Anticipatory shipping—retail’s crystal ball?
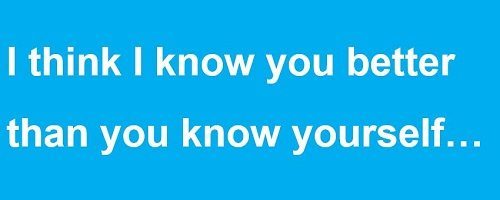
Can products be on their way to you even before you think of ordering them? Can a company accurately anticipate your order and ship before you even place it? Amazon and other online retailers are investing in machine learning to more accurately forecast consumer demand and reduce fulfillment and shipping costs
You’ve run out of toilet paper. You go online to order a 12 pack of your favorite kind—Charmin’s ultra-soft 3-ply rolls. You hover over the “Add to cart” button, but then you remember that you have 2 rolls stored away in another cabinet. Little do you know that Amazon has already begun shipping Charmin ultra-soft toilet paper to its hub in Stoughton, Massachusetts about 23 miles from your apartment in Cambridge[1]. When you go back online in 4 days to order your toilet paper, it arrives the same day. Boom! As the customer, you’re impressed that Amazon has beat its 2-day prime delivery promise, while Amazon has simultaneously managed to reduce its fulfillment and shipping costs through machine learning[2].
Amazon and a number of other online retailers are using machine learning to predict consumer demand, in hopes of more accurately forecasting demand and, in the case of Amazon, reducing costs[3]. As Amazon continues to challenge brick-and-mortar retailers by cutting prices for consumers, it faces strong pressures to simultaneously reduce its cost-to-serve and maintain its margins. For instance, the company’s logistics costs have skyrocketed in recent years. In 2017, the company’s fulfillment and shipping expenses amounted to $25.2 billion and $21.7 billion respectively[4]. In total, that represents 26.4% of Amazon’s net retail sales and has been growing since 2009 when the figure stood at just above 15%. Rising fulfillment and shipping costs have become such a critical risk factor for Amazon that in its 10-K annual filing, it notes:
“We rely on a limited number of shipping companies to deliver inventory to us and completed orders to our customers. If we are not able to negotiate acceptable terms with these companies or they experience performance problems or other difficulties, it could negatively impact our operating results and customer experience.”[5]
It has become evident that this is a major challenge that must be dealt with rapidly. Tackling the rise in fulfillment and shipping costs of this magnitude will enable prices to continue to be held as low as they have been for consumers.
In the short-term, Amazon is investing in what it calls “anticipatory shipping” to reduce both its fulfillment and shipping costs. Data from a customer’s previous purchases; items added to carts, but not yet purchased; items in the wishlist, and even data from a customer’s cursor movements are used in machine learning to predict what the customer will likely order[6]. This allows Amazon to use standard shipping, which is typically a third of the cost of 2-day expedited shipping, to send such items to its hub closest to the customer. In this way, once the customer actually places his/her order for the items, the items are much closer and less expensive to deliver (i.e., ground vs. air freight delivery).
Looking beyond the next two years, Amazon must address the biggest weakness in its value proposition in comparison to traditional brick-and-motor, which continues to be delivery time; the former typically takes 2 days to ship, while the latter works almost instantaneously. In order to be more convenient and as quick as its brick-and-mortar counterparts, Amazon must be able to deliver most products within hours and, eventually, minutes from the time an order is placed. To do so, it will need to augment its anticipatory shipping machine learning algorithm with its own delivery fleet that is able to transport products for short 25-50 mile distances, allowing last-mile deliveries to be made from its hubs to customers within minutes. Amazon has already taken initiative to bridge its speed gap relative to its brick-and-motor counterparts by testing drones and smart door locks inside customer homes[7]. But these technologies have to be paired with predictive analytic tools and anticipatory shipping to reduce delivery times to minutes[8]. In this way, the Charmin ultra-soft toilet paper that would have typically taken 2-days to be delivered, can be at your door within 60 minutes because it can be dispatched from Amazon’s hub in Stoughton, Massachusetts.
What does machine learning and anticipatory shipping ultimately mean for the customer? At least in theory, it should mean they’ll receive items faster, but will it also mean reduced shipping costs for Amazon’s customers?
As for Amazon, will it have to go a step beyond simply delivering to a hub closest to its customer, and actually ship to its customer the products that it anticipates he/she will want before a purchase is made? Will it have to risk occasionally leaving customers with free products they didn’t pay for? How far does Amazon need to go to truly eliminate the need for its brick-and-mortar counterparts?
[797 words]
[1] Alvalara TrustFile. (2018). Amazon Fulfillment Center Locations | Avalara TrustFile. [online] Available at: https://www.avalara.com/trustfile/en/resources/amazon-warehouse-locations.html [Accessed 14 Nov. 2018].
[2] Boone, T. and Ganeshan, R. (2013). Exploratory analysis of free shipping policies of online retailers. International Journal of Production Economics, 143(2), pp.627-632.
[3] Ulanoff, L. (2018). Amazon Knows What You Want Before You Buy It. [online] Mashable. Available at: https://mashable.com/2014/01/21/amazon-anticipatory-shipping-patent/#Ryy4twKmRiqb [Accessed 14 Nov. 2018].
[4] Bernard, Z. (2018). Amazon is spending more and more on shipping out your orders. [online] Business Insider. Available at: https://www.businessinsider.com/amazons-logistics-costs-are-growing-really-fast-charts-2018-2 [Accessed 14 Nov. 2018].
[5] Services.corporate-ir.net. (2018). SEC FILING | Amazon.com Inc Form 10-K. [online] Available at: http://services.corporate-ir.net/SEC.Enhanced/SecCapsule.aspx?c=97664&fid=15414896 [Accessed 14 Nov. 2018].
[6] Nichols, M. (2018). Amazon Wants to Use Predictive Analytics to Offer Anticipatory Shipping. [online] SmartData Collective. Available at: https://www.smartdatacollective.com/amazon-wants-predictive-analytics-offer-anticipatory-shipping/ [Accessed 14 Nov. 2018].
[7] Yu, D., Cheong, T. and Sun, D. (2017). Impact of supply chain power and drop-shipping on a manufacturer’s optimal distribution channel strategy. European Journal of Operational Research, 259(2), pp.554-563.
[8] Bishop, T. (2018). Jeff Bezos explains Amazon’s artificial intelligence and machine learning strategy. [online] GeekWire. Available at: https://www.geekwire.com/2017/jeff-bezos-explains-amazons-artificial-intelligence-machine-learning-strategy/ [Accessed 14 Nov. 2018].
Great read! how can this be established to small businesses? In the future, are there any privacy concerns that we are not aware of?
Thank you Jonathan!
Very interesting read, did not know about the anticipatory distribution, but it makes sense. However, I’d be curious to know how they handle all those that share accounts. For example if I have a sibling in LA who hovers over toilet paper, but the main account is mine and I’m in New York, do they anticipate delivery to NYC or LA? I wonder if this will lead Amazon to offer a family account option that allows individuals to login as themselves, but with a shared cost structure.
Thank you for reading! Yes, I too am curious about how they’ll tackle multiple users who have different addresses, but use the same Amazon account. Which address will they ship to–perhaps they can use the IP address of the person who looked up the product?
This is a very interesting application of machine learning. In retail, with the multitude of applications of data-driven technology, companies need to carefully consider how each will impact the business. For instance, if machine learning is being used to forecast inventory, the benefit is seen in cost reduction, but is not consumer-facing. If machine learning is used to refine individual customer product recommendations, for example, the customer gains from a better experience, and the company gains from increased conversion.
In this case, anticipatory shipping may significantly reduce shipping costs for Amazon, but perhaps more importantly, will improve the customer experience through convenience and “delight.[1]” Everyone loves to receive packages, and the delight factor typically increases if the customer is not anticipating its receipt. It will be interesting to monitor the customer response to this initiative, if it is properly executed.
[1] “Why Amazon’s Anticipatory Shipping Is Pure Genius.” Forbes, Forbes Magazine, 28 Jan. 2014, http://www.forbes.com/sites/onmarketing/2014/01/28/why-amazons-anticipatory-shipping-is-pure-genius/#78f75a2a4605.
This is very cool and almost has a minority report feel to it — predicting our behaviors ahead of time. Exciting, scary. It also relates a bit to some of the supply chain challenges we’ve seen throughout the course, including this week with the beer game. It’s counterintuitive on one hand to think of Amazon guessing when and what you will order, potentially wasting time and money shipping something you ultimately don’t want (and may need to return or will just keep and demand no charge). But in reality, after having studied some TOM, we see that holding inventory has its own (often less immediately apparent) cost. Further, there is a qualitative aspect to being able to service customers more quickly (and Amazon already does this to some degree with subscription services and one-click purchases). Finally, in a real way, this is merely an extension of existing inventory management logic; as the beer game showed, forecasting is inherently an educated guessing game so this new Amazon move isn’t as risky (or, if you like, even as revolutionary/novel) as it seems at first read.