Helping Heavy Industry Soar with Falkonry AI
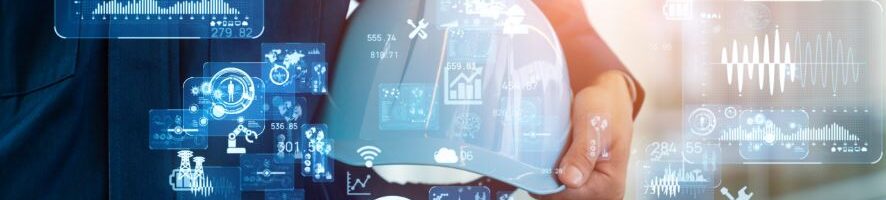
Digital innovation is creating a fourth industrial revolution. Falkonry is providing the AI as a service tools to enable this transformation.
Digitization of Heavy Industry
Modern factories and machinery plants are awash in data produced by increasingly complex arrays of sensors that monitor machinery health, efficiency, and more. The average factory produces 1TB of data a day, but only 1% of this data is analyzed and acted upon in real time1. This limitation results in lost productivity and production yield due to undetected equipment conditions and failures. Unplanned downtime costs industrial manufacturers $50 billion per year2. Tools that are better able to parse this data and allow operators to not only quickly detect failures and aberrant operations but also prevent them are sorely in need. Companies are increasingly turning to AI to analyze vast amounts of operational data in new ways and derive insights to increase their equipment efficiency. This integration of advanced analytics, AI, Internet of Things (IoT), and more to industry mark what is known as Industry 4.0 or the fourth industrial revolution3. Digital and predictive maintenance tools can help companies increase asset availability by 5 to 15% and reduce maintenance costs by 18 to 25%4. However, only 8% of companies are able to achieve their business goals with their investment in digital transformation5. Falkonry aims to be a key player in the fourth industrial revolution by helping companies bridge the gaps in their digital transformation.
How Does Falkonry Create and Capture Value?
Falkonry was created in 2012 by Nikunj Mehta, formerly an engineer at enterprise AI company C3.ai. To create value, Falkonry has developed a suite of time series AI tools (i.e. capable of analyzing data continuously over a period of time6) that can parse data from a variety of sources ranging from naval ship machinery to arc furnaces. In essence, Falkonry allows users to continuously analyze operational data from systems and machinery across an entire machinery or manufacturing plant. Falkonry then leverages both unsupervised and semi-supervised machine learning models to detect anomalies in equipment operation, establish root causes of deviant operation, unearth previously unseen causal relationships, and predict failures before they happen7. Falkonry’s tools are adaptable and designed to produce insights for subject matter experts and operators in real time, without requiring training with historical operating data or teams of data scientists. Falkonry then captures the value they create by packaging these tools into no-code, plug-and-play AI products that they sell to customers ranging from F500 companies to the US Navy. While still a start-up of 50 employees, Falkonry’s suite of tools has helped them grow to an approximate $78M valuation and become a leader in the predictive maintenance (PdM) space8.
Predictive Maintenance in Action: AI and Steel Manufacturing
Steel casting and milling equipment are long-term, high-capital investments for manufacturers. Because of this, steel manufacturers seek to get as much capacity out of their machines as possible to remain cost competitive. Downtime due to either planned maintenance or equipment failure can cost manufacturers millions of dollars per day9. Furthermore, sub-optimal equipment conditions can adversely affect steel quality, reducing production yield. With Falkonry, steelmakers can leverage an easy-to-use AI platform that plugs into their existing suite of instrumentation and monitoring tools, allowing operators to continuously monitor process and equipment data from across the entire plant simultaneously. This allows operators to detect patterns indicating everything from premature equipment wear to installation of a faulty replacement part well before they impact production or result in an unplanned shutdown. Additionally, operators can use the data and plant-level insights from Falkonry’s platform to more efficiently schedule planned maintenance activities10. Combined, this can save manufacturers millions.
AI as a Service: Opportunities and Challenges
As companies increasingly integrate industrial IoT devices with AI and push towards greater digitization, the PdM market is set to grow rapidly over the coming years. The PdM market is projected to grow from $6.3 billion today to $45.5 billion in 2032 at a 21.9% CAGR11. There are tremendous opportunities for companies like Falkonry to create and capture value in this market by providing to industry the AI as a service (AIaaS) tools that will grow this market. Despite this opportunity, there’s several challenges on the horizon for Falkonry. The first is the increasing competition in the market. As expected, there are a number of AI/ML-focused startups entering the PdM space. Additionally, major tech companies like IBM and Microsoft are also making investments in predictive maintenance technologies. While Falkonry is an innovative company, they’re still a relatively small startup and they may find themselves outflanked by bigger and better-funded competitors.
A second challenge is commoditization12. As AI/ML models become more ubiquitous and robust, Falkoner’s models themselves could start to become commoditized and they’ll be forced to compete with large companies on price. However, the data that Falkonry is collecting could allow them to find new niche’s in the PdM market to be competitive in. This highlights a final potential challenge.
As the value of industrial data becomes clearer, customers may gain greater bargaining power over AI/ML providers. Falkonry was able to leverage insights from data collected in the steel industry to develop more sophisticated AI models that predict not only a time to failure but a “time to anything” for equipment ranging from jet engines to generators13. As basic PdM AI models begin to become commoditized, access to large and diverse sets of industrial data will become an increasingly valuable competitive advantage. Large industrial customers may recognize this and begin to demand a premium for access to their data. This could make it harder for smaller AIaaS companies to compete in the space compared to large tech companies or early movers in the space.
References:
- Innovate Manufacturing Processes with cloud and ai. https://www.ibm.com/industries/industrial/resources/business-transformation-interactive/scenes/smart-factory/select/details/production-optimization. (n.d.). Retrieved November 30, 2022, from https://www.ibm.com/industries/industrial/resources/business-transformation-interactive/scenes/smart-factory/select/details/production-optimization/
- Shanker, N. (2021, February 25). Council post: Manufacturing without unplanned downtime could become a reality sooner than you think. Forbes. Retrieved November 30, 2022, from https://www.forbes.com/sites/forbestechcouncil/2021/02/26/manufacturing-without-unplanned-downtime-could-become-a-reality-sooner-than-you-think/?sh=1d18622d70d1
- What is Industry 4.0 and how does it work? IBM. (n.d.). Retrieved November 30, 2022, from https://www.ibm.com/topics/industry-4-0
- Bradbury, S., Carpizo, B., Gentzel, M., Horah, D., & Thibert, J. (2020, December 16). Digitally enabled reliability: Beyond predictive maintenance. McKinsey & Company. Retrieved November 30, 2022, from https://www.mckinsey.com/capabilities/operations/our-insights/digitally-enabled-reliability-beyond-predictive-maintenance
- Padmanabhan, V., Berez, S., & Gautheron, P. (2020, February 4). Four myths of Digital Transformation: What only 8% of companies know. Bain. Retrieved November 30, 2022, from https://www.bain.com/insights/four-myths-of-digital-transformation-what-only-8-percent-of-companies-know/
- Roell, A. (2022, July 21). An introduction to time series Artificial intelligence. AiThority. Retrieved November 30, 2022, from https://aithority.com/guest-authors/an-introduction-to-time-series-artificial-intelligence/
- Parwatay, S., Nikte, S., Ballampalli, R., Lee, C., Mehta, N., Falkonry, & Birla, S. (2022, January 28). Customer experience. Falkonry. Retrieved November 30, 2022, from https://falkonry.com/archives/category/customer-experience/
- Pitchbook
- Parwatay, S. (2022, January 28). AI-based operational excellence in Steel Manufacturing. Falkonry. Retrieved November 30, 2022, from https://falkonry.com/blog/ai-based-operational-excellence-in-steel-manufacturing/
- Ai in metals industry. Falkonry. (2022, September 24). Retrieved November 30, 2022, from https://falkonry.com/metals/
- Fact.MR – predictive maintenance market analysis by by deployment mode (cloud, on-premise predictive maintenance), by component (software, services), by organization size, by Vertical, by region – global forecast 2022-2032. Fact.MR, Market Research Company. (n.d.). Retrieved November 30, 2022, from https://www.factmr.com/report/predictive-maintenance-market
- Editors, F. T. C. (2017, April 10). Council post: The commoditization of AI and the long-term value of data. Forbes. Retrieved November 30, 2022, from https://www.forbes.com/sites/forbestechcouncil/2017/04/10/the-commoditization-of-ai-and-the-long-term-value-of-data/?sh=3568aaa0159c
- Nikte, S. (2022, September 5). Interview with Ian Hersey: Dod’s push for Digitalization and CBM+. Falkonry. Retrieved November 30, 2022, from https://falkonry.com/blog/interview-with-ian-hersey-dods-push-for-digitalization-and-cbm/
Coming from manufacturing and operations and as a person who have seen multiple downtimes due to equipment failures on assembly line, I cannot emphasize more on how important of a problem this is. The question still remains on the data you get from the equipment. Most of the large corporations have legacy systems in place that might not give you the right data that’s needed or sometimes can provide wrong information [ Since a lot of it depends on the sensors that monitor the equipment and assembly], the other issues is the hardware calibration that’s needed on a routine basis. Does Falkonry provide information on those sensor calibration and can take decisions based on the variances measured because otherwise a false positive is equally bad when there’s no issues on hand
The other question that I am trying to wrap my head around is how much of this data can be transferred and applied universally in building algorithms ? A robot which lifts a box of medical supplies from point A to B will have a different life cycle for maintenance compared to a similar robot which picks a much heavier object. How does Falkonry think about this dilemma? Do they create unique models for each customer or do they look at the models they previously have and modify them for this scenario?