize data on an integrated basis across channels, I believe this is thoroughly possible thanks to the rise of e-commerce shopping habits among consumers, and the use of credit cards. Glossier is in a unique position where it can track consumers’ shopping across various channels by potentially linking credit card numbers, and this would allow them to understand why and when consumers make certain purchases. Do certain promotions work better on certain channels? Do certain platforms (smartphone, website) work better for different products? These sorts of questions can be addressed and processed with machine learning and widescale data collection.