AirDNA: Smarter Short Term Rental Pricing with Human and Artificial Intelligence
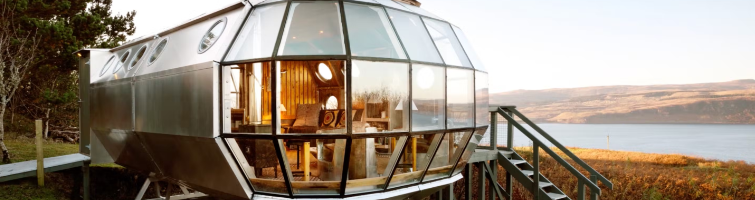
Smarter Short-Term Rental Pricing with Human and Artificial Intelligence
Founded in 2014 by an avid AirBnB host, AirDNA (the “Company”) provides short term rental (“STR”) data and analytics. Through its use of artificial intelligence, AirDNA creates value for STR hosts and investors throughout the short-term rental lifecycle, which includes property selection, listing optimization, dynamic pricing and competitive benchmarking.
The Company tracks daily performance of short-term rental listings to provide market reports and data products that feature occupancy rates, seasonal demand and revenue generated by short-term rentals, enabling vacation rental entrepreneurs and investors to improve property performance and make better data-driven decisions. The Company captures value by charging a monthly subscription price, which varies by the size of the target market:
- For a market with less than 100 active listings, customers are charged $19/month
- Between 100 and 1,000 active listings, customers are charged $39/month
- For a market with more than 1,000 active listings, customers are charged $99/month
AirDNA’s “secret sauce” lies in its three internally developed algorithms: its (1) reservation algorithm, (2) matching algorithm and (3) pricing algorithm.

- Reservation Algorithm: Determining overall demand, occupancy rates and booking rates for short term rentals in inherently difficult due to hosts blocking off specific dates for personal use. AirDNA solves this issue through its Reservation Algorithm, which allows hosts and investors to distinguish between blocked vs. reserved days, thus painting an accurate demand picture for any property in every market worldwide. Along with distinguishing between booked vs. blocked days, AirDNA’s algorithm analyzes the historical occupancy rates and booking lead times of every short-term vacation rental and predicts travel demand up to six months into the future based on real-time future bookings. To create an accurate demand score for properties, the Company created a booking curve based on average occupancy rates and how far in advance those properties were booked in the previous twelve months. This allows the Company to analyze real-time booking fluctuations from this standard booking curve to identify periods of high and low demand. This demand model is created based on the market, rental type, beds, and accommodates filters available in the tool.

2. Matching Algorithm: The amount of overlap between Airbnb and HomeAway varies widely between markets, some having up to 45% overlap on a monthly basis, and others having almost none. The Company’s matching algorithm enables investors and hosts to cut through the noise to account for dual-listed properties across various rental channels, including VRBO, AirBnB and third-party websites. AirDNA’s algorithm routinely achieves a recall score of 95%. Accounting for dual-listed properties is challenging in that properties cannot be identified based on physical address or geo-location (which is intentionally obfuscated). In order to accurately identify cross-platform listings that belong to the same rental property, AirDNA’s Lead Machine Learning Engineer developed an algorithm for identifying properties listed on multiple platforms. The algorithm is an XGBoost Classifier implemented in Python utilizing over fourteen different listing features. Features such as location, listing titles, listing descriptions, numbers of bedrooms, and calendar availability are inputted into a gradient tree-based model that ultimately outputs a probability a given pair is the same underlying property. Taking an algorithmic approach makes identifying overlap across listing platforms scalable and maximizes accuracy. AirDNA analyzes over 10,000,000 listings globally and recalculates matches on a monthly basis to resolve shifts in supply. AirDNA’s Listing Match Algorithm and the infusion of multi-platform data enables customers to see a more accurate representation of what is happening to vacation rental supply on a neighborhood, city, state, and country level.
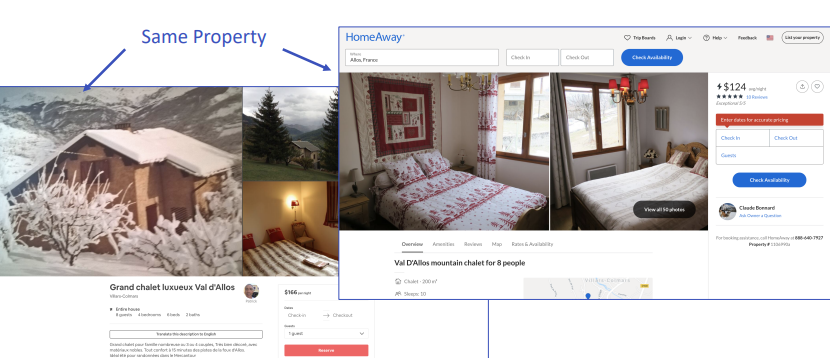
3. Pricing Algorithm: AirDNA’s pricing algorithm, or “Smart Rates”, provides investors and hosts with recommended daily rates for their specific property. The pricing algorithm considers the following pricing inputs: booking demand, seasonality, base rate, market influences, performance data and booking lead time. When calculating booking demand, the algorithm accounts for the number of rentals booked, the pick-up rate and what % of rentals have been booked. Smart Rates then provides the attribution for the pricing factors and their specific weighting behind the recommended rate (see below).

While host platforms provide pricing recommendations for STRs, AirDNA’s Smart Rates gives its customers the choice to optimize for max occupancy or highest pricing. Smart Rates also allows for hosts to hand pick a competitive set that they can monitor within the platform.
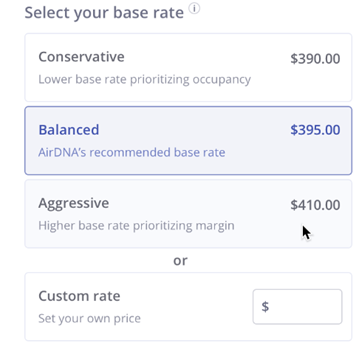
The model for recommended price demonstrates AirDNA’s accuracy in terms of recommended rate vs. reserved price.

In order to feed its internally developed algorithms, AirDNA tracks the daily performance of over 10 million properties on AirBnB and VRBO in 120,000 global markets. AirDNA also partners with over one million partner properties. This combination of scraped data and partner data has allowed AirDNA to build one of the world’s largest, most comprehensive vacation rental data and analytics platforms in the world.

Current Opportunities & Recommendations:
- With rising interest rates and inflation, AirDNA should focus on marketing its STR data and insights to homeowners looking to rent out their homes as a secondary source of income.
- AirDNA could also market its reservation algorithm / demand generation tool to hotel operators in markets with heavy STR activity. AirDNA’s demand generation tool could be integrated into the larger demand model that hotels use to price their rooms. As an alternative, AirDNA could expand its own reservation algorithm to account for hotel bookings via website scraping and charge STR hosts a premium for this enhanced demand forecast.
Current Challenges & Recommendations:
- AirDNA’s pricing algorithm’s largest challenge involves forecasting local demand in markets where they have limited partner data. If the pricing algorithm solely relies on scraped data, the algorithm isn’t as likely to generate differentiated pricing insights when compared to AirBnB’s own pricing algorithm (which is currently free for hosts). Furthermore, small local markets are likely to garner less frequent bookings, leading to a sparse data set and inaccurate pricing recommendations. AirDNA should focus on having partner data to complement its scraped data in every region that it operates in.
- Data integrity is also a significant challenge. Currently, AirDNA can legally scrape data from the websites of Airbnb and VRBO on an ongoing basis; however, there is a risk that this could change with future regulation. This sole reliance on partner data could be detrimental for the company’s algorithms and predictive insights. AirDNA should hire appropriate lobbyists and align with other organizations that rely on scraping for their business model.
Thank you for an interesting blog! I’m wondering how are the competitive forces from other players in the space like a real estate analytics platform that helps real estate investors and property owners set the right rental rates of their properties by analyzing the local market data and how AirDNA differentiates itself from these platforms? Other aspects that I’m curious is about is the efficiency of their tools & the accuracy of their input data.
Very interesting insight, thank you so much for sharing! I would love to learn their retention strategy as I imagine customers (investors, rental entrepreneurs) who are looking for investment opportunities, might leave the platform once they make their investment decisions. In this light, I also wonder if they could expand into a consumer space (but it will compete with travel aggregator websites like booking.com I assume?) leveraging its data further.
Thank you so much for this blogpost! I was not aware of AirDNA and it seems to be a very interesting business. Especially how the Matching Algorithm is able to figure out double listings on different websites. I am wondering if it would be possible to expand the search easily over more websites, or if AirDNA is limited here. Another point I would worry about is how closely the company is connected to access to data. Regulations in many countries might get in the way of their analysis. Furthermore, they seem to be really focused on analyzing some companies closely, like AirBNB, so their success is bound to the success of other companies?
Thanks for the post, Kaitlyn! One question I had as I was reading through was how AirDNA can collect more information about small local markets given that the frequency of bookings is lower but this data is critical for forecasting local demand. One potential option that I thought they could consider was whether or not there was a way to aggregate data from multiple small local markets without compromising the integrity and accuracy of the data and negatively impacting the conclusions being drawn from the available data set.
Very interesting post, Kaitlyn! This AI-powered tool can clearly empower homeowners to optimize utilization and revenues when renting their properties on Airbnb. I think one way they can pitch their value-add relative to pricing suggested by Airbnb is that Airdna is truly independent and charges a flat subscription fee. Arguable, Airbnb is incentivised to recommend below-market rates as they have a strong incentive to get properties booked (and only marginally incentivised to optimize for the highest rate). Airdna should position itself as a truly independent tool that improves decision making for short term renters.
This was a very interesting read, Kaitlyn! I had no idea that AirDNA existed. I’m also curious for the long term what their customer retention strategy is.