🍕 Pizza & 🤖 Robots: How Zume will change future meal consumption and the food supply chain
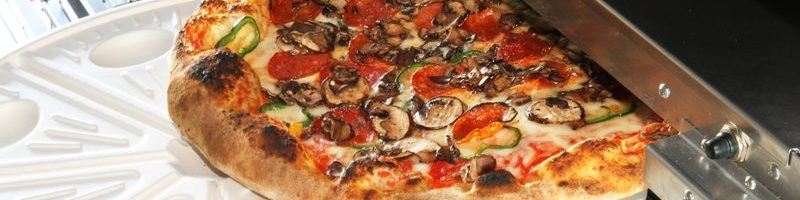
A robot throws on your last mushroom and pepperoni toppings before whisking your pizza into an oven in a delivery truck — after some driving, the oven senses when your pizza is done, and cuts your pizza into perfect slices… right as it rolls up to your front door. Let’s dive into how machine learning is evolving food tech to unlock cheaper meals, faster deliveries, and personalization at scale.
Imagine a world in which you finally step out of back-to-back meetings at 1PM — your stomach is loudly grumbling — and immediately as your mind locks in on “shrimp tacos”…  a delivery rolls up with freshly-made shrimp tacos awaiting your arrival. In fact, the truck had anticipated your order last week, and had already loaded up shrimp, salsa, and tortilla ingredients earlier in the day.
Today’s world is not there yet, but the growing importance of machine learning will play an important role in food consumption, with delivery robots and trucks anticipating when, where, and what you want to eat.
In Mountain View, Zume Pizza uses robots, humans, and machine learning to get you fresher, delicious pizza more quickly. Humans and robots work together to shape pizza dough and add toppings before they are put into a delivery truck, with pizzas cooked en-route to finish baking right before arriving at its destination. [1]
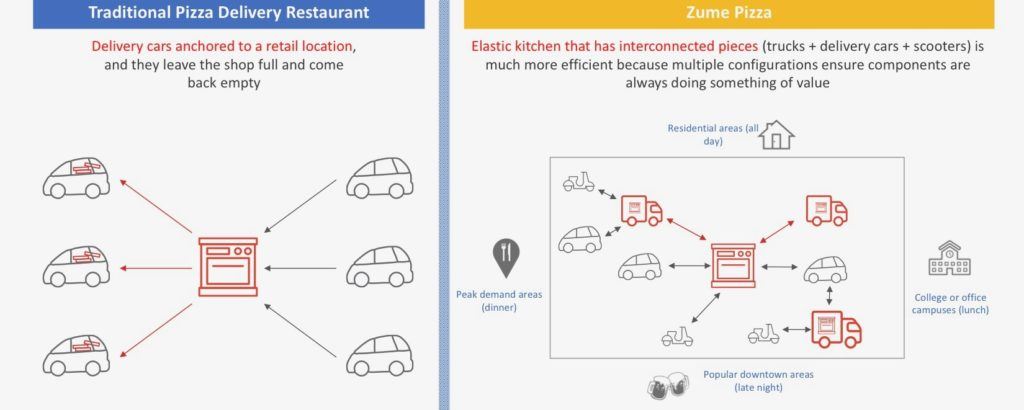
[Video Source: TechCrunch]
Delivering what you want to eat…before you know you’re even hungry
Re-routing in real-time ensures that your hot pizza stays hot:
Zume’s Baked On The Way™ Food Delivery Vehicles identifies the optimal delivery route while its pizzas bake en-route to the consumer [2],[3]. The machine algorithm responds to changes by re-routing or turning on-and-off the 56 ovens in each truck — this capability to flexibly integrate signals (e.g., traffic, historical data, future ordering patterns, weather, delivery truck GPS) is fundamental in automation of lightning-fast speed. Many delivery companies today rely on similar intelligence; for example, UberEats optimizes by calculating meal arrival based on predicted ETAs, historical data, and various real-time signals at the restaurant, and leverages spatio-temporal forecasting models to predict shortages in driver availability to incentivize drivers to log in during specific times. [4]
Predicting demand unlocks personalization at scale:
More data points on time, place, and type of consumption translates to smarter demand planning for hungry consumers. Unlocking the ability to predict creates opportunity to (a) pre-make food that you will want to purchase, and (b) position trucks in the right area to further minimize delivery time. For Zume, people tend to order pizza on the weekday around the same time and have it delivered to the same location; its algorithms further predicts density of orders in an area using weather, “zeitgeisty events like a Game of Thrones premiere”, and more. [5] Based on those factors, delivery trucks are loaded with pizzas that the algorithm thinks customers will want, and send them in that area in anticipation of future demand.
Forward deploying food inventory reduces supply chain waste:
Zume’s intelligence has additional effects on reducing ingredient inventory costs — the ability to better predict consumer eating patterns helps to stock the right number of ingredients, resulting in fresher food with lower cost and less spoilage risk. With this improved predictability, fresher food can become more accessible and less expensive over time.
Robots evolving to make more complex meals:
Expanding assortment is a gateway for many food possibilities, with CEO Alex Garden floating “coffee, steamed buns and frozen yogurt as potential ideas” — pizza was just the prototype [6]. In the future, robots can become smarter to take on more complex actions at a quicker pace. Zume has partnered with Welbilt to build version 2.0 for Zume’s trucks, with hyper-efficient, custom-built appliances to service a broader assortment of food. [3] It is critical for “the  integrated robots [to have] the ability to operate in dynamic, unpredictable environment” to better anticipate growing needs. [7]
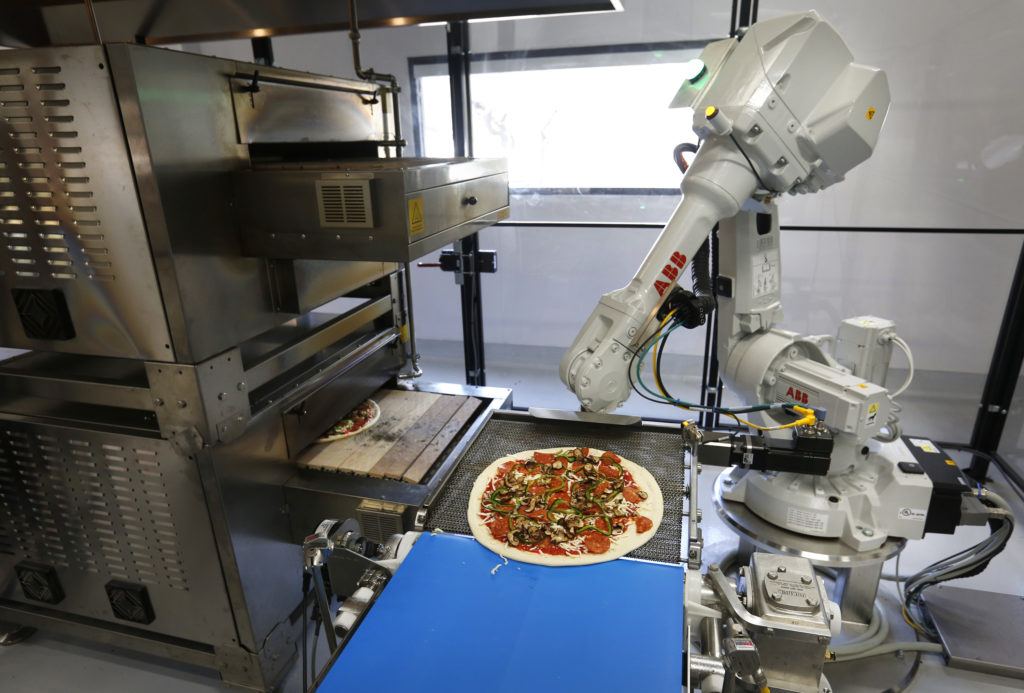
What’s next to help hungry consumers?
Zume has many challenging topics to consider, ranging from internal hiring to food safety to product investments. Some key steps to consider are:
- Capital investments: Due to capital intensiveness of the business, Zume should further evaluate how they want to efficiently allocate spend — there is a long-term tradeoff between investing in expanding density and geographic scope of current trucks versus investing in expansive technologies beyond the existing pizza prototype. [8],[9]
- Organizational design: As Zume continues to hire, leaders should evaluate whether current reporting lines paint a clear picture on internal priorities (e.g., data science report to CEO signals fundamental importance of analytics whereas having food safety report to CEO stresses a different priority).
- Integrating consumer preference data: In expanding outside pizza, Zume should dig into how to best understanding consumer intent without wide range of behavioral data and eating patterns.
As we think about evolving food technology, there are several key open questions we anticipate Zume and similar businesses to face: What are the biggest risks — is it in the technical build (e.g., building the predictive model or dataset itself), organizational design, or food and safety? Do you believe that machine learning may displace human activities in food production, and distribution?
(Word Count: 798)
Sources
[1] Entis, Laura; Baran, Jonathan. “This Robot-Made Pizza Is Baked in the Van on the Way to Your Door”. Fortune, September 29, 2016. http://fortune.com/2016/09/29/this-robot-made-pizza-is-baked-in-the-van-on-the-way-to-your-front-door/, accessed November 11, 2018.
[2] “Zume Pizza Unveils the Doughbot to Perfect the Art of Pizza Crust Creation“, Press Release. June 28, 2017. https://www.webwire.com/ViewPressRel.asp?aId=210316
[3] “Zume Pizza Expands Bake On The Way™, Forms Zume Inc & Partners with Welbilt to Go Beyond Pizza, Introducing Next Generation Food Delivery Vehicles”, Press Release. April 25, 2018. https://www.webwire.com/ViewPressRel.asp?aId=223197
[4] Hermann, Jeremy; Del Balso, Mike. Uber Engineering. “Scaling Machine Learning at Uber with Michelangelo”. November 2, 2018. https://eng.uber.com/scaling-michelangelo/
[5] Stolzoff, Simone. “These Two Silicon Valley Pizza Places Show The Challenges Posed By Automation And Inequality“. FastCompany, February 2, 2018. https://www.fastcompany.com/40511861/these-two-silicon-valley-pizza-places-show-the-challenges-posed-by-automation-and-inequality, accessed November 10, 2018
[6] Heater, Brian. “Zume looks to life beyond pizza”, TechCrunch. April 25, 2018. https://techcrunch.com/2018/04/25/zume-looks-to-life-beyond-pizza/ , accessed November 10, 2018
[7] Frost & Sullivan.  “Technology Growth Opportunity Series–Collaborative Robots.” September 29, 2017
https://cds-frost-com.prd2.ezproxy-prod.hbs.edu/p/71319/#!/ppt/c?id=D77F-00-1D-00-00
[8] The Economist. “Technology firms may struggle to disrupt the food business”. November 26, 2016. https://www.economist.com/business/2016/11/26/technology-firms-may-struggle-to-disrupt-the-food-business
[9] Zaleski, Olivia. “Inside Silicon Valley’s Robot Pizzeria”, Bloomberg. June 24, 2016. https://www.bloomberg.com/news/articles/2016-06-24/inside-silicon-valley-s-robot-pizzeria, accessed November 9, 2018
[10] Mannes, John. “Zume Pizza Adds Extra Automation as it Rolls Out to New Cities.” Techcrunch, June 28 2017. https://techcrunch.com/2017/06/28/zume-pizza-adds-extra-automation-as-it-rolls-out-to-new-cities/, accessed November 11, 2018
Im a bit skeptical that a robot will ever be able to predict what I want to eat and when I want to eat it. Half of the time I myself am not even sure what I want, so I question whether an algorithm will crack that code. Im also curious as to how Zume will deal with its operating expenses. Having trucks with expensive machinery driving around the roads feels expensive. Traffic also increases the liability and potential for accidents, Id be curious to see how the company deals with this going forward.
This is genius and scary at the same time. I think it can work pretty well with frequent customers who has a “predictable taste”, i.e. they eat mushroom pizza all the time, but I do not see it working with people who has random ideas, not a usual pattern of eating out / ordering, just something more spontaneous. I also see a risk in losing customers who decide to change their diets (what is more and more common), e.g. they can have a commitment of eating healthy from tomorrow or losing wait, etc., and then a pizza shows up at their door at day 1 of the new life as the biggest temptation based on their historical buying pattern, what they would like to change. This can be a real pain and can turn away loyal customers.
This is a fascinating application of machine learning in the food industry that has potentially applications for the delivery industry more broadly. If companies are able to better predict customer demand before they place an order, they could start the order fulfillment and shipping process when demand is low. This would allow the company to prepare orders evenly throughout the duration of the day, potentially reducing staffing needs. There’s also a possibility that anticipating consumer demand could allow companies to dispatch delivery vehicles during non-peak hours, thereby reducing the number of delivery vehicles on the road during peak rush hour. This would not only reduce delivery costs for the company, but would also reduce overall congestion in a city (which is often in large part caused by delivery vehicles). If companies are able to lower the costs associated with delivery, they can then lower the retail prices of the goods, thereby making it easier for people who live outside cities to obtain the items they need even if they do not have access to a car.
Hi Nancy, this is fascinating!! What an innovative application of machine learning to reduce operating costs AND improve service (an elusive double win). My thoughts on this is that while there are significant benefits to having operations aligned and ready to meet potential demand, espeically predicted spikes in demand, does this also make them more susceptible to huge and costly operational errors (e.g., if they project a lot of demand for a certain event and ramp up operations for that, and this demand doesn’t materialize?) Or on the flipside, if they are projecting low demand on a specific day and didn’t account for a certain unforseen spike, could they be caught even more off-guard than if they hadn’t made that projection?
Application of machine learning in food services can indeed decrease costs and reduce wastes. However, one thing to consider is that while it is possible to forecast demands at the aggregate level, forecasting demands at the individual food truck /restaurant level may be difficult and inaccurate. Individually, humans are unpredictable, and it is hard to tell with great precision/accuracy what each individual might want to eat on a particular day and what toppings he/she might want to try. This reminds me of the law of coin flipping — individual food trucks can have wildly inaccurate demand forecasts (equivalent of getting 20% heads or 30% heads), but at the aggregate level all of food services will have pretty accurate forecast (precisely 50% heads). The challenge is that if individual food truck/restaurant can’t forecast accurately and see the benefits of such technology, adoption will be slow and the impact of this technology will be very limited. Other questions to consider is the dependency on delivery. Can this business model succeed without delivery? Would people want to visit a restaurant without staff and human service? And how does this limit the Total Addressable Market of this technology?
Zume sounds quite bold and exciting. However, I cannot help but think that the asset-intensiveness of this model is a major obstacle to the scalability of their business. In addition, I am interested in understanding what is the required degree of accuracy on the demand predictability to realize the impact on inventory management and, consequently, meal prices describe by the author – especially if they enter the territory of more complex foods. The flipside of this is increased food waste and inefficient operating expense management.
Personally, I would recommend senior management to stick to simpler food types in the medium-term until POC and economic feasibility is achieved.