Racial Bias in Healthcare Algorithms
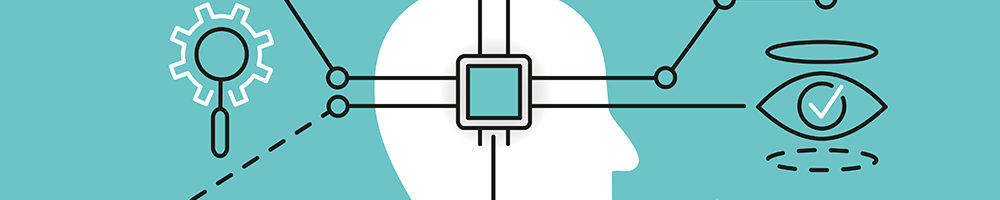
There's notoriously high human error in medicine, but algorithms can be imperfect, too. How should we handle this?
There’s notoriously high human error in medicine. Data driven decision making could help us improve healthcare outcomes, but algorithms based on data can be imperfect, too. What level of algorithmic error is acceptable as in the pursuit of better health outcomes?
Healthcare is a huge area of opportunity for data-driven decision making – in the US, healthcare spending is 20% of our GDP and it’s estimated that 30% ($750 billion!!!) is waste because of all the errors and inefficiencies in care. In the US, we have the highest cost of care of any country in the world, yet some of the worst health outcomes in the developed world.
So can algorithms help us suggest the right course of treatment for a patient and reduce human error? Can they help us most accurately diagnose a patient based on their clinical history? And can they help us drive down costs by comparing clinical outcomes based on different treatments?
With all this in mind, I was struck by this article from the Washington Post, titled “Racial Bias in a medical algorithm favors white patients over sicker black patients.” The article reports on an Optum algorithm that was found to have significant racial bias. The algorithm wasn’t intentionally racially biased (and in fact, had not included race as a category) – instead it used future healthcare spending as a proxy for future disease. But it turns out that white Americans spent about $1,800 more than black Americans on healthcare. As a result, the algorithm consistently recommended more medical care for the white Americans who the algorithm deemed to be “sicker” (when in fact, they were just consuming more of our healthcare resources). This is striking because it shows the dangers of correlating something like healthcare consumption with healthcare need – different populations may consume healthcare differently (for cultural reasons, accessibility of care, cost of care, insurance coverage, etc.) It also shows the risk of algorithms reinforcing bias – in this case, the algorithm recommended more healthcare invention for whites (which the algorithm deemed to be sicker), which only reinforced the existing discrepancy in healthcare consumption.
This is not a new issue. Studies in healthcare show racial bias in the care received – black women in particular are much less likely to receive pain medication, for example, and there’s been other studies that show they’re less likely to receive treatment for lung cancer and cholesterol medications than their white counterparts. But what is scary about an algorithm that’s racially biased is that race can be explicitly excluded from the algorithm – but that doesn’t mean bias was excluded since the measuring stick chosen (consumption of healthcare) differs by race.
I’m currently working on a start-up that cleans and joins data to enable algorithm development. How do you make sure that your algorithms aren’t biased, particularly when they can seem like a “black box” in terms of what’s recommended? And how do we manage the risk of data driven healthcare – presumably these algorithms can be corrected, but an early version might have issues. We are willing to accept human error, but are we willing to accept algorithm error, particularly in healthcare where decisions have life or death consequences.
In this case, researchers were able to correct the bias with a relatively simple solution. They tweaked the algorithm to determine how a sick patient was based on their actual conditions, rather than on their healthcare spending.
The end of the article mentions a future where we may stress test algorithms with data scientists (just as security firms test whether a company’s data security is sufficient).
What do you think? Are the benefits of data driven medicine worth the risk?
This is a great article! One thing I struggle with understanding is whether its possible to satisfy two objectives at the same time. That is – by accounting for racial bias, can you at the same time ensure the overall accuracy of the algorithm and vice versa? If mathematically these two conditions aren’t guaranteed to be satisfied by the same algorithm, how do you think about the tradeoffs we are willing to make?
Great read Sarah! The initial question you have raised has been studied and debated extensively. The fact is as humans we are more forgiving towards human error and NOT AT ALL towards machine errors. Ideally, the combined error rate of all healthcare systems together should be the point at which we start preferring machines. But people still would want a machine to be 100% accurate. Therefore, for the near future, most people would prefer algorithms to be used as a complement to human judgement.
Unfortunately, since algorithms are built by humans with bias, removing that bias is a very hard problem to solve. I do like the idea of stress-testing with data scientists. I also wonder if instead of removing race as a field altogether, we could use it to our advantage. Using such fields to bucket data into different groups can help us analyze each individually. This can identify significance of factors within specific groups, can also maybe help identify some underlying causes that affect certain groups more than others. Ideally, it will also surface the bias when the results are compared across all buckets.
This is a great article, thank you for sharing. I must say, this article also has me terrified, and perhaps indicates our need to have prudent regulatory frameworks in place for sensitive industries such as healthcare, education, security, law & order. The potential for harm if models are missed applied could be catastrophic.
Second, this article also brings to mind a topic that we have discussed a few times in class. The potential for algorithms to reinforce pre-existing biases that are found in the natural world. Therefore before relying solely on the machine, it is always prudent for a human to assess the outputs to basically gut check the data for negative social outcomes.
Stress testing, much like peer review in academica, sounds like a prudent method achieving that. Perhaps this shows then that the application of AI technology for sensitive industries should be done within open-source / collaborative models, instead of closed / proprietary development