nTopology- Generative Design & The Future of Product Development
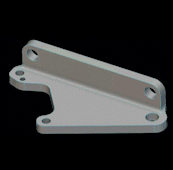
Engineers long saw themselves as immune to AI disintermediation– does generative AI mean we have to rethink that assumption?
Value Proposition:
While product design and development have been improved on since the day of hand-drawn models and analysis, it is still far from an optimized process. There is a vast array of different computer-aided design (CAD) software used to model products. Those all export to different finite element analysis (FEA) systems used to analyze the properties and limits of said products. Modeling, and especially iterating on the results of FEA, takes a lot of person-hours and (from personal experience as a recovering engineer) can be a very frustrating process.
Enter, nTopology. nTopology leverages generative AI to optimize the CAD process. Designers define the boundary conditions and product requirements, enter their initial design, and the system takes iteration into its own hands.
nTopology is a goal-seeking system. The user provides an initial input and boundary conditions, and the system iterates automatically until it reaches an optimized solution. This is the same process a designer would use to manually craft a 3D model, but far faster and more efficient.
Challenges
Technical: nTopology has opted against a standard “black box” model. Instead they have discretized the process into individual, user-controlled “boxes” to allow their clients to have more granular control. Similar to Professor Avi Goldfarb’s point about decoupling prediction and judgement, nTopology leaves the entirety of the latter to the designer. While this is an appealing feature for engineers, who value control over their work, it increases the technical complexity of the product, as now multiple predictive and individually-actuated models are required.
Consumer-Facing: As previously mentioned, engineers deeply value control over their work. While generative AI can optimize the process and make their roles more efficient, there is a psychological resistance towards allowing a program to take the reins. Additionally, convincing users of safety and reliability of the models is a core challenge. FEA lives by the adage “garbage in = garbage out.” Convincing users that the AI is sufficiently able to apply constraints and equations in the way an experienced engineer would is a major hurdle.
Competition: While generative design is still a nascent field, there are some sharks beginning to circle. Namely ANSYS, a well-renowned software company who makes FEA software used across multiple high-reliability industries (healthcare, automotive, aerospace, etc…) recently debuted “Design Discovery.” This allows users to immediately optimize their designs as they perform FEA. This should absolutely be on nTopology’s radar, as ANSYS is a well-established company with deep pockets. Winning bids against them will require both outpricing them and making the design process so seamless that users want to forgo their existing systems.
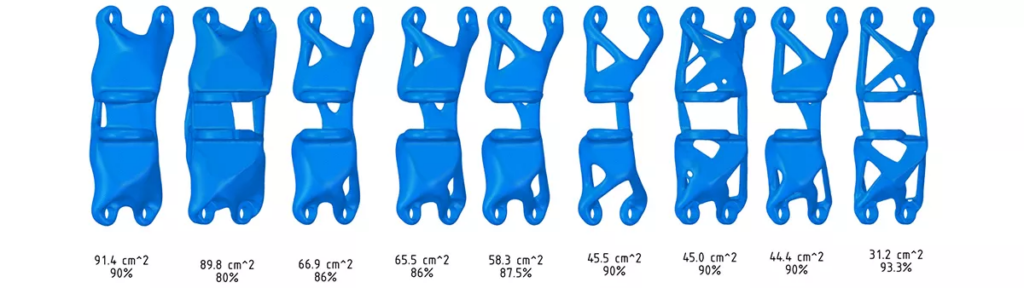
Opportunities
Efficiency: In my experience, the worst part of being a mechanical engineer is the tedium of CAD. Systems are usually complex and hard to use. It’s easy to make errors that take hours to resolve, and having to iterate ad infinitum to earn the extra performance of detail-orientation is often a mind-numbing procedure. The idea of allowing a generative AI to take optimization into its control while still granting the engineer overall design judgement is a highly-appealing product.
Additionally, designing complex geometry often has high-payoff for lightweighting while maintaining strength properties, but physically designing these complex surfaces is incredibly time consuming. An opportunity for nTopology is to focus on promoting the AI as a “last mile” designer. The engineer does the bulk of the work in getting the initial design built, then the AI controls the more-frustrating final tweaks.
Scientific Advancement: All of FEA is built on a few core equations behind the phsyics of materials and structures. While we know a lot about the behaviors of classically-manufactured structures, the advent of new processes (like 3D-printing and laser sintering) has brought new challenges to our understanding of how products behave. Generative AI like nTopology poses an opportunity for scientists and engineers to run studies and optimization exploration far faster than traditional build-iterate-test methodology. nTopology even offers a feature that optimizes the internal print structure of additively-manufactured products, an area previously left as a “black box” to designers.
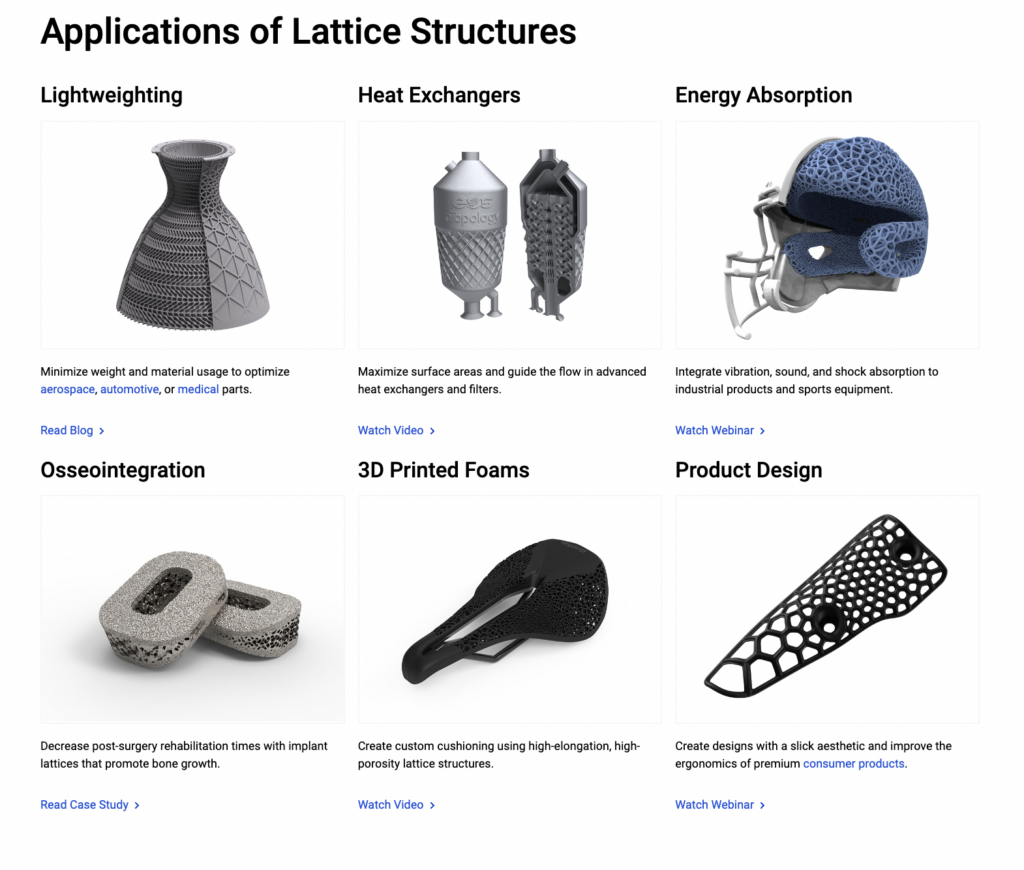
Cost-Savings: A benefit of being able to rapidly iterate on your design and manufacturing plan is that you reduce the need for excess materials and person-hours in designing. This poses a significant cost-savings opportunity for mechanical product firms.
What Should nTopology Do?
Focus on the Product as a Last-Mile Solution: When you’re selling to users you are also potentially disintermediating, positioning yourself as a tool to make their lives easier rather than a disruptor is a much more effective marketing tool.
Run Studies and Write Whitepapers Focused on Reliability and Effectiveness for New Manufacturing Techniques: This is a big jump in design technology and methodology. Establishing effectiveness and trust in the industry makes selling far easier. nTopology should focus on building academic and industry partners who are willing to run design studies and physical tests on generatively-designed products to prove the AI can do what a human can faster without sacrificing quality.
Develop an End-To-End Design Solution: This is something nTopology has already started to do brilliantly. Unlike ANSYS, which is a standalone FEA product that allows you to import CAD from other systems, nTopology integrates directly with CAD software. Putting the whole pipeline in a single suite is a big differentiator for them as a solution.
This is fascinating — thanks for posting, Katelyn! I am not familiar with this type of engineering at all, so it’s really cool to see an AI application geared towards creating physical products (as I typically think of AI in the software realm). I agree that they should focus on developing an end-to-end solution to differentiate themselves, as this reduces friction for the buyer and improves the user experience. I do wonder whether the “last-mile” option puts them squarely in the realm of “incremental solution” rather then “systems change agent” as Professor Goldfarb was talking about today, which could put them at risk of being disrupted if a more radical offering upends the current FEA process in a way that creates value-step change for the stakeholders involved.
I love this! I’m too far removed by now from my mechanical engineering days, but even though I enjoyed playing with Solidworks and the feeling of modernity using simulations versus hand drafting, I can’t help but feeling that generative design is the next step in the evolution and maybe it’s time for some engineers to cede some of the “control” in design criteria. I think the ability of the computer to come up with an optimal design for weigh-reduction/load-bearing trade-off would not only be faster but beyond some typical engineering creativity (just like nature has interesting structures evolved over a very long period of time). In my opinion, this should go beyond the convenience tool for existing engineers and rather democratize product development the way no-code is doing for software.
My hope for nTopology is that they pursue the end-to-end solution, ideally acquire or partner for the right 3D-printing technology (bypassing manufacturability concerns) and making a great UX (I never enjoyed ANSYS learning curve)