ASOS: Meeting Your Fashion Needs with Data
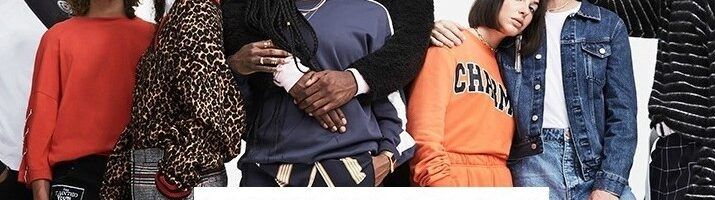
ASOS is a British online fashion and cosmetic retailer. It reported a revenue of 3.3B GBP for the FY 2020 (19% growth over LY)[1]. The online retailer boasts of a highly data driven culture and attributes much of its growth to its ability to draw insights from large product and consumer data.
ASOS has a product catalog of over 85,000 items with 5,000 new products added every week. In the fiercely trend-driven fashion world, the company needs to produce personalized data models as quickly as possible to maximize value delivered to its 23 million active users.
In this blog post, I will talk about the two successful products and features that depend on large data capture and analytics to give ASOS a distinct competitive edge – Recommendation Tool and Visual Search.
While most of the online retailers have been experimenting with building a successful recommendation tool, ASOS has invested considerably in building a separate data science team. This allows the retailer to move beyond the predictive and prescriptive analytics to the latest evolution i.e., cognitive analytics. You can think of cognitive analytics as a matchmaking tool between the company and its customers. Using machine learning in real-time, this tool matches customers with experiences that will promote maximum engagement and drive desired outcomes (leading to higher purchase decisions)[2]. This feature allows ASOS to track individual behavior in real time and allows for quick scalability of recommendations to all of its customers whenever and wherever they engage[3].
The second feature, image recognition, was one of the first unique features offered by ASOS over other online retailers. It allows its customers to scan a clothing and based on its characteristics, the website recommends a listing of like products. As an added benefit, the app also offers recommendations on how to pair the scanned item with other items on the website to improve your fashion quotient. Visual search tools leverage machine vision to identify relevant items on the web and make suggestions.[4]
While the company has successfully built many more such products and features, it is worthwhile to spend some time to reflect on how it has been able to stay ahead of its competition in leveraging data.
Investment in technology: the ASOS teams identified tools (such as Azure DB) that would help them accelerate the modeling process. Using such tools, they analyze gigabytes of product and customer data to produce a recommendation in milliseconds (based on multiple variables such as likelihood of a customer viewing, saving, adding to cart, and completing the purchase).
Change in Organizational Structure: ASOS envisioned a new, digital organization with collaborating teams across functions focused on developing best practices for dealing with data science and modeling. Hence they brought various component teams such as big data team, data science team and API team, together to form fully cross-functional teams aligned to clear business outcomes[5].
Culture of continuous iteration and experimentation: quickly realizing that the machine learning models are rarely successful in their first iterations, these cross-functional teams focused on collecting data to refine product features (such as refining training model). They invested heavily in team’s ability to test and measure quickly using standardizations such as automatic infrastructure provisioning, centralized experimentation tracking tools, real time dashboards etc.
The initiatives mentioned above have helped ASOS to engage with customers and create value for them. Knowing what customers like, how they spend their time on the platform and their individual spending habits, has helped ASOS capture some of that value.
While most of the retailers struggled in 2020 due to global pandemic hurting customer sentiments, ASOS recorded a 19% growth in revenue, an addition of 3.1 million customers and 119 million GBP increase in EBIT. Apart from these financial metrices, key customer engagement and business metrices have also improved consistently for ASOS – units/basket rose from 2.8 in 2016 to 3.2 in 2020. While conversion is up from 2.8% to 3.0% for the same period[6]. This is particularly impressive at the time when other retailers are struggling to get customers to buy more and spending more on marketing efforts.
Going forward, ASOS is well positioned to strengthen its multi-brand platform strategy, test new ideas faster using data, hence scale quicker and pivot more easily as customer trends change.
At the beginning of February 2021, ASOS acquired high-street brand Topshop’s online business in a $363.3 million deal. This acquisition is in line with company’s strategy to further strengthen its data-based strategy by getting access to more data and build synergies. It also has sights on further international expansion, specially North America.
Going forward, it will be crucial for ASOS to successfully integrate its online strategy with social media platforms such as Instagram and TikTok. The future of customer engagements will not be just about recommending relevant products or getting quick conversions, it will be about authentically communicating with customers across their online presence. This is how ASOS can be on top of changing preferences and behaviors of its customers and keep them engaged as the young millennials grow older.
[1] https://www.investorschronicle.co.uk/education/2021/03/10/asos-s-global-growth-potential-still-has-much-to-offer-investors/
[2]https://blog.adobe.com/en/2017/12/12/the-data-story-behind-really-knowing-what-your-customer-wants.html#gs.wdlich
[3] https://customers.microsoft.com/en-gb/story/asos-retailers-azure
[4]https://medium.datadriveninvestor.com/3-retail-leaders-using-big-data-ai-to-drive-efficiency-6358706730f5
[5] https://medium.com/asos-techblog/agile-in-the-age-of-a-i-133762aa0d42
[6] https://www.investorschronicle.co.uk/education/2021/03/10/asos-s-global-growth-potential-still-has-much-to-offer-investors/
Thank you so much for this Surabhi! I really admire the level of dedication ASOS is putting into developing its own data analytics capability. With Cognitive Analytics, I wonder what are some of the specific types of data the company must collect in order to improve its recommendation over time.
Thanks for the great article, Surahbi. I was using Asos the other day and I started to feel some similarities to Threadless. There are just so many options to choose from and everything seemed to be priced similarly. Another interesting tool they have created, in addition to the ones you focused on, is the size recommendation tool. I am not positive, but I am thinking this tool is also powered by data and ML in some way to provide accurate size recommendations. With the tool you answer a series of preference questions like, “how do you like your clothes to fit”. Asos will then recommend which size you should choose for that specific item. For me this added huge value, because I usually avoid buying clothes online in fear they will not fit well. This simple tool gave me the confidence that I was buying something that fit me based on my specific fit preferences. I am imagining this tool also helps Asos build your profile to better recommend products to you.
Thanks for this article, Surahbi. I love how mentioned the change in organization structure to accommodate data culture. I know from my own experiences that many companies tend to think that just because they’ve created a separate data science team, they can sit back and enjoy the fruits of a big data culture. But good data cultures requires a top down approach and good execution throughout the entire organization.