PayPal’s Use of Machine Learning to Enhance Fraud Detection (and more)
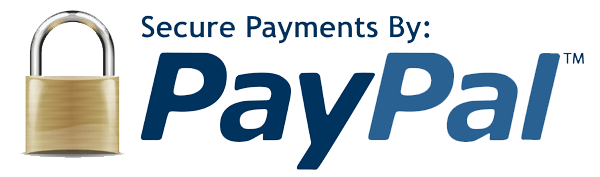
This essay will begin by focusing on how and why PayPal is leveraging machine learning in fraud detection today. It will then consider additional potential applications of machine learning across the customer journey, and how these applications may serve to reduce costs and increase customer engagement.
This essay will begin by focusing on how and why PayPal is leveraging machine learning in fraud detection today. It will then consider additional potential applications of machine learning across the customer journey, and how these applications may serve to reduce costs and increase customer engagement.
As of today, PayPal primarily leverages machine learning to enhance its risk management and fraud detection capabilities. Online transaction fraud is a huge and growing concern that is “expected to reach $25bn by 2020, which means that $4 in every $1,000 will be fraudulent.”[1] From a profitability standpoint, being able to detect fraudulent transactions is imperative for PayPal since payment processing is a low margin business where revenues are split among multiple players: issuing banks, networks, merchant bank, payment gateways, and processors (See Exhibit 1). [2] From a customer engagement and retention standpoint, PayPal must ensure that good customers are able to complete their transactions (no false positives). Going forward, frictionless consumer payment experiences will be key for PayPal to defend its incumbent position in an industry with growing competition (e.g. Apple Pay, Amazon Pay, Visa Checkout, etc.).
Why is machine learning being used to enhance fraud detection? Traditionally, financial institutions automatically flagged transactions as “high risk” based on a set of clearly defined rules (See Exhibit 2), and then either denied or manually reviewed flagged transactions. However, this traditional approach to fraud detection is falling short given the:
- Increased availability of data and humans’ inability to leverage it all (vs. increased effectiveness of machine learning when faced with more data). [3]
- Increased complexity and types of data (See Exhibit 3), and inability to capture relationships with a discrete set of rules.
- Human errors and bias. [4]
- Increased sophistication of fraudsters (many of whom are actually leveraging machine learning themselves).
- High incidence of false positives, which reduce sales (and revenues) and increase manual reviews and thus operating costs. [5] [6]
To maintain its leading-edge position in risk management, PayPal acquired the fraud detection start-up Simility in June 2018. Simility uses the following approach to build fraud detection models: [7]
- Use unsupervised models to find clusters and identify anomalies
- Manually review and label them
- Train a supervised model using the labels
It is also worth highlighting that Simility continues to believe that human intelligence plays a role in fraud detection. Their basic premise is that human intelligence is being used to create new fraud techniques every day, and models that have been trained on past data may either miss those transactions or take too long to incorporate the pattern into their model. [8]
As I reflect on the future of online fraud and consider an e-commerce ecosystem in which the use of machine learning and payment method tokenization is widespread, I wonder whether we will ever reach the point of almost inexistent fraud or whether fraudsters will continuously be able to find loopholes.
Beyond fraud detection, PayPal is assessing opportunities to leverage Natural Language Processing to increase the efficiency of their customer service function in the coming years. For example, by introducing well-functioning chatbots and restricting human interaction to instances when it adds unique value, PayPal could significantly reduce SG&A costs without harming the customer experience. Autonomous research quantified this cost saving potential in their 2015 report, “the finance sector can leverage AI technology to cut 22% of operating costs.”[9]
Additionally, I believe that PayPal could further leverage machine learning in the medium term to improve its product offering to customers. The payment experience itself is becoming increasingly commoditized, and some competitors such as Amazon (with their Amazon Go “no checkout” grocery stores) and merchants such as Uber are even going as far as omitting it from the buyers’ experience. In response to this trend, PayPal is seeking ways to increase its revenue streams and maintain brand relevance beyond the checkout button. To this end, PayPal has offered additional funding sources (e.g. PayPal Credit) and financial management capabilities (e.g. partnership with Acorns investment startup) to consumers, as well as customized loan terms (e.g. PayPal Working Capital) and end-to-end services (e.g. marketing tools, shipping) to merchants. I believe that machine learning gives PayPal the opportunity to further personalize these products for their customers. For instance, PayPal could leverage available data on a consumer’s spending patterns and social presence on Venmo to: i) offer suggestions on how much he/she should save, ii) tailor investment opportunities to his/her wealth and risk profile, iii) tailor a merchant’s product placement to his/her preferences.[10] Additionally, the more consumers engage with these products, the better the model will become, and the more relevant the recommendations. While these machine learning applications would clearly bring value to end consumers, I wonder whether PayPal could avoid trespassing the thin line of infringing on users’ privacy.
(799 words)
Exhibit 1: Online payments processing players and fees
Source: Online Payments Processing: How Pricing Really Works. PayPal
Exhibit 2: Illustration of Rules-Based Fraud Detection
Source: Amazon Web Services. “Fraud Detection with Amazon Machine Learning on AWS.” 22 Sept. 2017. Reading.
Exhibit 3: Simility’s use of structured and unstructured data to reduce fraud
Source: “Transform and Enrich Your Data.” Simility.
Endnotes
[1] Anderton, Kevin. “The Future of Fraud.” Forbes, 27 May 2016.
[2] Online Payments Processing: How Pricing Really Works. PayPal
[3] Brynjolfsson, Erik, and Andrew McAfee. “What’s Driving The Machine Learning Explosion.” Harvard Business Review Digital Articles.
[4] Amazon Web Services. “Fraud Detection with Amazon Machine Learning on AWS.” 22 Sept. 2017. Reading.
[5] “Cutting down on payment-related Fraud via Machine Learning and AI.” China TravelNews, 15 Aug. 2018.
[6] “What Are Your Fraud Rules Costing You?” Blog Against Fraud, Kount, 27 Aug. 2014.
[7] Sandepudi, Ravi. A Primer on Machine Learning Models for Fraud Detection. Simility, 28 June 2017
[8] Sandepudi, Ravi. A Primer on Machine Learning Models for Fraud Detection. Simility, 28 June 2017
[9] Kruse, Jacob, et al. Machine Intelligence & Augmented Finance. Autonomous, Apr. 2018
[10] Wojcik, Natalia. “Pefin, a fintech start-up, is using A.I. to offer financial advice. Just don’t call it a ‘robo advisor..'” CNBC, 9 Sept. 2017.
Casilda – this is a very well-reasoned and thoroughly researched paper. Well done on understanding the nuances of a very rich and complex payment ecosystem, where (as you point out) many collaborators (e.g., merchant acquirers, issuing banks, card networks, unbundled gateways, etc.) all compete for a sliver of the typically meager 2-3% transaction fees. You’re absolutely right in that addressing fraud through machine learning is the near-term key to success for PayPal in handling online fraud. Rules-based engines just become too unwieldy and are not as flexible as machine learning algorithms. PayPal’s acquisition of Simility also points out a unique approach that many corporations take in first taking on small minority investments in high-potential start-ups (often with potential strategic benefits) to have a seat at the table when these companies grow and are ripe for acquisition or future rounds of funding. (In Dec 2017, PayPal had participated in Simility’s $17.5M round: https://venturebeat.com/2017/12/12/fraud-detection-startup-simility-raises-17-5-million-from-accel-paypal-others/). I also agree with your and Simility’s assessment that human judgment will (and should) continue to play a role in fraud management near-term. A well-structured fraud prevention stack should consist of multiple tools to help fend off various forms of fraud. Well done!
I wonder if PayPal could use Simility to not only identify fraudulent payments, but also criminal networks? I would guess that most fraudulent transactions aren’t merely one-offs, but part of a larger network of unsavory activity. By identifying the payers and recipients of fraudulent payments, could we learn how criminal enterprises launder money through the financial system?
Good research paper Casilda. I really think that fraud is one of the biggest topics for Fintechs today. I agree with Kate, Paypal can use this technology to go beyond fraud detection. One way a digital bank in the Middle East leveraged this technology is to detect accounts are prone to be part of a criminal network / money laundry scheme. This would enhance Paypal’s reputation and increase customer trust in the platform.
Thank you Casilda !
This is amazing work Casilda. Thank you for sharing. I’m wondering, based on your projected rates of fraud ($4 for every $1000), whether having imbalanced data affects the overall challenge of developing algorithms that can detect these problems.
This is a great writeup! Fraud is definitely a major issue, and innovation here directly saves hassle for customers and money for PayPal. This article is timely for me, because PayPal actually thought one of my accounts was fraudulent. I guess I am naturally a sketchy guy.
The question I have is whether the fraudsters themselves will continue to get smarter here and even use machine learning. At what point will we have computer vs. computer battling it out for the best fraud? Or, at a certain point, will machines be smart enough to not do evil?
Great write-up, Casilda!
I wanted to ask – is there still a significant human element in all of this? Meaning, is the labeling portion heavily dependent on humans? I am hoping that maybe, eventually, we can move to a world where machine learning is detecting new types of fraud that humans would have never noticed in the first place. Additionally, I worried if we’re relying on humans to detect and label fraud, we’ll always be one step behind the fraudsters themselves.
Interesting article on online fraud. I am left wondering if there are new or innovative ways that PayPal can leverage other technologies in addition to machine learning? Could Face ID or thumbprint identification help to authenticate users when they make a purchase? That data point, when used in conjunction with an algorithm could be even more effective at identifying fraud.