SUALAB: Deep Learning For Flexible Operation In Traditional Manufacturing
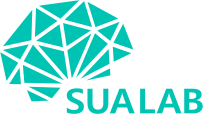
Shifts in the consumer demand are pushing the traditional manufacturers to be more efficient than any other times before. SUALAB is using machine learning on vision inspection to help those manufacturers fulfill the new demand and maintaining control over costs.
The era of small, frequent, various orders has arrived in the traditional manufacturing industries such as electronics. [1] Lots of US manufactures migrated to Taiwan, China, and Vietnam, therefore, are relocating their facilities to their home country, to deal with faster turnover and rising wages in the developing countries, resulting in the increase in the overall production cost.
Efficiency is the most critical issue for these manufacturers to maintain their profit and to secure their customers. Little increase in the defected products, or the delivery delay can now cause worse loss than before. For instance, the production recipe – including the raw material and required equipment – changes every time when the new order puts in the place, resulting in the increase in the production complexity.
The concept of using machine learning to efficiency has been around from 1950s. [2] Manufacturers have been using machine learning to inspect the work-in-process and finished goods. The error patterns, such as a scratch on the PCB board, were manually recorded by human. However, as the product types become more versatile, the error rate of these conventional inspection machines increased a lot, making the manufacturers spend more on hiring additional labors to re-inspect the machine-inspected products. Over a 10-year period from 2005 to 2015, 78% of firms had improved their ability to fill this demand but had lost control over costs. [3]
SUALAB: next generation of machine learning inspection
SUALAB, a start-up in Korea founded in 2013, helps the manufacturing industry by offering an analytics solution, SuaKIT, that can replace the human eye in the industrial field based on machine learning. SuaKIT receives a bunch of normal and defective product images from the client, categorizes the given images and creates a deep learning model based on the features it extracted from the classified images. [4] As it gathers more data, the precision of the solution gets higher. It also classifies the types of the errors.
[Exhibit 1. SuaKIT core functions]
It is helping industries that could not apply the conventional machine inspection due to the complexity of the product, such as Solar cell, and the industries with higher error rates, such as PCB manufacturers to improve the efficiency with the machine learning.
According to the VP of business development at SUALAB, the biggest challenge is to obtain enough amount of the “errors”, a natural limitation since the defects are always less than normal products. The company is trying to solve this issue in a completely different way – It is now working on a system which learns from abundant “normal” data rather than defects. Under this new scheme, with the same amount of data, the error-detection rate gets around 50% higher than before. [5]
In the longer term, the company is to provide the clients with the root causes of the errors in the longer term, a step further from the error categorization system which act as a black box that the user never knows what’s inside before dissembling. While breaking this “black box” requires a large amount of technical investment, this is critical in improving the accuracy of whole solution.
Recommendation
One thing I would recommend to SUALAB is to engage the industry leaders, such as Samsung, to use the solution, as proven in the case of Aspiring minds. A lot of potential customers hesitate to adapt the breakthrough technologies. In case of SuaKIT, the problem becomes worse, since the current inspection system be changed, and the failing in inspection directly results in the loss. To convince such top-tier clients, starting from their suppliers (e.g. cover glass supplier of Samsung) would be a practical approach.
The other recommendation is to specialize the internal organization. The key to successful market expansion is to identify each customer’s unique needs and customize SuaKIT to the specific environment. The current system lets sales and R&D team serve everything; the sales team feeds R&D team the client needs and R&D works on the product. As the company grows, it would be difficult to continuously offer a high-quality service if one team serves every client. So, I would diversify those team based on the industry, while maintain current product development process, so that the dedicated sales and researchers can promptly communicate to devise a better service meeting the client demand.
In relation to these recommendations, my open questions to the company are as following:
- Among industry and geography, which aspect is considered more importantly when expanding the client?
- Data security / leakage seems to be a significant risk. To which extent do you share the data when developing the product for different clients?
- As the organization grew from 2013, how has the organization structure changed? How does SUALAB recruit and retain the top-tier engineers?
(783 words)
[1] John Hagel at el., “The future of manufacturing – making things in a changing world”, Deloitte University Press (May 2015), https://www.deloitte.com/insights/, accessed November 2018.
[2] Brynjolfsson, E. and McAfee, A. (2017) ‘WHAT’S DRIVING THE MACHINE LEARNING EXPLOSION? Three factors make this AI’s moment’, Harvard Business Review Digital Articles, pp. 12–13.
[3] Raghav Narsalay, Aaroshi Sen and David Light (2018), “How Manufacturers Can Get Faster, More Flexible, and Cheaper,” Harvard Business Review Digital Articles, pp. 2-5.
[4] SUALAB, “Product,” http://www.sualab.com/, accessed November 2018.
[5] Author’s interview with VP of Business Development, November 8, 2018.
This is a pretty exciting company given its potential for improving rework and production quality! The data sharing/security risk question you pose is a thought-provoking one, and an additional tension I see about sharing information is that as they give their client companies more information about what is going wrong, companies may eventually improve their processes to an error rate of zero. Consequently, it seems that essentially as Sualab helps companies improve, they also decrease their own client pool and business.
In addition, you mentioned the blackbox problem that they face where they don’t actually know what the algorithm is “thinking.” I think this article posed an interesting way to interpret the blackbox problem–essentially breaking down the machine learning model into more interpretable decision trees: https://hamsabastani.github.io/interp.pdf