Grubhub – Revolutionizing the food-ordering landscape

Grubhub – the leading online and mobile food-ordering company, with 8.2million active diners and 50,000 restaurants in its network across 1,100 cities – is redefining the way people order food. Insights from significant amount of data generated is helping the company refine the food-discovery process for its diners, i.e. right food at the right time at the right price. On the other hand, the restaurants are able to optimize their delivery footprints, pricing and online profiles. Let’s see how Grubhub does all of this.
Grubhub has been connecting diners with local restaurants since its inception in 2004. It is a two-sided platform with diners and restaurants as the two major stakeholders. Few add a third stakeholder – drivers – to complete the puzzle. Currently valued at ~USD 2.9B, GrubHub reported annual revenues of USD 493 million and net income of USD 49.6 million in 2016 with a 36% and 30% y-o-y increase respectively over 2015.[1] The number of active diners rose to 8.17 million and daily grubs to ~275,000, both with 21% increase y-o-y. Big data has contributed significantly to product improvement. This has helped the company add more than one million active diners during 2016, while sales and marketing expenses rose by only 21 percent.
Value Creation
Let us see how Grubhub creates value for its diners and restaurants:
Diners: Discovery
The extensive data collected from tens of thousands of daily order reviews help Grubhub put the right restaurant and the right meal in front of diners at the right time[2]. Just like Amazon, Grubhub’s recommendation engine is based on several factors including past orders and their cuisines, past reviews, popular restaurants (based on other diners’ reviews) in the neighborhood, similar diners’ ordering habits, etc. This helps Grubhub curate and feature on homepage the list of restaurants the diner might like. Figure 1(a), 1(b) and 1(c) show such recommendations from my Grubhub homepage.
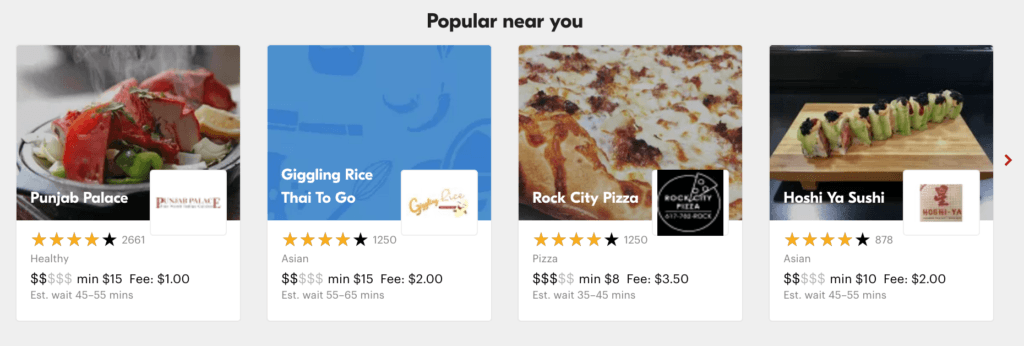
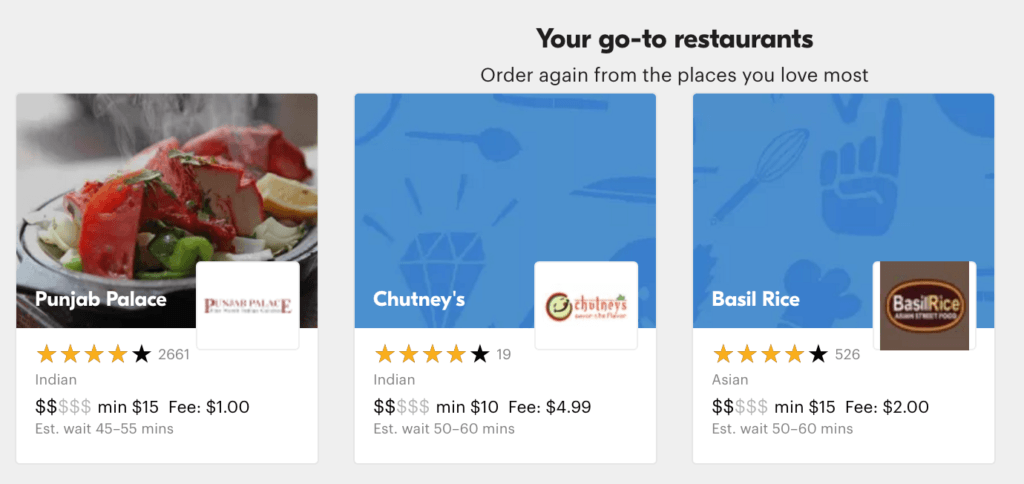
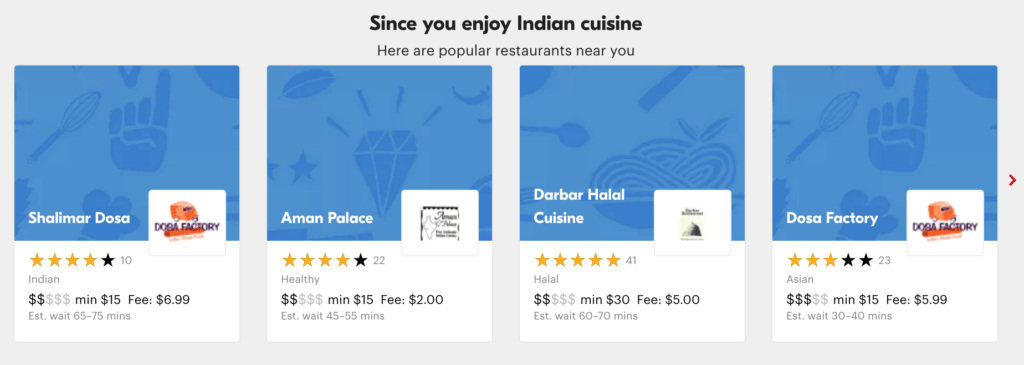
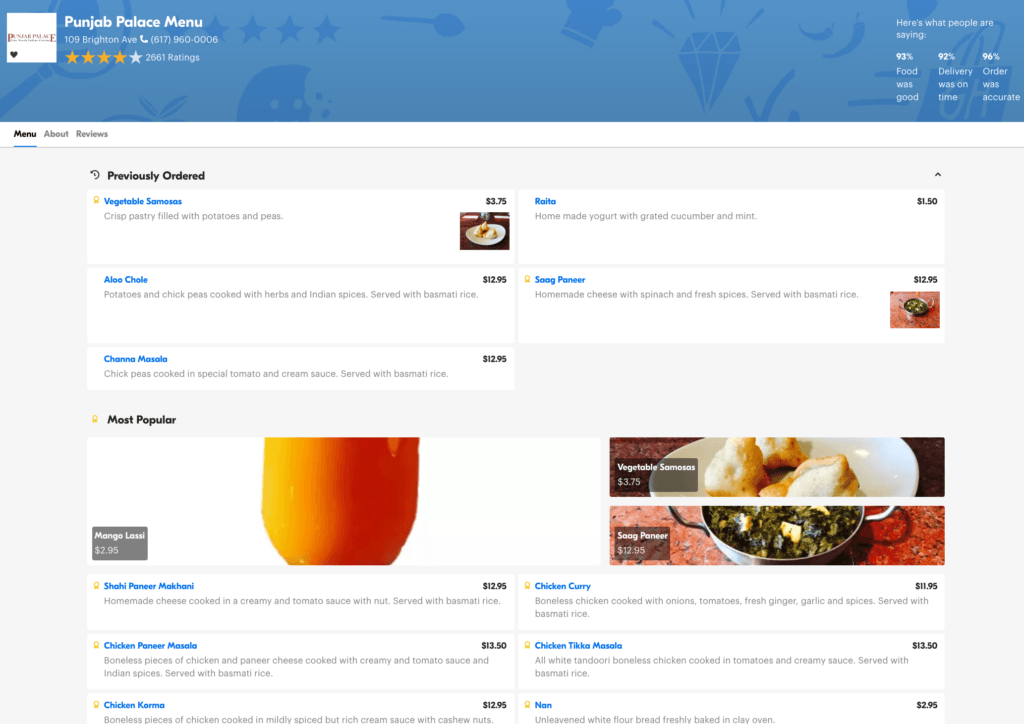
Next, within a specific restaurant page, Grubhub leverages data to show diner the previously ordered and the most popular dishes, as shown in figure 2. This list is curated from thousands and thousands of orders fulfilled by the restaurant on Grubhub’s platform. Another important opportunity here would be to suggest sides/ desserts based on what similar diners have ordered in the past, something along the lines of what Amazon does.
Further, GrubHub recently launched its skill on Amazon Alexa allowing diners to re-order their past orders directly on Alexa[3] and without using their laptop/phone. This is another manifestation of how data is being leveraged.
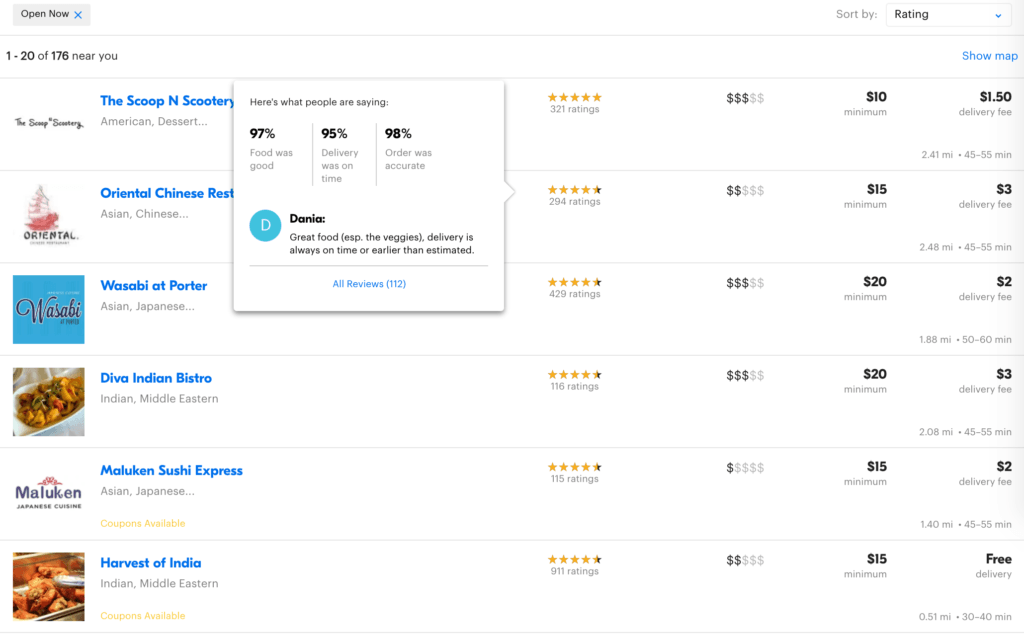
Once the order has been fulfilled, Grubhub nudges the diner to leave feedback on quality of food, correctness of order, timely delivery, etc. The real-time feedback captured from diners feeds into the reviews and ratings which show up as the user is making the decision. One challenge here – GrubHub makes sure to remove the fraud ratings, such as ones fed by the restaurants (at times) on single-item orders (eg. water bottle) to ensure the sanctity of the rating system. What would be further helpful is to capture feedback (data) dish-wise eg. how spicy was the curry, etc. This can further help the company help the diners make better choice about various dishes on the menu.
Restaurants: Order and customer management
Grubhub, in 2016, launched a new product for its restaurants – Grubcentral – designed to provide actionable insights to restaurants based on the orders fulfilled by the restaurant. This is aimed at helping restaurants optimize the inventory, delivery footprint, pricing, etc.[2]
Drivers: Order fulfillment
While Grubhub’s website and 10K do not mention this aspect explicitly, they will need to revamp their delivery and order tracking system. This again can leverage data science. Based on the past order delivery data collected from drivers and time of the day, weather, etc., Grubhub can predict the ETA in real time. They can also accordingly optimize the delivery route for the drivers enabling them to do multiple pick-ups and deliveries on the way, similar to Uber Pool model.
Industry: A Year in Delivery[4]
Being the market leader, GrubHub also has been a pioneer in publishing the major trends in the food ordering industry in United States. This interesting press release by the company mentions how Grubhub analyzes orders by date and identifies the most frequent dishes by popular events like Game of Thrones finale, President election, etc. The study also published geography/ city wise popular dishes and other similar user habits, as shown in figure 4.
Here’s a video on ‘A Year in Delivery’:
While this helped Grubhub predict trends for 2017 and accordingly target customers and onboard new restaurants, it also helps the broader industry to accordingly improve product offerings.
Figure 4: Foor-ordering trends published by Grubhub[4]
Value capture
In terms of value capture, it’s all the indirect network effects game for the company. More restaurants à more diners à easy discovery à more orders à more restaurants… Grubhub’s business model rests on ~15% commission on order value from the restaurants, so it’s able to capture more value by increasing the number of total orders, through all the data science described above.
Challenge
As described above, the major challenge is the robustness of the data. This is overcome by employing right data science and analysis practices and not letting the data get skewed by fraudulent order and reviews. To my belief, the company is doing a great job at presenting the most transparent data and recommendations to its hungry diners and help them order their Grub accordingly.
Sources:
[1] https://investors.grubhub.com/investors/press-releases/press-release-details/2017/Grubhub-Reports-Record-Fourth-Quarter-And-Full-Year-2016-Results/default.aspx
[2] https://s2.q4cdn.com/772508021/files/doc_financials/2016annual/2016-Annual-Report.pdf
[3] https://www.grubhub.com/alexa
[4] https://media.grubhub.com/media/press-releases/press-release-details/2016/Grubhub-Announces-A-Year-In-Delivery-Uncovering-2016s-Top-Delivery-Trends/default.aspx
Great post Mohit. I am curious to know how complex the recommendation algorithm is, I usually find that recommendations are never really personalized, showing go-to/popular/by cuisine does not seem super complex and I’ve never really found a food delivery platform that delivers recommendations based on a diners taste by identifying taste profiles (restaurant cuisine, quality, and flavor profiles). Do you see this happening anytime soon? I know that would make order decisions much faster for me, and would make it less likely for me to switch to other delivery in order to find a place to order from.
Lulu – Well said. Most recommendation engines are not that personalized. Speaking about Grubhub, I won’t say they are quite there yet, but their recommendations for me are definitely better than other services I have tried. A part of that is to do with the number of orders I have made. The more orders you make, the more Grubhub knows about you and the better recommendations it gives you. One interesting thing to note is that the recommendation results shown on the home page are not paid results, as much as I understand. When you search for restaurants on the search page, the ranking does take into account the revenue percentage shared by the restaurant. This transparent results featuring system on homepage makes recommendations really transparent.
Further, as I mentioned in the possible opportunities, once Grubhub starts knowing your taste in detail (eg. you like ‘spicy’ Chana masala, etc.), that’s when the real magic will kick in. Because then the recommendations can really be on the dish level based on what people are saying about that dish. Current ratings are based on high-level quality and correctness of food, and delivery timeliness. Further, Grubhub will also be able to suggest side dishes based on popular combinations.
In a nutshell, agree it is not quite there yet but it will have to reach there soon to maintain its competitive advantage in the crowding industry.