Who Me? For that Project? How AI is Changing the Consulting World
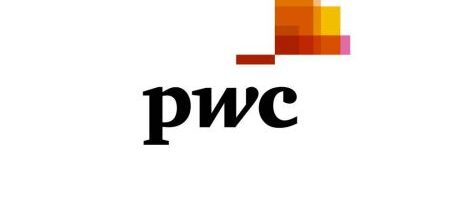
In a company where human-based services drive value creation, Artificial Intelligence (AI) is changing the name of the game.
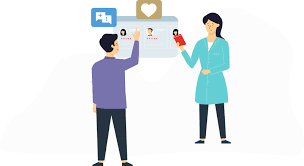
How AI is Changing the Game
In 2018, I submitted a proposal to the PwC Emerging Tech Competition to leverage Artificial Intelligence for assigning staff members to consulting engagements in order to expedite staffing decisions and better align resources to projects.
When I joined the firm, I noticed multiple problems with the way in which staffing was traditionally done. Staffing for the most part was a data-blind process and resources were often misused and underutilized.
Artificial Intelligence (AI) offered a solution because using machine learning allowed the firm to staff team members faster, and also match employees to projects that align with their interests and career goals. The use of machine learning facilitated better matching of individuals with specific skillsets for particular types of engagements.
Value Capture of Using AI to Assign Staff to Engagements
The overall value of this use of AI is that resources are used more effectively, which drives higher revenue because less personnel are idle at any given time. In addition, staff members are more engaged on their projects, contributing to greater work satisfaction and commitment. Not only are staff members happier with their project placement, but the strengths and expertise of staff are better matched with client needs. At the average consulting firm, the turnover rate is 20-25% annually and average tenure is about 2.5-3 years. By increasing staff members’ overall satisfaction with their jobs, companies have the ability to decease churn and drive down costs associated with finding and hiring replacements.
How It Will Work
The idea was to train the staffing model using employee interests, project requirements and TalentLink, which is the program used to manage staff requisition and skills (i.e. it served as a data book with information on all of the employees at the firm).
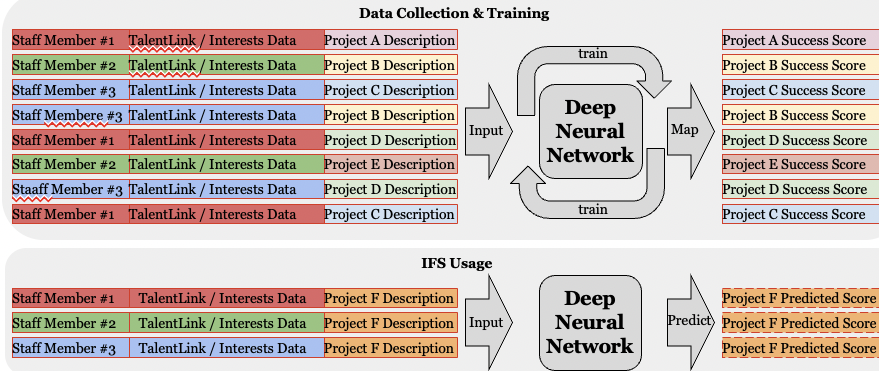
Data to train the staffing model is collected through two steps: (1) from general TalentLink and project information and (2) from post-engagement surveys. Staff will be responsible for updating their TalentLink profiles and Engagement Leaders (i.e. Partners) will be responsible for providing the project information and submitting post-engagement surveys.
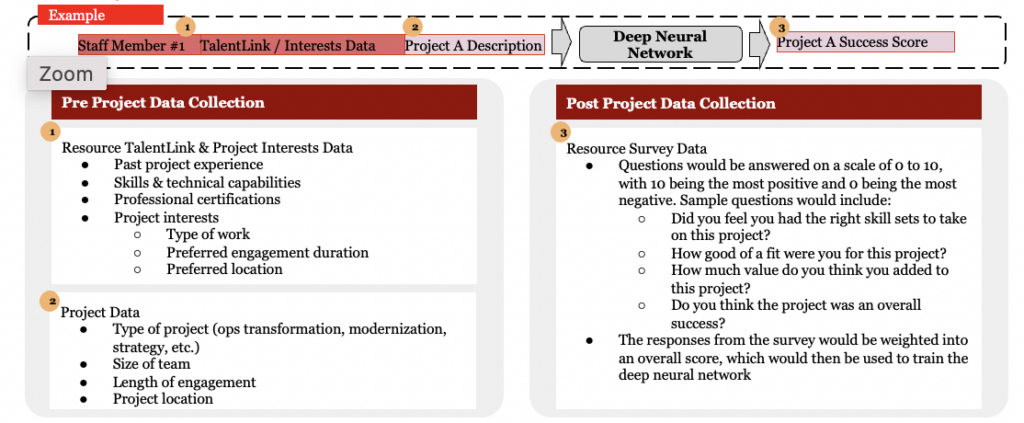
The model matches upcoming projects with available staff members, prioritizing staff experience, skills and interests when making staffing decisions.
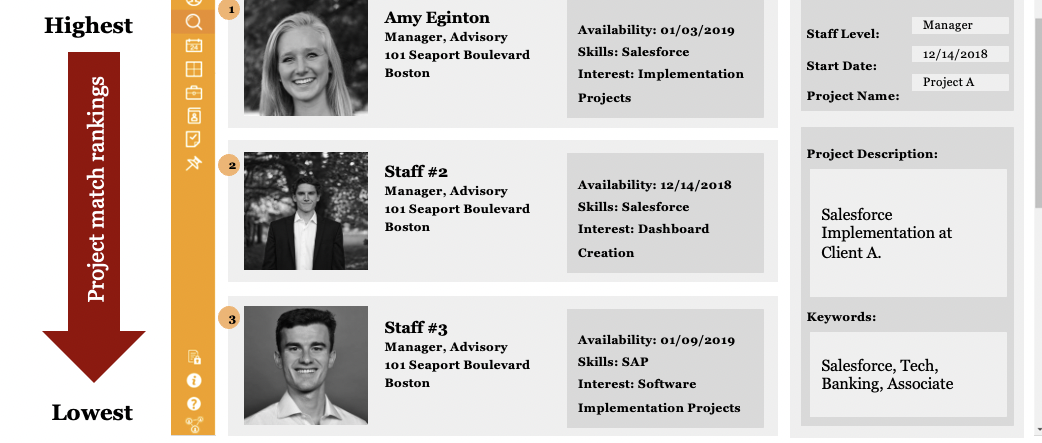
Artificial Intelligence empowers the firm to utilize its resources to their greatest extent and become a more agile organization. Quicker and better staffing means more chargeable time to the client, resulting in higher potential profit.
Challenges of Implementing AI for Staffing
Challenges for implementing AI for this use include limitations with the accuracy of staff members’ TalentLink profiles and incomplete post-engagement surveys. The model hinges on a certain level of engagement from both staff and engagement leaders. If there is a lack of responsiveness form Partners, the model might need to be updated so that Directors or Managers are responsible for providing the necessary information. In addition, the system will need ample data in order to “learn” and iterate on its recommendations. Therefore, significant lead time is required before it can be fully implemented at the firm.
Where Are We Today?
The proposal won the Emerging Tech Competition at PwC and is now under development by the firm’s product engineering team. The idea is not for this AI tool to replace the HR team that is currently managing the staffing assignments, but rather to improve the approach in which engagement staffing is done. There is currently a pilot being running with some members of the Deals Advisory team and the goal is to finalize the AI by Q2 2023 and then roll it out to the full consulting team by the end of Q4 2023.
I liked reading about this, thank you for taking the time to write about it. I have seen a number of HR AI/ML companies pop up recently and it seems they are particularly prone to the “how biased is the algorithm” question, which I have to imagine applies here as well. ML applications in other employee software systems (like electronic health records) also run into the problem you highlighted about having employees buy into the system. Given that the employees have to input their preferences, does it leave room to game the system, or does collective inaction/lack of buy in mean the product suffers enough to never get off the ground? Will employees be ok with a ML generated assignment? To me it presents a bunch of very interesting organizational challenges and questions!
This is so interesting! I wonder what the reaction from partners or project leaders was as I can imagine that staffing can be a process driven also by qualitative elements, such as “I really enjoy working with x”, “I am already familiar with x style of working and works for me”, “I need exposure to y to turn him into a supporter for my promotion”, etc. Could you help me with a bit of color on this?
Super interesting post! As a former consultant, this model resonates strongly with me, as which project you get staffed on has massive impact on how long you stay in consulting for. I wonder how this might impact consultant happiness over time: while I do agree that engagement in a project is a major driver of satisfaction, I also know many of my colleagues would relish their time “on the beach”, particularly when they just got off a tougher / longer engagement. With the increased staffing efficiency of this model, there would naturally be less down time in-between projects — I wonder how that might drive tenure in the long-run?
This is AWESOME and I love that this tool also addresses some bias and gives more agency to the staff. I wish my firm had been able to use a tool like this! I’m curious what the feedback was after this was launched – who was happiest and who was most irritated by the platform when it was proposed?
This is amazing Amy! congrats!
This is so interesting because even thinking about the Corporate world I come from, this could be super useful and would help in eliminating the “I know him/her/them bias. For example, you may have two internal people being considered for a job, but you go with the person you’ve worked with, or even there could be a third person that is not even being considered because they have never worked with the manager looking to fill a position.
Again it depends on keeping the data up to date, wich could be implemented in a more “formal” type of step every time a new project is completed. Almost as must-do feedback.
Hopefully, it works! sounds like the consulting people allocation can be tedious.
This is such an impressive project. Serious props!
I was a consultant at another firm. Like PwC, we solved for similar things in our staffing: skills, interests, availability. But we also staffed intentionally based on gaps. Our belief was that all analysts and associates needed to be well-rounded and have at least 1-2 reps of all major skill groups, even if they intended to specialize in a given topic/function. Counterintuitively, we might staff someone with absolutely no quant experience to a team tasked with building a complex financial model because the experience was important for that person’s development and because the rest of the team could pick up slack as the person learned.
Do you think it would it be possible to include in a more development-focused approach in the model you designed for PwC? I worry that matching just abilities to needs might under-serve junior associates and new-joiners.
This is super interesting! I wonder if this has the bias of self-enforcing what you’re good at vs. what you want to do? If someone hates excel models but is really good at them, how does the algorithm balance agency in project choice / what they find interesting vs. what is necessary for the firm to succeed and what they are good at?
Well done, Amy! This is super cool– when i was reading it, i couldn’t help but wonder whether the value prop for PWC is truly about decreasing employee churn as consulting is an ‘up or out’ industry and thus there could be a natural inclination to keep people somewhat dissatisfied? That being siad, this was a brilliant idea and i hope we get to hear more about it in class